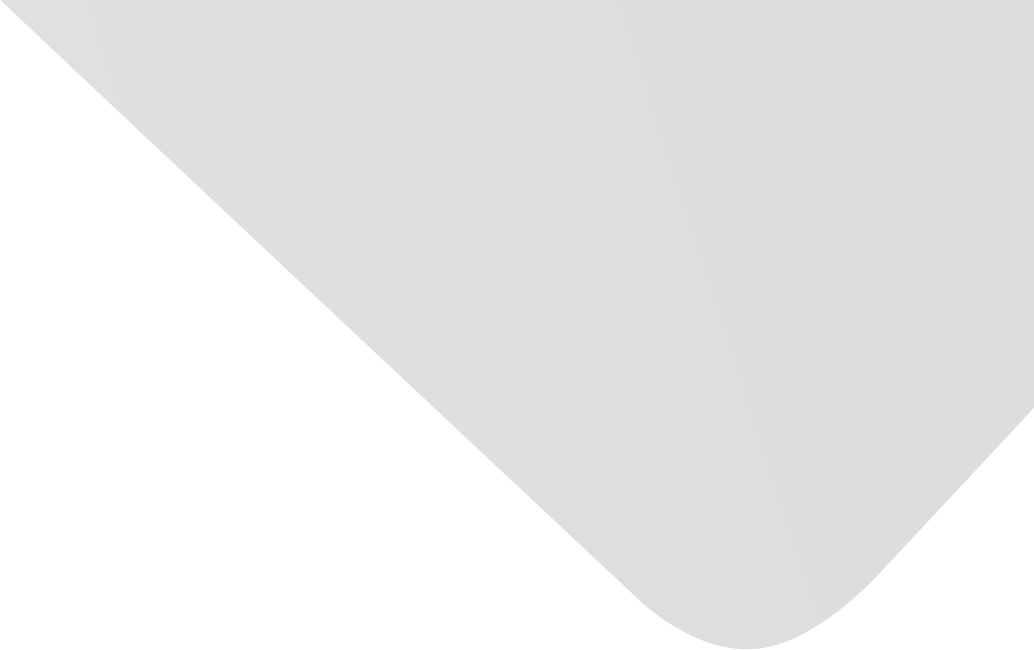
Fault Diagnosis for Rotating Machinery Based on Convolutional Neural Network and Empirical Mode Decomposition
Joint Authors
Source
Issue
Vol. 2017, Issue 2017 (31 Dec. 2017), pp.1-12, 12 p.
Publisher
Hindawi Publishing Corporation
Publication Date
2017-08-20
Country of Publication
Egypt
No. of Pages
12
Main Subjects
Abstract EN
The analysis of vibration signals has been a very important technique for fault diagnosis and health management of rotating machinery.
Classic fault diagnosis methods are mainly based on traditional signal features such as mean value, standard derivation, and kurtosis.
Signals still contain abundant information which we did not fully take advantage of.
In this paper, a new approach is proposed for rotating machinery fault diagnosis with feature extraction algorithm based on empirical mode decomposition (EMD) and convolutional neural network (CNN) techniques.
The fundamental purpose of our newly proposed approach is to extract distinguishing features.
Frequency spectrum of the signal obtained through fast Fourier transform process is trained in a designed CNN structure to extract compressed features with spatial information.
To solve the nonstationary characteristic, we also apply EMD technique to the original vibration signals.
EMD energy entropy is calculated using the first few intrinsic mode functions (IMFs) which contain more energy.
With features extracted from both methods combined, classification models are trained for diagnosis.
We carried out experiments with vibration data of 52 different categories under different machine conditions to test the validity of the approach, and the results indicate it is more accurate and reliable than previous approaches.
American Psychological Association (APA)
Xie, Yuan& Zhang, Tao. 2017. Fault Diagnosis for Rotating Machinery Based on Convolutional Neural Network and Empirical Mode Decomposition. Shock and Vibration،Vol. 2017, no. 2017, pp.1-12.
https://search.emarefa.net/detail/BIM-1204236
Modern Language Association (MLA)
Xie, Yuan& Zhang, Tao. Fault Diagnosis for Rotating Machinery Based on Convolutional Neural Network and Empirical Mode Decomposition. Shock and Vibration No. 2017 (2017), pp.1-12.
https://search.emarefa.net/detail/BIM-1204236
American Medical Association (AMA)
Xie, Yuan& Zhang, Tao. Fault Diagnosis for Rotating Machinery Based on Convolutional Neural Network and Empirical Mode Decomposition. Shock and Vibration. 2017. Vol. 2017, no. 2017, pp.1-12.
https://search.emarefa.net/detail/BIM-1204236
Data Type
Journal Articles
Language
English
Notes
Includes bibliographical references
Record ID
BIM-1204236