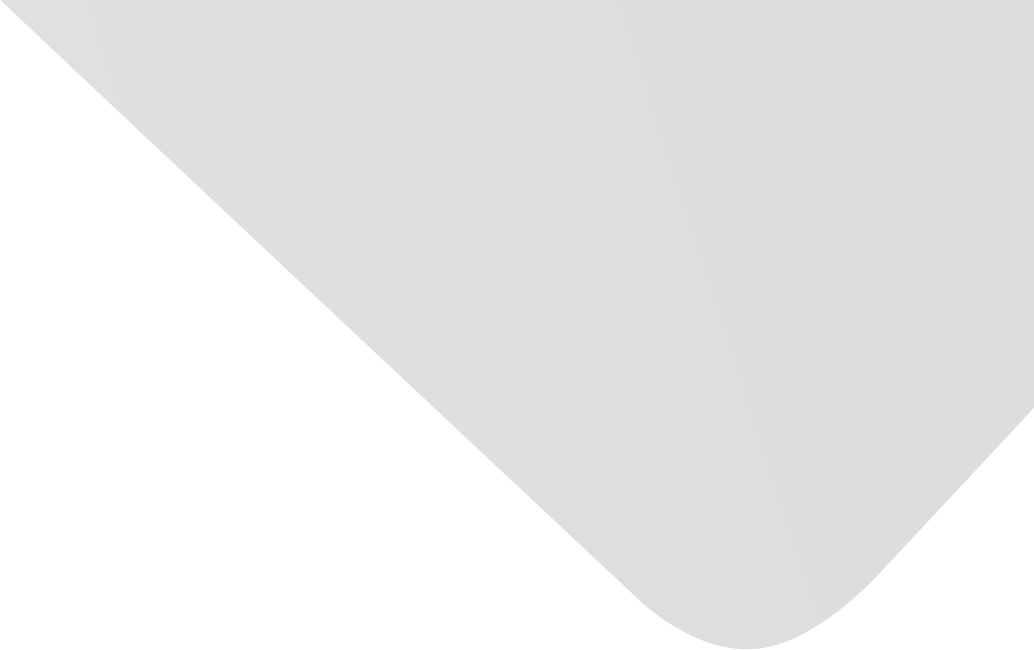
A Neural Network Based Intelligent Support Model for Program Code Completion
Joint Authors
Rahman, Md. Mostafizer
Watanobe, Yutaka
Nakamura, Keita
Source
Issue
Vol. 2020, Issue 2020 (31 Dec. 2020), pp.1-18, 18 p.
Publisher
Hindawi Publishing Corporation
Publication Date
2020-07-14
Country of Publication
Egypt
No. of Pages
18
Main Subjects
Abstract EN
In recent years, millions of source codes are generated in different languages on a daily basis all over the world.
A deep neural network-based intelligent support model for source code completion would be a great advantage in software engineering and programming education fields.
Vast numbers of syntax, logical, and other critical errors that cannot be detected by normal compilers continue to exist in source codes, and the development of an intelligent evaluation methodology that does not rely on manual compilation has become essential.
Even experienced programmers often find it necessary to analyze an entire program in order to find a single error and are thus being forced to waste valuable time debugging their source codes.
With this point in mind, we proposed an intelligent model that is based on long short-term memory (LSTM) and combined it with an attention mechanism for source code completion.
Thus, the proposed model can detect source code errors with locations and then predict the correct words.
In addition, the proposed model can classify the source codes as to whether they are erroneous or not.
We trained our proposed model using the source code and then evaluated the performance.
All of the data used in our experiments were extracted from Aizu Online Judge (AOJ) system.
The experimental results obtained show that the accuracy in terms of error detection and prediction of our proposed model approximately is 62% and source code classification accuracy is approximately 96% which outperformed a standard LSTM and other state-of-the-art models.
Moreover, in comparison to state-of-the-art models, our proposed model achieved an interesting level of success in terms of error detection, prediction, and classification when applied to long source code sequences.
Overall, these experimental results indicate the usefulness of our proposed model in software engineering and programming education arena.
American Psychological Association (APA)
Rahman, Md. Mostafizer& Watanobe, Yutaka& Nakamura, Keita. 2020. A Neural Network Based Intelligent Support Model for Program Code Completion. Scientific Programming،Vol. 2020, no. 2020, pp.1-18.
https://search.emarefa.net/detail/BIM-1209112
Modern Language Association (MLA)
Rahman, Md. Mostafizer…[et al.]. A Neural Network Based Intelligent Support Model for Program Code Completion. Scientific Programming No. 2020 (2020), pp.1-18.
https://search.emarefa.net/detail/BIM-1209112
American Medical Association (AMA)
Rahman, Md. Mostafizer& Watanobe, Yutaka& Nakamura, Keita. A Neural Network Based Intelligent Support Model for Program Code Completion. Scientific Programming. 2020. Vol. 2020, no. 2020, pp.1-18.
https://search.emarefa.net/detail/BIM-1209112
Data Type
Journal Articles
Language
English
Notes
Includes bibliographical references
Record ID
BIM-1209112