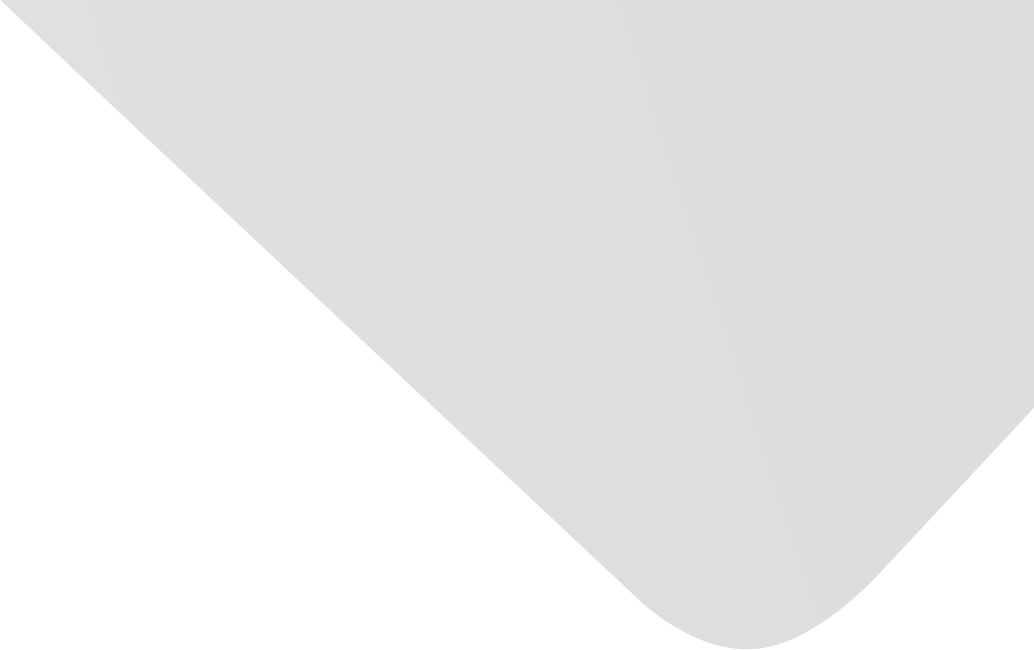
Cycle-Consistent Adversarial GAN: The Integration of Adversarial Attack and Defense
Joint Authors
Bu, Haibing
Jiang, Lingyun
Chen, Jian
Qin, RuoXi
Qiao, Kai
Yu, Wanting
Wang, Linyuan
Yan, Bin
Source
Security and Communication Networks
Issue
Vol. 2020, Issue 2020 (31 Dec. 2020), pp.1-9, 9 p.
Publisher
Hindawi Publishing Corporation
Publication Date
2020-02-21
Country of Publication
Egypt
No. of Pages
9
Main Subjects
Information Technology and Computer Science
Abstract EN
In image classification of deep learning, adversarial examples where input is intended to add small magnitude perturbations may mislead deep neural networks (DNNs) to incorrect results, which means DNNs are vulnerable to them.
Different attack and defense strategies have been proposed to better research the mechanism of deep learning.
However, those researches in these networks are only for one aspect, either an attack or a defense.
There is in the improvement of offensive and defensive performance, and it is difficult to promote each other in the same framework.
In this paper, we propose Cycle-Consistent Adversarial GAN (CycleAdvGAN) to generate adversarial examples, which can learn and approximate the distribution of the original instances and adversarial examples, especially promoting attackers and defenders to confront each other and improve their ability.
For CycleAdvGAN, once the GeneratorA and D are trained, GA can generate adversarial perturbations efficiently for any instance, improving the performance of the existing attack methods, and GD can generate recovery adversarial examples to clean instances, defending against existing attack methods.
We apply CycleAdvGAN under semiwhite-box and black-box settings on two public datasets MNIST and CIFAR10.
Using the extensive experiments, we show that our method has achieved the state-of-the-art adversarial attack method and also has efficiently improved the defense ability, which made the integration of adversarial attack and defense come true.
In addition, it has improved the attack effect only trained on the adversarial dataset generated by any kind of adversarial attack.
American Psychological Association (APA)
Jiang, Lingyun& Qiao, Kai& Qin, RuoXi& Wang, Linyuan& Yu, Wanting& Chen, Jian…[et al.]. 2020. Cycle-Consistent Adversarial GAN: The Integration of Adversarial Attack and Defense. Security and Communication Networks،Vol. 2020, no. 2020, pp.1-9.
https://search.emarefa.net/detail/BIM-1208395
Modern Language Association (MLA)
Jiang, Lingyun…[et al.]. Cycle-Consistent Adversarial GAN: The Integration of Adversarial Attack and Defense. Security and Communication Networks No. 2020 (2020), pp.1-9.
https://search.emarefa.net/detail/BIM-1208395
American Medical Association (AMA)
Jiang, Lingyun& Qiao, Kai& Qin, RuoXi& Wang, Linyuan& Yu, Wanting& Chen, Jian…[et al.]. Cycle-Consistent Adversarial GAN: The Integration of Adversarial Attack and Defense. Security and Communication Networks. 2020. Vol. 2020, no. 2020, pp.1-9.
https://search.emarefa.net/detail/BIM-1208395
Data Type
Journal Articles
Language
English
Notes
Includes bibliographical references
Record ID
BIM-1208395