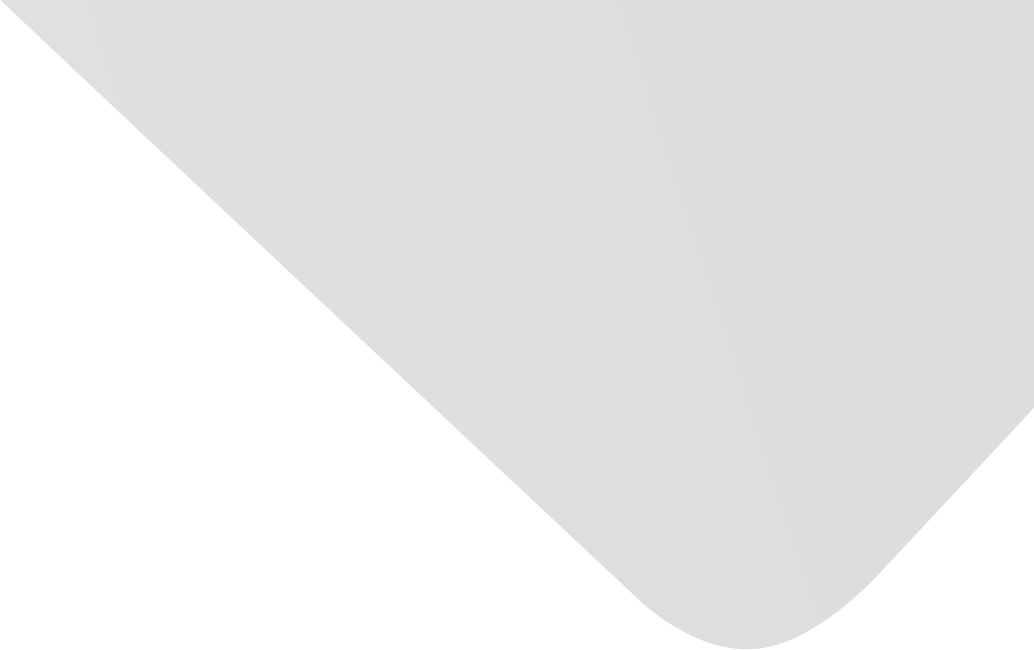
Content-Aware Compressive Sensing Recovery Using Laplacian Scale Mixture Priors and Side Information
Joint Authors
Xie, Zhonghua
Ma, Lihong
Liu, Lingjun
Source
Mathematical Problems in Engineering
Issue
Vol. 2018, Issue 2018 (31 Dec. 2018), pp.1-15, 15 p.
Publisher
Hindawi Publishing Corporation
Publication Date
2018-01-29
Country of Publication
Egypt
No. of Pages
15
Main Subjects
Abstract EN
Nonlocal methods have shown great potential in many image restoration tasks including compressive sensing (CS) reconstruction through use of image self-similarity prior.
However, they are still limited in recovering fine-scale details and sharp features, when rich repetitive patterns cannot be guaranteed; moreover the CS measurements are corrupted.
In this paper, we propose a novel CS recovery algorithm that combines nonlocal sparsity with local and global prior, which soften and complement the self-similarity assumption for irregular structures.
First, a Laplacian scale mixture (LSM) prior is utilized to model dependencies among similar patches.
For achieving group sparsity, each singular value of similar packed patches is modeled as a Laplacian distribution with a variable scale parameter.
Second, a global prior and a compensation-based sparsity prior of local patch are designed in order to maintain differences between packed patches.
The former refers to a prediction which integrates the information at the independent processing stage and is used as side information, while the latter enforces a small (i.e., sparse) prediction error and is also modeled with the LSM model so as to obtain local sparsity.
Afterward, we derive an efficient algorithm based on the expectation-maximization (EM) and approximate message passing (AMP) frame for the maximum a posteriori (MAP) estimation of the sparse coefficients.
Numerical experiments show that the proposed method outperforms many CS recovery algorithms.
American Psychological Association (APA)
Xie, Zhonghua& Ma, Lihong& Liu, Lingjun. 2018. Content-Aware Compressive Sensing Recovery Using Laplacian Scale Mixture Priors and Side Information. Mathematical Problems in Engineering،Vol. 2018, no. 2018, pp.1-15.
https://search.emarefa.net/detail/BIM-1208732
Modern Language Association (MLA)
Xie, Zhonghua…[et al.]. Content-Aware Compressive Sensing Recovery Using Laplacian Scale Mixture Priors and Side Information. Mathematical Problems in Engineering No. 2018 (2018), pp.1-15.
https://search.emarefa.net/detail/BIM-1208732
American Medical Association (AMA)
Xie, Zhonghua& Ma, Lihong& Liu, Lingjun. Content-Aware Compressive Sensing Recovery Using Laplacian Scale Mixture Priors and Side Information. Mathematical Problems in Engineering. 2018. Vol. 2018, no. 2018, pp.1-15.
https://search.emarefa.net/detail/BIM-1208732
Data Type
Journal Articles
Language
English
Notes
Includes bibliographical references
Record ID
BIM-1208732