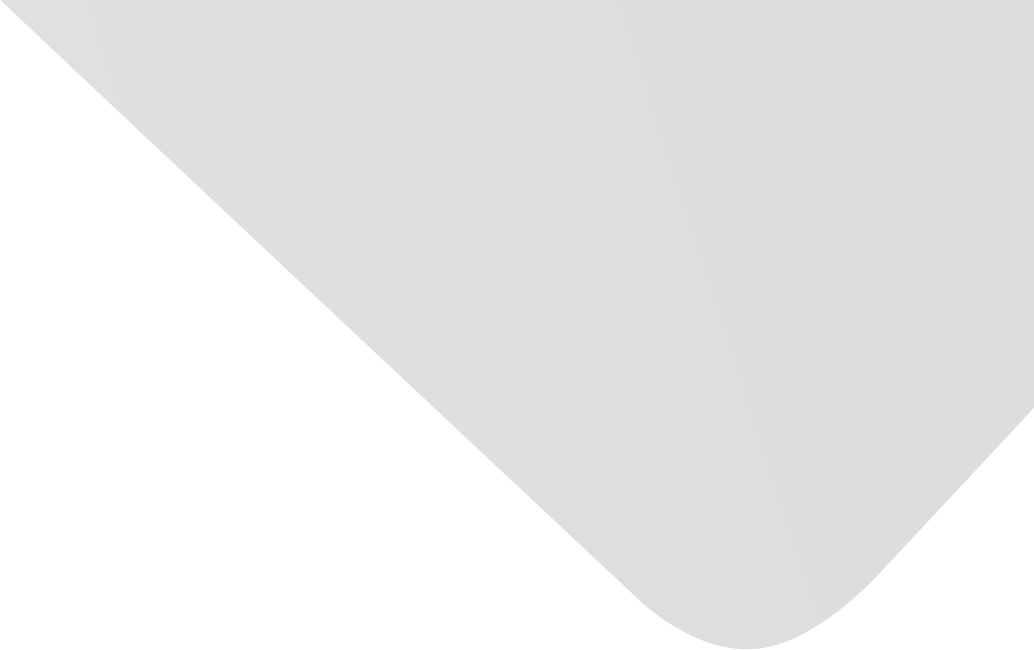
GFD: A Weighted Heterogeneous Graph Embedding Based Approach for Fraud Detection in Mobile Advertising
Joint Authors
Dong, Shoubin
Li, Tenghui
Huang, Song
Hu, Jinlong
Zhuang, Yi
Source
Security and Communication Networks
Issue
Vol. 2020, Issue 2020 (31 Dec. 2020), pp.1-12, 12 p.
Publisher
Hindawi Publishing Corporation
Publication Date
2020-09-04
Country of Publication
Egypt
No. of Pages
12
Main Subjects
Information Technology and Computer Science
Abstract EN
Online mobile advertising plays a vital role in the mobile app ecosystem.
The mobile advertising frauds caused by fraudulent clicks or other actions on advertisements are considered one of the most critical issues in mobile advertising systems.
To combat the evolving mobile advertising frauds, machine learning methods have been successfully applied to identify advertising frauds in tabular data, distinguishing suspicious advertising fraud operation from normal one.
However, such approaches may suffer from labor-intensive feature engineering and robustness of the detection algorithms, since the online advertising big data and complex fraudulent advertising actions generated by malicious codes, botnets, and click-firms are constantly changing.
In this paper, we propose a novel weighted heterogeneous graph embedding and deep learning-based fraud detection approach, namely, GFD, to identify fraudulent apps for mobile advertising.
In the proposed GFD approach, (i) we construct a weighted heterogeneous graph to represent behavior patterns between users, mobile apps, and mobile ads and design a weighted metapath to vector algorithm to learn node representations (graph-based features) from the graph; (ii) we use a time window based statistical analysis method to extract intrinsic features (attribute-based features) from the tabular sample data; (iii) we propose a hybrid neural network to fuse graph-based features and attribute-based features for classifying the fraudulent apps from normal apps.
The GFD approach was applied on a large real-world mobile advertising dataset, and experiment results demonstrate that the approach significantly outperforms well-known learning methods.
American Psychological Association (APA)
Hu, Jinlong& Li, Tenghui& Zhuang, Yi& Huang, Song& Dong, Shoubin. 2020. GFD: A Weighted Heterogeneous Graph Embedding Based Approach for Fraud Detection in Mobile Advertising. Security and Communication Networks،Vol. 2020, no. 2020, pp.1-12.
https://search.emarefa.net/detail/BIM-1208580
Modern Language Association (MLA)
Hu, Jinlong…[et al.]. GFD: A Weighted Heterogeneous Graph Embedding Based Approach for Fraud Detection in Mobile Advertising. Security and Communication Networks No. 2020 (2020), pp.1-12.
https://search.emarefa.net/detail/BIM-1208580
American Medical Association (AMA)
Hu, Jinlong& Li, Tenghui& Zhuang, Yi& Huang, Song& Dong, Shoubin. GFD: A Weighted Heterogeneous Graph Embedding Based Approach for Fraud Detection in Mobile Advertising. Security and Communication Networks. 2020. Vol. 2020, no. 2020, pp.1-12.
https://search.emarefa.net/detail/BIM-1208580
Data Type
Journal Articles
Language
English
Notes
Includes bibliographical references
Record ID
BIM-1208580