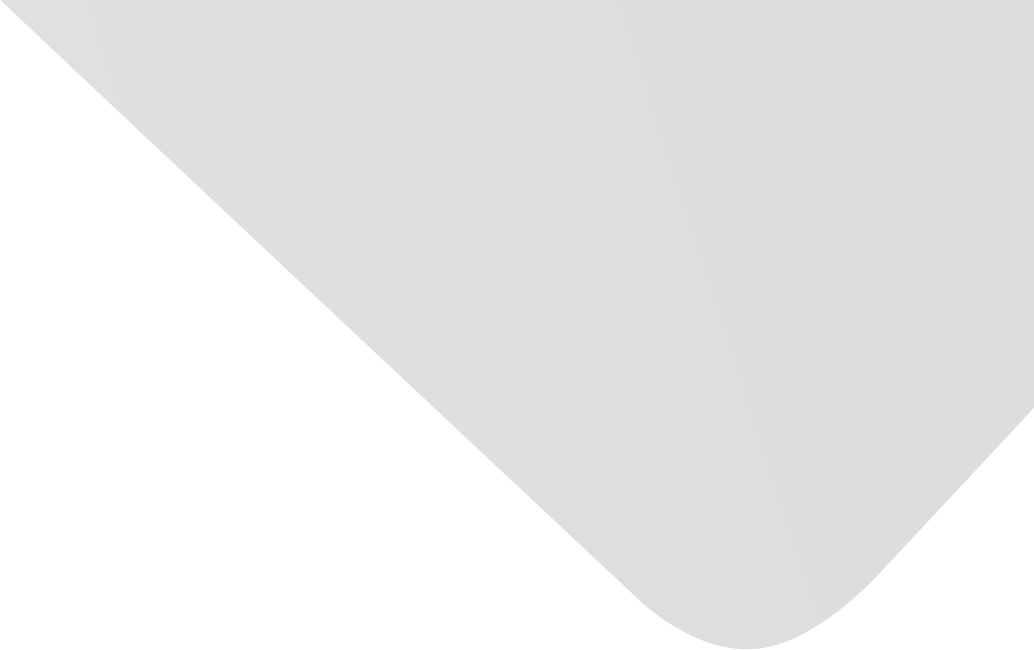
Optimal Policy Learning for Disease Prevention Using Reinforcement Learning
Joint Authors
Mahmoud, Marwan
Al-Khasawneh, Mahmoud Ahmad
Imtiaz, Muhammad
Uddin, M. Irfan
Shah, Syed Atif Ali
Alam Khan, Zahid
Feng, Zhengyong
Mast, Noor
Source
Issue
Vol. 2020, Issue 2020 (31 Dec. 2020), pp.1-13, 13 p.
Publisher
Hindawi Publishing Corporation
Publication Date
2020-11-28
Country of Publication
Egypt
No. of Pages
13
Main Subjects
Abstract EN
Diseases can have a huge impact on the quality of life of the human population.
Humans have always been in the quest to find strategies to avoid diseases that are life-threatening or affect the quality of life of humans.
Effective use of resources available to human to control different diseases has always been critical.
Researchers are recently more interested to find AI-based solutions to control the human population from diseases due to the overwhelming popularity of deep learning.
There are many supervised techniques that have always been applied for disease diagnosis.
However, the main problem of supervised based solutions is the availability of data, which is not always possible or not always complete.
For instance, we do not have enough data that shows the different states of humans and different states of environments, and how all different actions taken by humans or viruses have ultimately resulted in a disease that eventually takes the lives of humans.
Therefore, there is a need to find unsupervised based solutions or some techniques that do not have a dependency on the underlying dataset.
In this paper, we have explored the reinforcement learning approach.
We have tried different reinforcement learning algorithms to research different solutions for the prevention of diseases in the simulation of the human population.
We have explored different techniques for controlling the transmission of diseases and its effects on health in the human population simulated in an environment.
Our algorithms have found out policies that are best for the human population to protect themselves from the transmission and infection of malaria.
The paper concludes that deep learning-based algorithms such as Deep Deterministic Policy Gradient (DDPG) have outperformed traditional algorithms such as Q-Learning or SARSA.
American Psychological Association (APA)
Alam Khan, Zahid& Feng, Zhengyong& Uddin, M. Irfan& Mast, Noor& Shah, Syed Atif Ali& Imtiaz, Muhammad…[et al.]. 2020. Optimal Policy Learning for Disease Prevention Using Reinforcement Learning. Scientific Programming،Vol. 2020, no. 2020, pp.1-13.
https://search.emarefa.net/detail/BIM-1209121
Modern Language Association (MLA)
Alam Khan, Zahid…[et al.]. Optimal Policy Learning for Disease Prevention Using Reinforcement Learning. Scientific Programming No. 2020 (2020), pp.1-13.
https://search.emarefa.net/detail/BIM-1209121
American Medical Association (AMA)
Alam Khan, Zahid& Feng, Zhengyong& Uddin, M. Irfan& Mast, Noor& Shah, Syed Atif Ali& Imtiaz, Muhammad…[et al.]. Optimal Policy Learning for Disease Prevention Using Reinforcement Learning. Scientific Programming. 2020. Vol. 2020, no. 2020, pp.1-13.
https://search.emarefa.net/detail/BIM-1209121
Data Type
Journal Articles
Language
English
Notes
Includes bibliographical references
Record ID
BIM-1209121