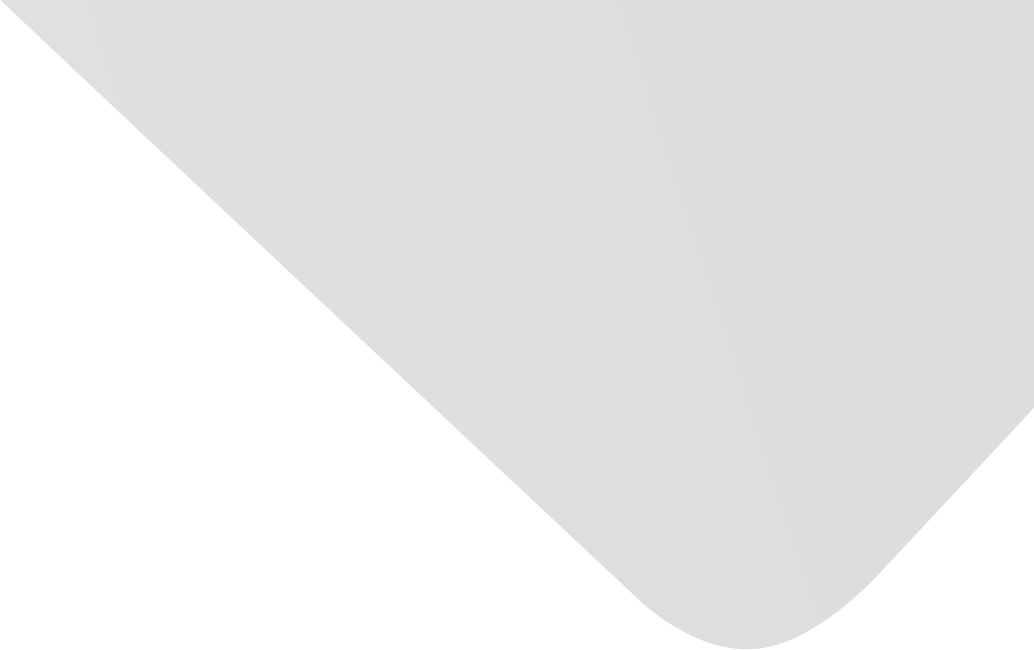
Radiomics and Machine Learning Differentiate Soft-Tissue Lipoma and Liposarcoma Better than Musculoskeletal Radiologists
Joint Authors
Montet, Xavier
Hamard, M.
Boudabbous, S.
Malinauskaite, Ieva
Hofmeister, Jeremy
Burgermeister, Simon
Neroladaki, Angeliki
Source
Issue
Vol. 2020, Issue 2020 (31 Dec. 2020), pp.1-9, 9 p.
Publisher
Hindawi Publishing Corporation
Publication Date
2020-01-07
Country of Publication
Egypt
No. of Pages
9
Main Subjects
Abstract EN
Distinguishing lipoma from liposarcoma is challenging on conventional MRI examination.
In case of uncertain diagnosis following MRI, further invasive procedure (percutaneous biopsy or surgery) is often required to allow for diagnosis based on histopathological examination.
Radiomics and machine learning allow for several types of pathologies encountered on radiological images to be automatically and reliably distinguished.
The aim of the study was to assess the contribution of radiomics and machine learning in the differentiation between soft-tissue lipoma and liposarcoma on preoperative MRI and to assess the diagnostic accuracy of a machine-learning model compared to musculoskeletal radiologists.
86 radiomics features were retrospectively extracted from volume-of-interest on T1-weighted spin-echo 1.5 and 3.0 Tesla MRI of 38 soft-tissue tumors (24 lipomas and 14 liposarcomas, based on histopathological diagnosis).
These radiomics features were then used to train a machine-learning classifier to distinguish lipoma and liposarcoma.
The generalization performance of the machine-learning model was assessed using Monte-Carlo cross-validation and receiver operating characteristic curve analysis (ROC-AUC).
Finally, the performance of the machine-learning model was compared to the accuracy of three specialized musculoskeletal radiologists using the McNemar test.
Machine-learning classifier accurately distinguished lipoma and liposarcoma, with a ROC-AUC of 0.926.
Notably, it performed better than the three specialized musculoskeletal radiologists reviewing the same patients, who achieved ROC-AUC of 0.685, 0.805, and 0.785.
Despite being developed on few cases, the trained machine-learning classifier accurately distinguishes lipoma and liposarcoma on preoperative MRI, with better performance than specialized musculoskeletal radiologists.
American Psychological Association (APA)
Malinauskaite, Ieva& Hofmeister, Jeremy& Burgermeister, Simon& Neroladaki, Angeliki& Hamard, M.& Montet, Xavier…[et al.]. 2020. Radiomics and Machine Learning Differentiate Soft-Tissue Lipoma and Liposarcoma Better than Musculoskeletal Radiologists. Complexity،Vol. 2020, no. 2020, pp.1-9.
https://search.emarefa.net/detail/BIM-1207345
Modern Language Association (MLA)
Malinauskaite, Ieva…[et al.]. Radiomics and Machine Learning Differentiate Soft-Tissue Lipoma and Liposarcoma Better than Musculoskeletal Radiologists. Complexity No. 2020 (2020), pp.1-9.
https://search.emarefa.net/detail/BIM-1207345
American Medical Association (AMA)
Malinauskaite, Ieva& Hofmeister, Jeremy& Burgermeister, Simon& Neroladaki, Angeliki& Hamard, M.& Montet, Xavier…[et al.]. Radiomics and Machine Learning Differentiate Soft-Tissue Lipoma and Liposarcoma Better than Musculoskeletal Radiologists. Complexity. 2020. Vol. 2020, no. 2020, pp.1-9.
https://search.emarefa.net/detail/BIM-1207345
Data Type
Journal Articles
Language
English
Notes
Includes bibliographical references
Record ID
BIM-1207345