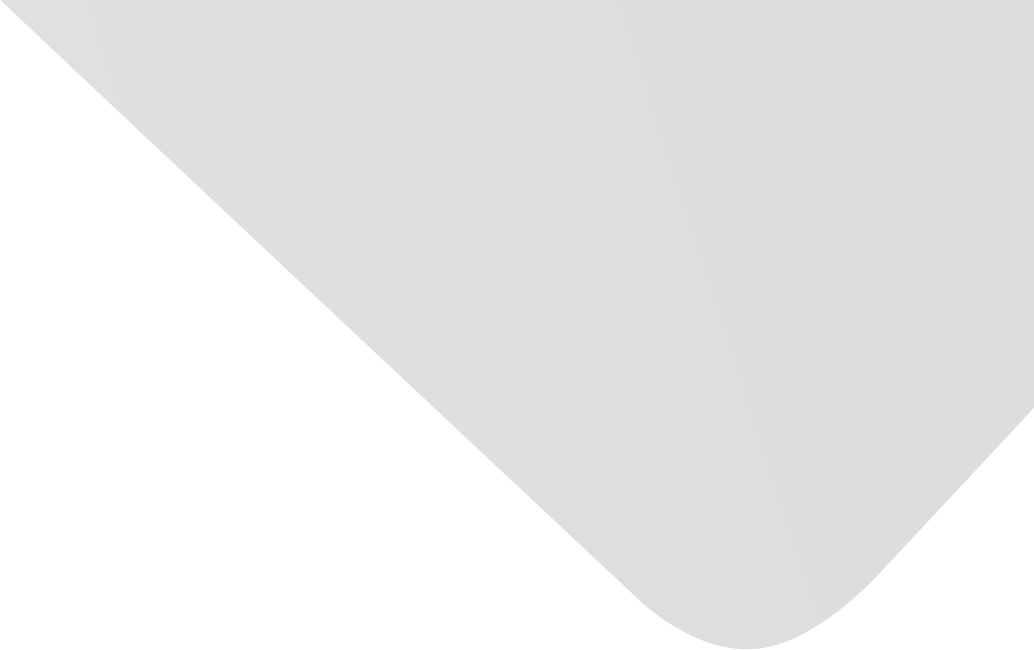
DPAEG: A Dependency Parse-Based Adversarial Examples Generation Method for Intelligent Q&A Robots
Joint Authors
Wang, Jian
Xue, Mingfu
Yuan, Chengxiang
Liu, Weiqiang
Source
Security and Communication Networks
Issue
Vol. 2020, Issue 2020 (31 Dec. 2020), pp.1-15, 15 p.
Publisher
Hindawi Publishing Corporation
Publication Date
2020-01-13
Country of Publication
Egypt
No. of Pages
15
Main Subjects
Information Technology and Computer Science
Abstract EN
Recently, the natural language processing- (NLP-) based intelligent question and answer (Q&A) robots have been used ubiquitously.
However, the robustness and security of current Q&A robots are still unsatisfactory, e.g., a slight typo in the user’s question may cause the Q&A robot unable to return the correct answer.
In this paper, we propose a fast and automatic test dataset generation method for the robustness and security evaluation of current Q&A robots, which can work in black-box scenarios and thus can be applied to a variety of different Q&A robots.
Specifically, we propose a dependency parse-based adversarial examples generation (DPAEG) method for Q&A robots.
DPAEG first uses the proposed dependency parse-based keywords extraction algorithm to extract keywords from a question.
Then, the proposed algorithm generates adversarial words according to the extracted keywords, which include typos and words that are spelled similarly to the keywords.
Finally, these adversarial words are used to generate a large number of adversarial questions.
The generated adversarial questions which are similar to the original questions do not affect human’s understanding, but the Q&A robots cannot answer these adversarial questions correctly.
Moreover, the proposed method works in a black-box scenario, which means it does not need the knowledge of the target Q&A robots.
Experiment results show that the generated adversarial examples have a high success rate on two state-of-the-art Q&A robots, DrQA and Google Assistant.
In addition, the generated adversarial examples not only affect the correct answer (top-1) returned by DrQA but also affect the top-k candidate answers returned by DrQA.
The adversarial examples make the top-k candidate answers contain fewer correct answers and make the correct answers rank lower in the top-k candidate answers.
The human evaluation results show that participants with different genders, ages, and mother tongues can understand the meaning of most of the generated adversarial examples, which means that the generated adversarial examples do not affect human’s understanding.
American Psychological Association (APA)
Xue, Mingfu& Yuan, Chengxiang& Wang, Jian& Liu, Weiqiang. 2020. DPAEG: A Dependency Parse-Based Adversarial Examples Generation Method for Intelligent Q&A Robots. Security and Communication Networks،Vol. 2020, no. 2020, pp.1-15.
https://search.emarefa.net/detail/BIM-1208462
Modern Language Association (MLA)
Xue, Mingfu…[et al.]. DPAEG: A Dependency Parse-Based Adversarial Examples Generation Method for Intelligent Q&A Robots. Security and Communication Networks No. 2020 (2020), pp.1-15.
https://search.emarefa.net/detail/BIM-1208462
American Medical Association (AMA)
Xue, Mingfu& Yuan, Chengxiang& Wang, Jian& Liu, Weiqiang. DPAEG: A Dependency Parse-Based Adversarial Examples Generation Method for Intelligent Q&A Robots. Security and Communication Networks. 2020. Vol. 2020, no. 2020, pp.1-15.
https://search.emarefa.net/detail/BIM-1208462
Data Type
Journal Articles
Language
English
Notes
Includes bibliographical references
Record ID
BIM-1208462