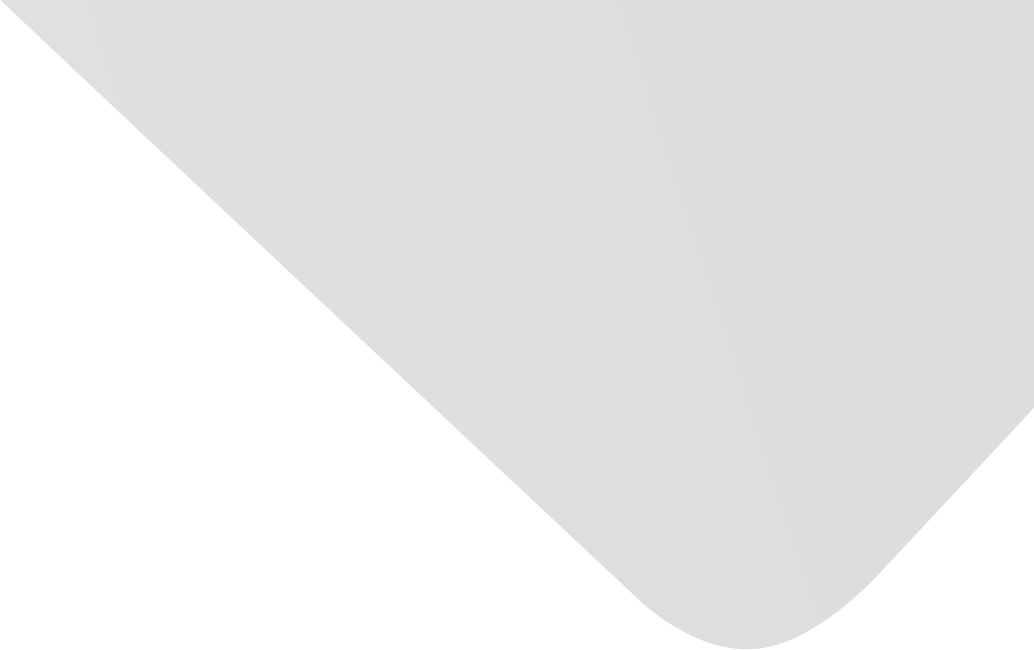
Fault Diagnosis of Oil Pumping Machine Retarder Based on Sound Texture-Vibration Entropy Characteristics and Gray Wolf Optimization-Support Vector Machine
Joint Authors
Zhao, Shutao
Chang, Ke
Wang, Erxu
Li, Bo
Wang, Kedeng
Wu, Qingquan
Source
Issue
Vol. 2020, Issue 2020 (31 Dec. 2020), pp.1-13, 13 p.
Publisher
Hindawi Publishing Corporation
Publication Date
2020-05-11
Country of Publication
Egypt
No. of Pages
13
Main Subjects
Abstract EN
In order to diagnose the retarder faults of oil pumping machine accurately in complex environments and improve the generalization of the algorithm, a GWO-SVM fault diagnosis algorithm based on the combination of sound texture and vibration entropy characteristics was proposed.
Firstly, the acquired sound signal was purified by band-pass filter, then generalized S-transform was developed to extract the box dimension, directivity, and contrast ratio, which reflect the characteristics of time-frequency spectrum, to construct three-dimensional texture eigenvectors.
Secondly, the K parameter of variational mode decomposition (VMD) was reasonably selected by the energy method, and then the vibration signal was decomposed to get modal components, and the permutation entropy was obtained from modal components.
Finally, joint eigenvectors were constructed and fed into SVM for learning.
The gray wolf optimization (GWO) algorithm was used to optimize the parameters of the SVM model based on mixed kernel function, which reduces the impact of sensor frequency response, environmental noise, and load fluctuation disturbance on the accuracy of retarder fault diagnosis.
The results showed that the GWO-SVM fault diagnosis method, which is based on the combination of sound texture and vibration entropy characteristics, makes full use of the complementary advantages of signal frequency band.
And the overall diagnostic accuracy for the experimental samples reaches 100%, which has good generalization ability.
American Psychological Association (APA)
Zhao, Shutao& Chang, Ke& Wang, Erxu& Li, Bo& Wang, Kedeng& Wu, Qingquan. 2020. Fault Diagnosis of Oil Pumping Machine Retarder Based on Sound Texture-Vibration Entropy Characteristics and Gray Wolf Optimization-Support Vector Machine. Shock and Vibration،Vol. 2020, no. 2020, pp.1-13.
https://search.emarefa.net/detail/BIM-1209744
Modern Language Association (MLA)
Zhao, Shutao…[et al.]. Fault Diagnosis of Oil Pumping Machine Retarder Based on Sound Texture-Vibration Entropy Characteristics and Gray Wolf Optimization-Support Vector Machine. Shock and Vibration No. 2020 (2020), pp.1-13.
https://search.emarefa.net/detail/BIM-1209744
American Medical Association (AMA)
Zhao, Shutao& Chang, Ke& Wang, Erxu& Li, Bo& Wang, Kedeng& Wu, Qingquan. Fault Diagnosis of Oil Pumping Machine Retarder Based on Sound Texture-Vibration Entropy Characteristics and Gray Wolf Optimization-Support Vector Machine. Shock and Vibration. 2020. Vol. 2020, no. 2020, pp.1-13.
https://search.emarefa.net/detail/BIM-1209744
Data Type
Journal Articles
Language
English
Notes
Includes bibliographical references
Record ID
BIM-1209744