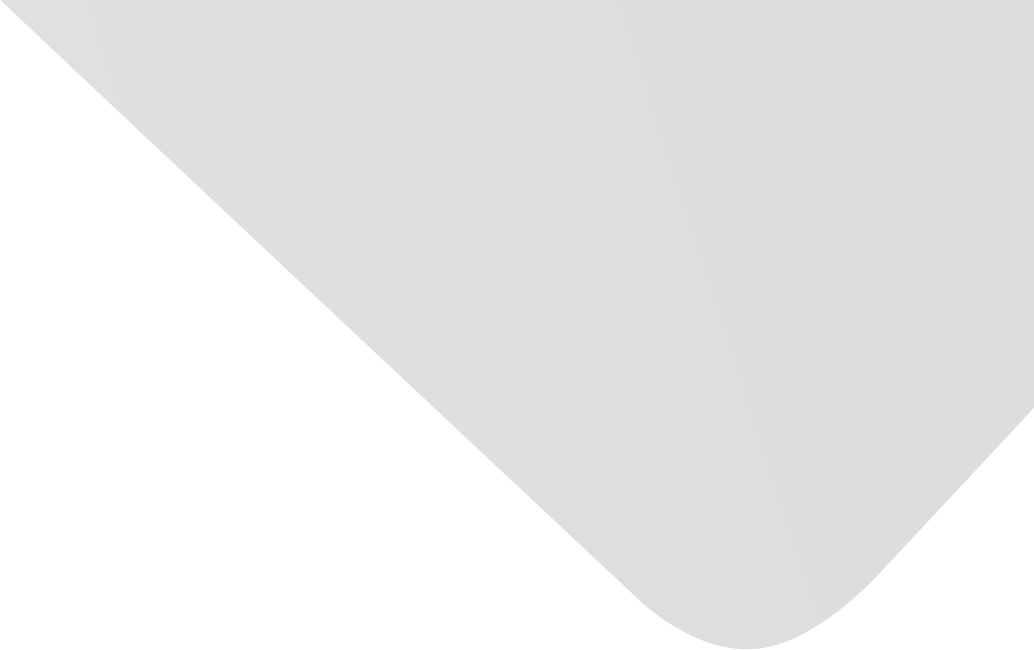
Combining LSTM Network Ensemble via Adaptive Weighting for Improved Time Series Forecasting
Joint Authors
Source
Mathematical Problems in Engineering
Issue
Vol. 2018, Issue 2018 (31 Dec. 2018), pp.1-8, 8 p.
Publisher
Hindawi Publishing Corporation
Publication Date
2018-08-05
Country of Publication
Egypt
No. of Pages
8
Main Subjects
Abstract EN
Time series forecasting is essential for various engineering applications in finance, geology, and information technology, etc.
Long Short-Term Memory (LSTM) networks are nowadays gaining renewed interest and they are replacing many practical implementations of the time series forecasting systems.
This paper presents a novel LSTM ensemble forecasting algorithm that effectively combines multiple forecast (prediction) results from a set of individual LSTM networks.
The main advantages of our LSTM ensemble method over other state-of-the-art ensemble techniques are summarized as follows: (1) we develop a novel way of dynamically adjusting the combining weights that are used for combining multiple LSTM models to produce the composite prediction output; for this, our method is devised for updating combining weights at each time step in an adaptive and recursive way by using both past prediction errors and forgetting weight factor; (2) our method is capable of well capturing nonlinear statistical properties in the time series, which considerably improves the forecasting accuracy; (3) our method is straightforward to implement and computationally efficient when it comes to runtime performance because it does not require the complex optimization in the process of finding combining weights.
Comparative experiments demonstrate that our proposed LSTM ensemble method achieves state-of-the-art forecasting performance on four real-life time series datasets publicly available.
American Psychological Association (APA)
Choi, Jae Young& Lee, Bumshik. 2018. Combining LSTM Network Ensemble via Adaptive Weighting for Improved Time Series Forecasting. Mathematical Problems in Engineering،Vol. 2018, no. 2018, pp.1-8.
https://search.emarefa.net/detail/BIM-1206247
Modern Language Association (MLA)
Choi, Jae Young& Lee, Bumshik. Combining LSTM Network Ensemble via Adaptive Weighting for Improved Time Series Forecasting. Mathematical Problems in Engineering No. 2018 (2018), pp.1-8.
https://search.emarefa.net/detail/BIM-1206247
American Medical Association (AMA)
Choi, Jae Young& Lee, Bumshik. Combining LSTM Network Ensemble via Adaptive Weighting for Improved Time Series Forecasting. Mathematical Problems in Engineering. 2018. Vol. 2018, no. 2018, pp.1-8.
https://search.emarefa.net/detail/BIM-1206247
Data Type
Journal Articles
Language
English
Notes
Includes bibliographical references
Record ID
BIM-1206247