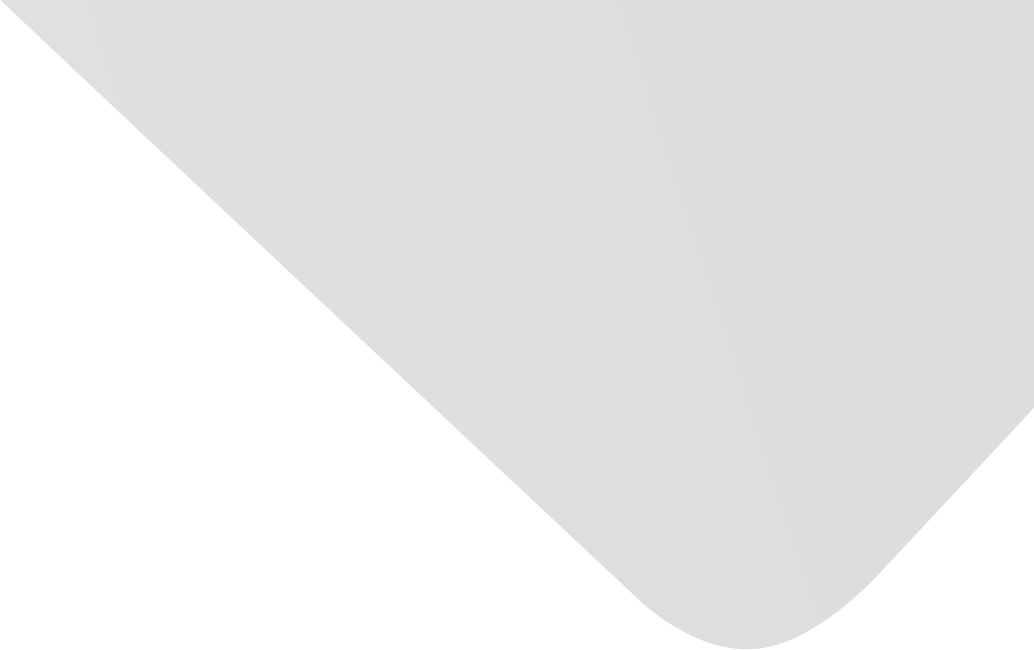
Research on Rolling Bearing Fault Diagnosis Using Improved Majorization-Minimization-Based Total Variation and Empirical Wavelet Transform
Joint Authors
Li, Wenjie
Ou, Yangli
He, Shuilong
Hu, Chaofan
Bao, Jiading
Source
Issue
Vol. 2020, Issue 2020 (31 Dec. 2020), pp.1-11, 11 p.
Publisher
Hindawi Publishing Corporation
Publication Date
2020-05-15
Country of Publication
Egypt
No. of Pages
11
Main Subjects
Abstract EN
Bearings are among the most widely used core components in mechanical equipment.
Their failure creates the potential for serious accidents and economic losses.
Vibration signature analyses are the most common approach to assess the viability of bearings due to its ease of measurement and high correlation with structural dynamics.
However, the collected vibration signals of rolling bearings are usually nonstationary and are inevitably accompanied by noise interference.
This makes it difficult to extract the feature frequency for the failed bearing and affects the diagnosis accuracy.
The majorization-minimization-based total variation (TV-MM) denoising algorithm effectively removes the noise interference from the signal and highlights the related feature information.
The value of its main parameter λ determines the quality of the denoising effect.
However, manually selecting parameters requires professional experience in a process that it is time-consuming and laborious, while the use of genetic algorithms is cumbersome.
Therefore, an improved particle swarm algorithm (IPSO) is used to find the optimal solution of λ.
The IPSO utilises the mutation concept in genetic algorithms to reinitialise the particles with a certain probability after each update.
In addition, the empirical wavelet transform (EWT) is an adaptive signal processing method suitable for processing nonlinear and nonstationary signals.
Therefore, this paper presents an ensemble analysis method that combines the IPSO, TV-MM, and EWT.
First, IPSO is used to optimise the denoising parameter λ.
The TV-MM under this parameter effectively removes the background noise interference and improves the accuracy of the subsequent modal decomposition.
Then, the EWT is used for the adaptive division to produce a set of sequences.
Finally, Hilbert envelope demodulation is performed on each component to realise fault diagnosis.
The results from simulations and signals received from defective bearings with outer race fault, inner race fault, and rolling element fault demonstrate the effectiveness of the proposed method for fault diagnosis of rolling bearings.
American Psychological Association (APA)
Ou, Yangli& He, Shuilong& Hu, Chaofan& Bao, Jiading& Li, Wenjie. 2020. Research on Rolling Bearing Fault Diagnosis Using Improved Majorization-Minimization-Based Total Variation and Empirical Wavelet Transform. Shock and Vibration،Vol. 2020, no. 2020, pp.1-11.
https://search.emarefa.net/detail/BIM-1209811
Modern Language Association (MLA)
Ou, Yangli…[et al.]. Research on Rolling Bearing Fault Diagnosis Using Improved Majorization-Minimization-Based Total Variation and Empirical Wavelet Transform. Shock and Vibration No. 2020 (2020), pp.1-11.
https://search.emarefa.net/detail/BIM-1209811
American Medical Association (AMA)
Ou, Yangli& He, Shuilong& Hu, Chaofan& Bao, Jiading& Li, Wenjie. Research on Rolling Bearing Fault Diagnosis Using Improved Majorization-Minimization-Based Total Variation and Empirical Wavelet Transform. Shock and Vibration. 2020. Vol. 2020, no. 2020, pp.1-11.
https://search.emarefa.net/detail/BIM-1209811
Data Type
Journal Articles
Language
English
Notes
Includes bibliographical references
Record ID
BIM-1209811