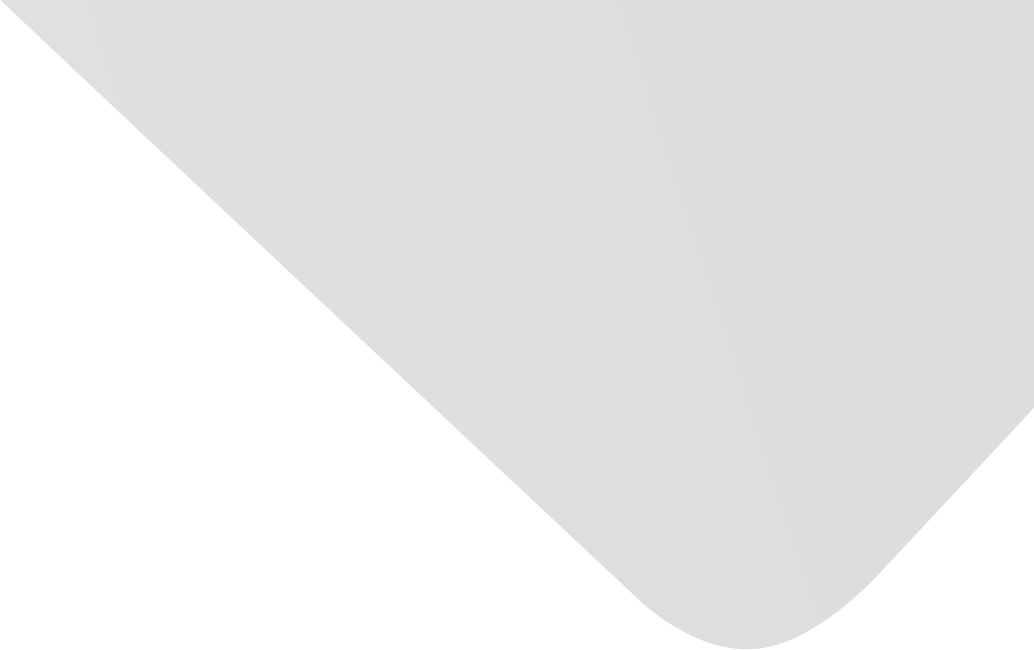
Exploiting the Relationship between Pruning Ratio and Compression Effect for Neural Network Model Based on TensorFlow
Joint Authors
Zhang, Yiwen
Liu, Bo
Wu, Qilin
Cao, Qian
Source
Security and Communication Networks
Issue
Vol. 2020, Issue 2020 (31 Dec. 2020), pp.1-8, 8 p.
Publisher
Hindawi Publishing Corporation
Publication Date
2020-04-30
Country of Publication
Egypt
No. of Pages
8
Main Subjects
Information Technology and Computer Science
Abstract EN
Pruning is a method of compressing the size of a neural network model, which affects the accuracy and computing time when the model makes a prediction.
In this paper, the hypothesis that the pruning proportion is positively correlated with the compression scale of the model but not with the prediction accuracy and calculation time is put forward.
For testing the hypothesis, a group of experiments are designed, and MNIST is used as the data set to train a neural network model based on TensorFlow.
Based on this model, pruning experiments are carried out to investigate the relationship between pruning proportion and compression effect.
For comparison, six different pruning proportions are set, and the experimental results confirm the above hypothesis.
American Psychological Association (APA)
Liu, Bo& Wu, Qilin& Zhang, Yiwen& Cao, Qian. 2020. Exploiting the Relationship between Pruning Ratio and Compression Effect for Neural Network Model Based on TensorFlow. Security and Communication Networks،Vol. 2020, no. 2020, pp.1-8.
https://search.emarefa.net/detail/BIM-1208441
Modern Language Association (MLA)
Liu, Bo…[et al.]. Exploiting the Relationship between Pruning Ratio and Compression Effect for Neural Network Model Based on TensorFlow. Security and Communication Networks No. 2020 (2020), pp.1-8.
https://search.emarefa.net/detail/BIM-1208441
American Medical Association (AMA)
Liu, Bo& Wu, Qilin& Zhang, Yiwen& Cao, Qian. Exploiting the Relationship between Pruning Ratio and Compression Effect for Neural Network Model Based on TensorFlow. Security and Communication Networks. 2020. Vol. 2020, no. 2020, pp.1-8.
https://search.emarefa.net/detail/BIM-1208441
Data Type
Journal Articles
Language
English
Notes
Includes bibliographical references
Record ID
BIM-1208441