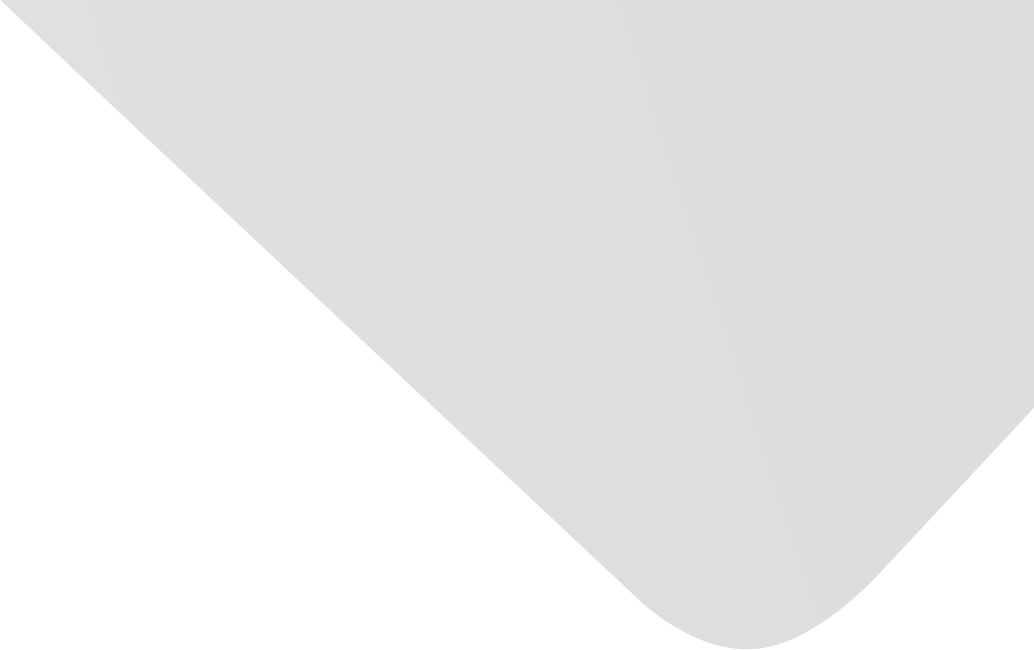
Challenging the Adversarial Robustness of DNNs Based on Error-Correcting Output Codes
Joint Authors
Zhang, Bowen
Tondi, Benedetta
Lv, Xixiang
Barni, Mauro
Source
Security and Communication Networks
Issue
Vol. 2020, Issue 2020 (31 Dec. 2020), pp.1-11, 11 p.
Publisher
Hindawi Publishing Corporation
Publication Date
2020-11-16
Country of Publication
Egypt
No. of Pages
11
Main Subjects
Information Technology and Computer Science
Abstract EN
The existence of adversarial examples and the easiness with which they can be generated raise several security concerns with regard to deep learning systems, pushing researchers to develop suitable defence mechanisms.
The use of networks adopting error-correcting output codes (ECOC) has recently been proposed to counter the creation of adversarial examples in a white-box setting.
In this paper, we carry out an in-depth investigation of the adversarial robustness achieved by the ECOC approach.
We do so by proposing a new adversarial attack specifically designed for multilabel classification architectures, like the ECOC-based one, and by applying two existing attacks.
In contrast to previous findings, our analysis reveals that ECOC-based networks can be attacked quite easily by introducing a small adversarial perturbation.
Moreover, the adversarial examples can be generated in such a way to achieve high probabilities for the predicted target class, hence making it difficult to use the prediction confidence to detect them.
Our findings are proven by means of experimental results obtained on MNIST, CIFAR-10, and GTSRB classification tasks.
American Psychological Association (APA)
Zhang, Bowen& Tondi, Benedetta& Lv, Xixiang& Barni, Mauro. 2020. Challenging the Adversarial Robustness of DNNs Based on Error-Correcting Output Codes. Security and Communication Networks،Vol. 2020, no. 2020, pp.1-11.
https://search.emarefa.net/detail/BIM-1208859
Modern Language Association (MLA)
Zhang, Bowen…[et al.]. Challenging the Adversarial Robustness of DNNs Based on Error-Correcting Output Codes. Security and Communication Networks No. 2020 (2020), pp.1-11.
https://search.emarefa.net/detail/BIM-1208859
American Medical Association (AMA)
Zhang, Bowen& Tondi, Benedetta& Lv, Xixiang& Barni, Mauro. Challenging the Adversarial Robustness of DNNs Based on Error-Correcting Output Codes. Security and Communication Networks. 2020. Vol. 2020, no. 2020, pp.1-11.
https://search.emarefa.net/detail/BIM-1208859
Data Type
Journal Articles
Language
English
Notes
Includes bibliographical references
Record ID
BIM-1208859