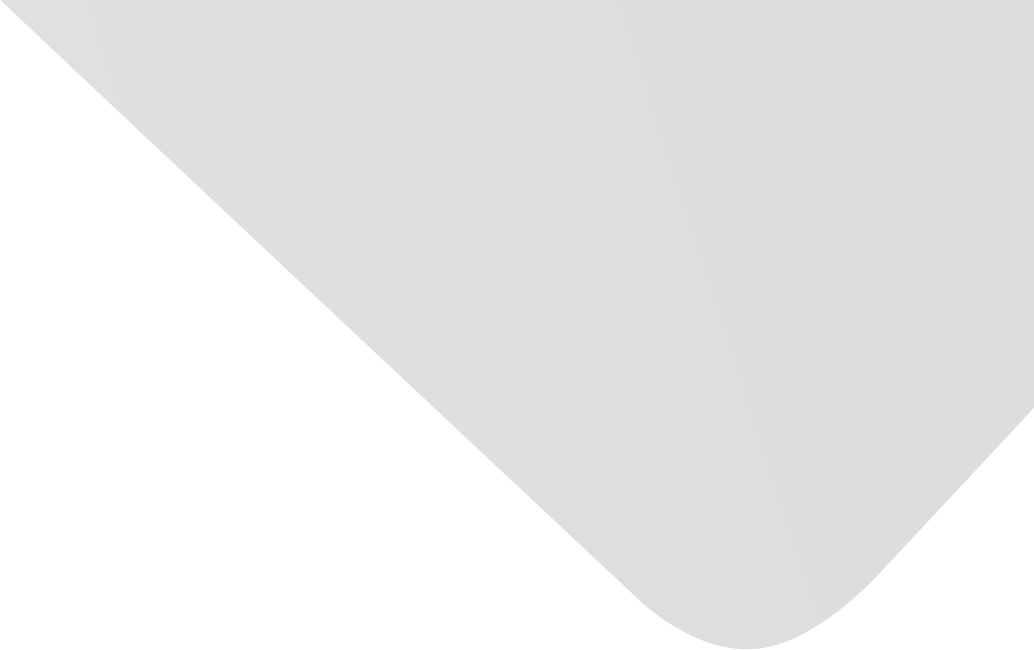
Multitask Convolutional Neural Network for Rolling Element Bearing Fault Identification
Joint Authors
Xu, Yuemei
Jia, Mingxing
Hong, Maoyi
Hu, Xiyu
Source
Issue
Vol. 2020, Issue 2020 (31 Dec. 2020), pp.1-10, 10 p.
Publisher
Hindawi Publishing Corporation
Publication Date
2020-07-14
Country of Publication
Egypt
No. of Pages
10
Main Subjects
Abstract EN
As one of the most vital parts of rotating equipment, it is an essential work to diagnose rolling bearing failure.
The traditional signal processing-based rolling bearing fault diagnosis algorithms rely on artificial feature extraction and expert knowledge.
The working condition of rolling bearings is complex and changeable, so the traditional algorithm is slightly lacking adaptability.
The damage degree also plays a crucial role in fault monitoring.
Different damage degrees may take different remedial measures, but traditional fault-diagnosis algorithms roughly divide the damage degree into several categories, which do not correspond to the continuous value of the damage degree.
To solve the abovementioned two problems, this paper proposes a fault-diagnosis algorithm based on “end-to-end” one-dimensional convolutional neural network.
The one-dimensional convolution kernel and the pooling layer are directly applied to the original time domain signal.
Feature extraction and classifier are merged together, and the extracted features are used to judge the damage degree at the same time.
Then, the generalization ability of the model is studied under a variety of conditions.
Experiments show that the algorithm can achieve more than 99% accuracy and can accurately give the damage degree of the bearing.
It has good performance under different speeds, different types of motors, and different sampling frequencies, and so it has good generalization ability.
American Psychological Association (APA)
Jia, Mingxing& Xu, Yuemei& Hong, Maoyi& Hu, Xiyu. 2020. Multitask Convolutional Neural Network for Rolling Element Bearing Fault Identification. Shock and Vibration،Vol. 2020, no. 2020, pp.1-10.
https://search.emarefa.net/detail/BIM-1209695
Modern Language Association (MLA)
Jia, Mingxing…[et al.]. Multitask Convolutional Neural Network for Rolling Element Bearing Fault Identification. Shock and Vibration No. 2020 (2020), pp.1-10.
https://search.emarefa.net/detail/BIM-1209695
American Medical Association (AMA)
Jia, Mingxing& Xu, Yuemei& Hong, Maoyi& Hu, Xiyu. Multitask Convolutional Neural Network for Rolling Element Bearing Fault Identification. Shock and Vibration. 2020. Vol. 2020, no. 2020, pp.1-10.
https://search.emarefa.net/detail/BIM-1209695
Data Type
Journal Articles
Language
English
Notes
Includes bibliographical references
Record ID
BIM-1209695