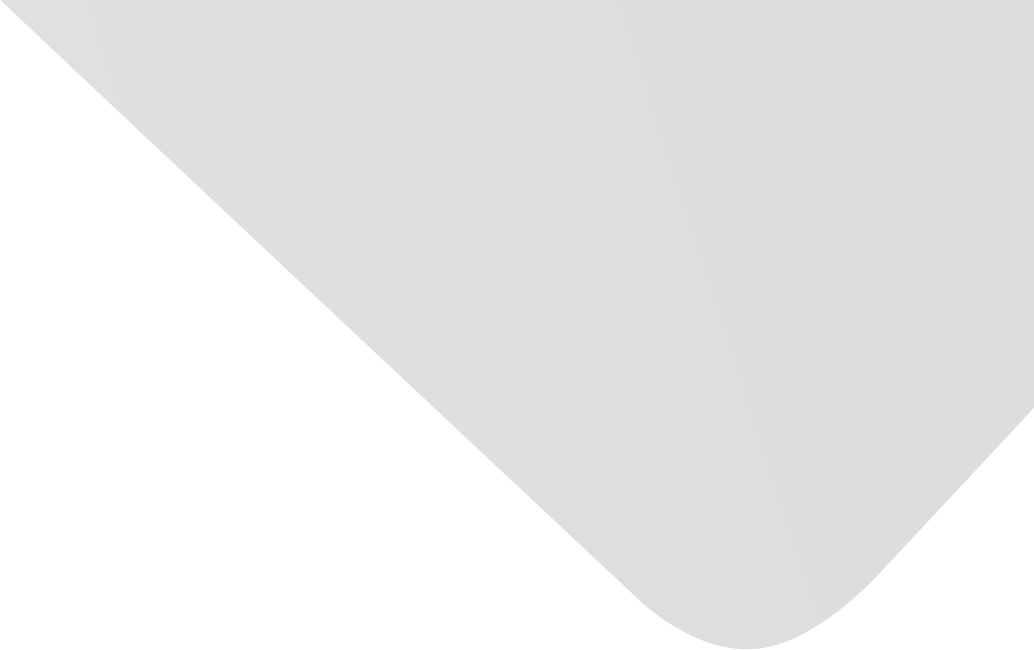
High-Efficiency Min-Entropy Estimation Based on Neural Network for Random Number Generators
Joint Authors
Lin, Jingqiang
Lv, Na
Chen, Tianyu
Zhu, Shuangyi
Yang, Jing
Ma, Yuan
Jing, Jiwu
Source
Security and Communication Networks
Issue
Vol. 2020, Issue 2020 (31 Dec. 2020), pp.1-18, 18 p.
Publisher
Hindawi Publishing Corporation
Publication Date
2020-02-17
Country of Publication
Egypt
No. of Pages
18
Main Subjects
Information Technology and Computer Science
Abstract EN
Random number generator (RNG) is a fundamental and important cryptographic element, which has made an outstanding contribution to guaranteeing the network and communication security of cryptographic applications in the Internet age.
In reality, if the random number used cannot provide sufficient randomness (unpredictability) as expected, these cryptographic applications are vulnerable to security threats and cause system crashes.
Min-entropy is one of the approaches that are usually employed to quantify the unpredictability.
The NIST Special Publication 800-90B adopts the concept of min-entropy in the design of its statistical entropy estimation methods, and the predictive model-based estimators added in the second draft of this standard effectively improve the overall capability of the test suite.
However, these predictors have problems on limited application scope and high computational complexity, e.g., they have shortfalls in evaluating random numbers with long dependence and multivariate due to the huge time complexity (i.e., high-order polynomial time complexity).
Fortunately, there has been increasing attention to using neural networks to model and forecast time series, and random numbers are also a type of time series.
In our work, we propose several new and efficient approaches for min-entropy estimation by using neural network technologies and design a novel execution strategy for the proposed entropy estimation to make it applicable to the validation of both stationary and nonstationary sources.
Compared with the 90B’s predictors officially published in 2018, the experimental results on various simulated and real-world data sources demonstrate that our predictors have a better performance on the accuracy, scope of applicability, and execution efficiency.
The average execution efficiency of our predictors can be up to 10 times higher than that of the 90B’s for 106 sample size with different sample spaces.
Furthermore, when the sample space is over 22 and the sample size is over 108, the 90B’s predictors cannot give estimated results.
Instead, our predictors can still provide accurate results.
Copyright© 2019 John Wiley & Sons, Ltd.
American Psychological Association (APA)
Lv, Na& Chen, Tianyu& Zhu, Shuangyi& Yang, Jing& Ma, Yuan& Jing, Jiwu…[et al.]. 2020. High-Efficiency Min-Entropy Estimation Based on Neural Network for Random Number Generators. Security and Communication Networks،Vol. 2020, no. 2020, pp.1-18.
https://search.emarefa.net/detail/BIM-1208420
Modern Language Association (MLA)
Lv, Na…[et al.]. High-Efficiency Min-Entropy Estimation Based on Neural Network for Random Number Generators. Security and Communication Networks No. 2020 (2020), pp.1-18.
https://search.emarefa.net/detail/BIM-1208420
American Medical Association (AMA)
Lv, Na& Chen, Tianyu& Zhu, Shuangyi& Yang, Jing& Ma, Yuan& Jing, Jiwu…[et al.]. High-Efficiency Min-Entropy Estimation Based on Neural Network for Random Number Generators. Security and Communication Networks. 2020. Vol. 2020, no. 2020, pp.1-18.
https://search.emarefa.net/detail/BIM-1208420
Data Type
Journal Articles
Language
English
Notes
Includes bibliographical references
Record ID
BIM-1208420