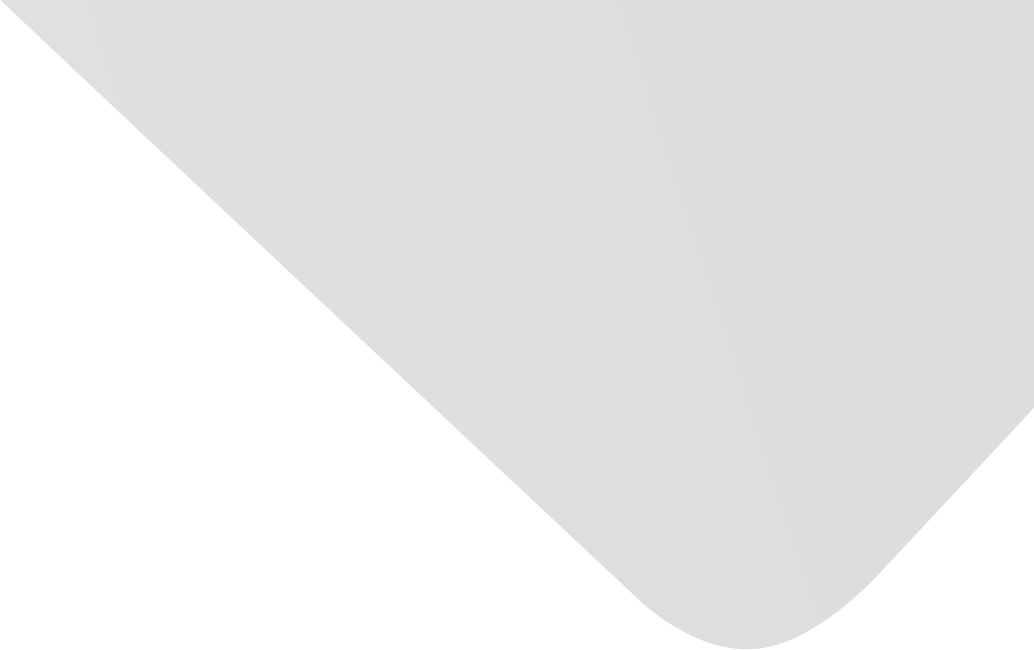
Key-Skeleton-Pattern Mining on 3D Skeletons Represented by Lie Group for Action Recognition
Joint Authors
Li, Guang
Liu, Kai
Ding, Wenwen
Cheng, Fei
Chen, Boyang
Source
Mathematical Problems in Engineering
Issue
Vol. 2018, Issue 2018 (31 Dec. 2018), pp.1-14, 14 p.
Publisher
Hindawi Publishing Corporation
Publication Date
2018-12-05
Country of Publication
Egypt
No. of Pages
14
Main Subjects
Abstract EN
The human skeleton can be considered as a tree system of rigid bodies connected by bone joints.
In recent researches, substantial progress has been made in both theories and experiments on skeleton-based action recognition.
However, it is challenging to accurately represent the skeleton and precisely eliminate noisy skeletons from the action sequence.
This paper proposes a novel skeletal representation, which is composed of two subfeatures to recognize human action: static features and dynamic features.
First, to avoid scale variations from subject to subject, the orientations of the rigid bodies in a skeleton are employed to capture the scale-invariant spatial information of the skeleton.
The static feature of the skeleton is defined as a combination of the orientations.
Unlike previous orientation-based representations, the orientation of a rigid body in the skeleton is defined as the rotations between the rigid body and the coordinate axes in three-dimensional space.
Each rotation is mapped to the special orthogonal group SO(3).
Next, the rigid-body motions between the skeleton and its previous skeletons are utilized to capture the temporal information of the skeleton.
The dynamic feature of the skeleton is defined as a combination of the motions.
Similarly, the motions are represented as points in the special Euclidean group SE(3).
Therefore, the proposed skeleton representation lies in the Lie group (SE(3)×⋯×SE(3), SO(3)×⋯×SO(3)), which is a manifold.
Using the proposed representation, an action can be considered as a series of points in this Lie group.
Then, to recognize human action more accurately, a new pattern-growth algorithm named MinP-PrefixSpan is proposed to mine the key-skeleton-patterns from the training dataset.
Because the algorithm reduces the number of new patterns in each growth step, it is more efficient than the PrefixSpan algorithm.
Finally, the key-skeleton-patterns are used to discover the most informative skeleton sequences of each action (skeleton sequence).
Our approach achieves accuracies of 94.70%, 98.87%, and 95.01% on three action datasets, outperforming other relative action recognition approaches, including LieNet, Lie group, Grassmann manifold, and Graph-based model.
American Psychological Association (APA)
Li, Guang& Liu, Kai& Ding, Wenwen& Cheng, Fei& Chen, Boyang. 2018. Key-Skeleton-Pattern Mining on 3D Skeletons Represented by Lie Group for Action Recognition. Mathematical Problems in Engineering،Vol. 2018, no. 2018, pp.1-14.
https://search.emarefa.net/detail/BIM-1209076
Modern Language Association (MLA)
Li, Guang…[et al.]. Key-Skeleton-Pattern Mining on 3D Skeletons Represented by Lie Group for Action Recognition. Mathematical Problems in Engineering No. 2018 (2018), pp.1-14.
https://search.emarefa.net/detail/BIM-1209076
American Medical Association (AMA)
Li, Guang& Liu, Kai& Ding, Wenwen& Cheng, Fei& Chen, Boyang. Key-Skeleton-Pattern Mining on 3D Skeletons Represented by Lie Group for Action Recognition. Mathematical Problems in Engineering. 2018. Vol. 2018, no. 2018, pp.1-14.
https://search.emarefa.net/detail/BIM-1209076
Data Type
Journal Articles
Language
English
Notes
Includes bibliographical references
Record ID
BIM-1209076