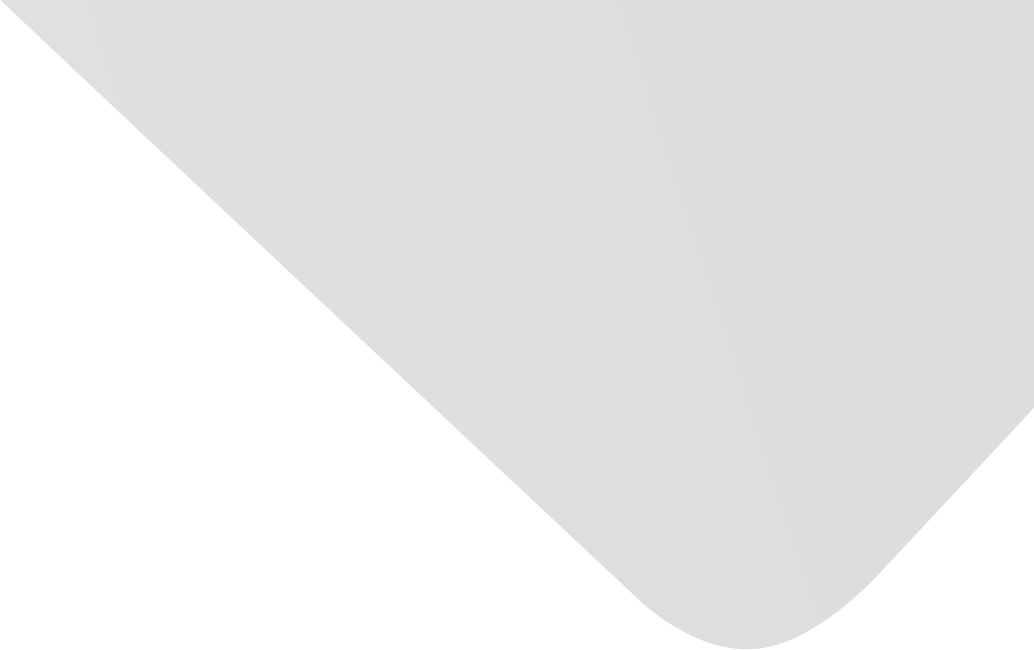
Ensemble Network Architecture for Deep Reinforcement Learning
Joint Authors
Chen, Xi-liang
Cao, Lei
Lai, Jun
Li, Chen-xi
Xu, Zhi-xiong
Source
Mathematical Problems in Engineering
Issue
Vol. 2018, Issue 2018 (31 Dec. 2018), pp.1-6, 6 p.
Publisher
Hindawi Publishing Corporation
Publication Date
2018-04-05
Country of Publication
Egypt
No. of Pages
6
Main Subjects
Abstract EN
The popular deep Q learning algorithm is known to be instability because of the Q-value’s shake and overestimation action values under certain conditions.
These issues tend to adversely affect their performance.
In this paper, we develop the ensemble network architecture for deep reinforcement learning which is based on value function approximation.
The temporal ensemble stabilizes the training process by reducing the variance of target approximation error and the ensemble of target values reduces the overestimate and makes better performance by estimating more accurate Q-value.
Our results show that this architecture leads to statistically significant better value evaluation and more stable and better performance on several classical control tasks at OpenAI Gym environment.
American Psychological Association (APA)
Chen, Xi-liang& Cao, Lei& Li, Chen-xi& Xu, Zhi-xiong& Lai, Jun. 2018. Ensemble Network Architecture for Deep Reinforcement Learning. Mathematical Problems in Engineering،Vol. 2018, no. 2018, pp.1-6.
https://search.emarefa.net/detail/BIM-1206061
Modern Language Association (MLA)
Chen, Xi-liang…[et al.]. Ensemble Network Architecture for Deep Reinforcement Learning. Mathematical Problems in Engineering No. 2018 (2018), pp.1-6.
https://search.emarefa.net/detail/BIM-1206061
American Medical Association (AMA)
Chen, Xi-liang& Cao, Lei& Li, Chen-xi& Xu, Zhi-xiong& Lai, Jun. Ensemble Network Architecture for Deep Reinforcement Learning. Mathematical Problems in Engineering. 2018. Vol. 2018, no. 2018, pp.1-6.
https://search.emarefa.net/detail/BIM-1206061
Data Type
Journal Articles
Language
English
Notes
Includes bibliographical references
Record ID
BIM-1206061