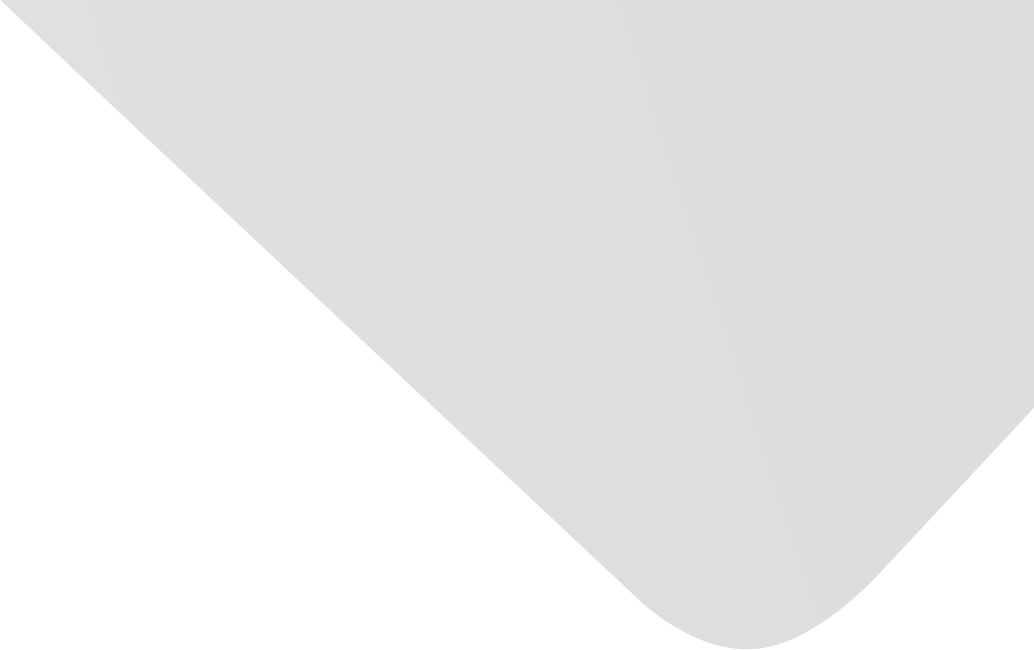
Learning Multimodal Deep Representations for Crowd Anomaly Event Detection
Joint Authors
Huang, Shao-nian
Huang, Dong-jun
Zhou, Xinmin
Source
Mathematical Problems in Engineering
Issue
Vol. 2018, Issue 2018 (31 Dec. 2018), pp.1-13, 13 p.
Publisher
Hindawi Publishing Corporation
Publication Date
2018-01-31
Country of Publication
Egypt
No. of Pages
13
Main Subjects
Abstract EN
Anomaly event detection in crowd scenes is extremely important; however, the majority of existing studies merely use hand-crafted features to detect anomalies.
In this study, a novel unsupervised deep learning framework is proposed to detect anomaly events in crowded scenes.
Specifically, low-level visual features, energy features, and motion map features are simultaneously extracted based on spatiotemporal energy measurements.
Three convolutional restricted Boltzmann machines are trained to model the mid-level feature representation of normal patterns.
Then a multimodal fusion scheme is utilized to learn the deep representation of crowd patterns.
Based on the learned deep representation, a one-class support vector machine model is used to detect anomaly events.
The proposed method is evaluated using two available public datasets and compared with state-of-the-art methods.
The experimental results show its competitive performance for anomaly event detection in video surveillance.
American Psychological Association (APA)
Huang, Shao-nian& Huang, Dong-jun& Zhou, Xinmin. 2018. Learning Multimodal Deep Representations for Crowd Anomaly Event Detection. Mathematical Problems in Engineering،Vol. 2018, no. 2018, pp.1-13.
https://search.emarefa.net/detail/BIM-1208337
Modern Language Association (MLA)
Huang, Shao-nian…[et al.]. Learning Multimodal Deep Representations for Crowd Anomaly Event Detection. Mathematical Problems in Engineering No. 2018 (2018), pp.1-13.
https://search.emarefa.net/detail/BIM-1208337
American Medical Association (AMA)
Huang, Shao-nian& Huang, Dong-jun& Zhou, Xinmin. Learning Multimodal Deep Representations for Crowd Anomaly Event Detection. Mathematical Problems in Engineering. 2018. Vol. 2018, no. 2018, pp.1-13.
https://search.emarefa.net/detail/BIM-1208337
Data Type
Journal Articles
Language
English
Notes
Includes bibliographical references
Record ID
BIM-1208337