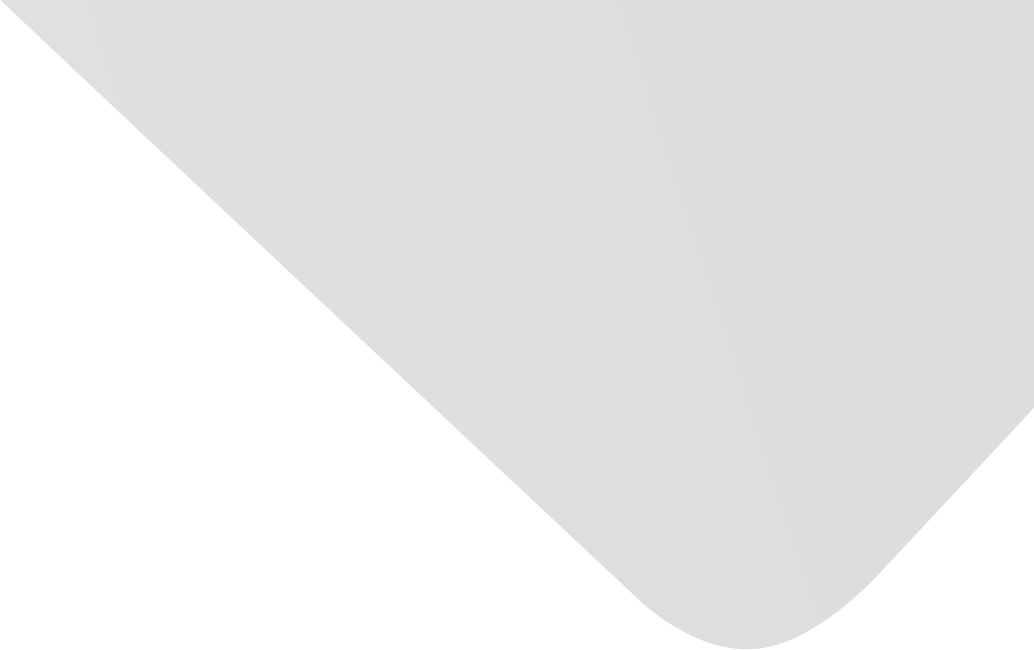
Multimodal Feature Learning for Video Captioning
Joint Authors
Source
Mathematical Problems in Engineering
Issue
Vol. 2018, Issue 2018 (31 Dec. 2018), pp.1-8, 8 p.
Publisher
Hindawi Publishing Corporation
Publication Date
2018-02-19
Country of Publication
Egypt
No. of Pages
8
Main Subjects
Abstract EN
Video captioning refers to the task of generating a natural language sentence that explains the content of the input video clips.
This study proposes a deep neural network model for effective video captioning.
Apart from visual features, the proposed model learns additionally semantic features that describe the video content effectively.
In our model, visual features of the input video are extracted using convolutional neural networks such as C3D and ResNet, while semantic features are obtained using recurrent neural networks such as LSTM.
In addition, our model includes an attention-based caption generation network to generate the correct natural language captions based on the multimodal video feature sequences.
Various experiments, conducted with the two large benchmark datasets, Microsoft Video Description (MSVD) and Microsoft Research Video-to-Text (MSR-VTT), demonstrate the performance of the proposed model.
American Psychological Association (APA)
Lee, Sujin& Kim, Incheol. 2018. Multimodal Feature Learning for Video Captioning. Mathematical Problems in Engineering،Vol. 2018, no. 2018, pp.1-8.
https://search.emarefa.net/detail/BIM-1206682
Modern Language Association (MLA)
Lee, Sujin& Kim, Incheol. Multimodal Feature Learning for Video Captioning. Mathematical Problems in Engineering No. 2018 (2018), pp.1-8.
https://search.emarefa.net/detail/BIM-1206682
American Medical Association (AMA)
Lee, Sujin& Kim, Incheol. Multimodal Feature Learning for Video Captioning. Mathematical Problems in Engineering. 2018. Vol. 2018, no. 2018, pp.1-8.
https://search.emarefa.net/detail/BIM-1206682
Data Type
Journal Articles
Language
English
Notes
Includes bibliographical references
Record ID
BIM-1206682