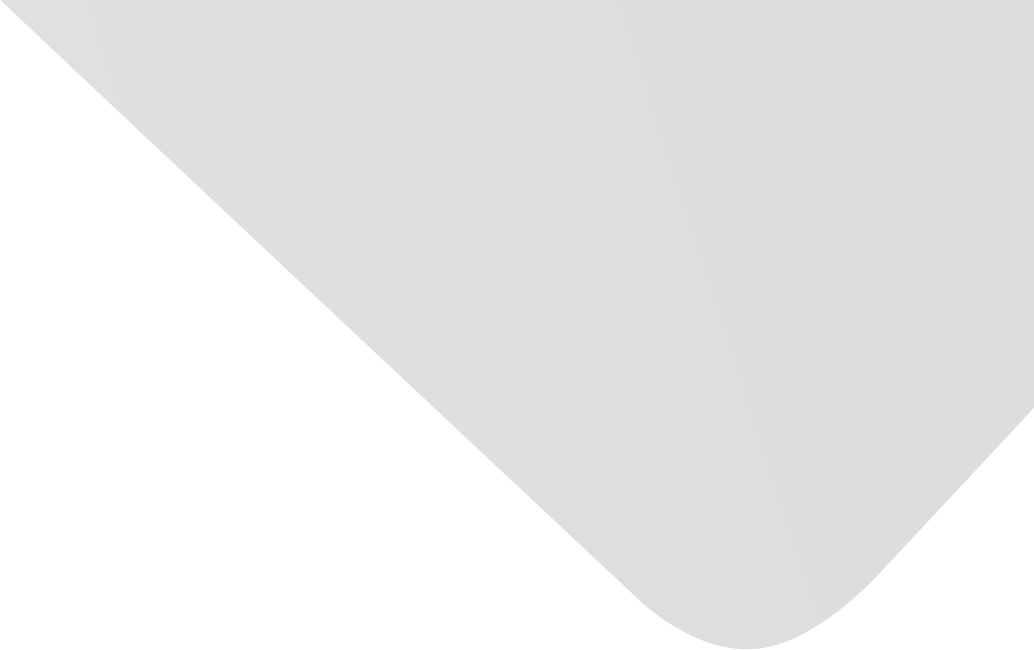
Detecting Shilling Attacks with Automatic Features from Multiple Views
Joint Authors
Zhao, Qingshan
Cao, Jianfang
Hao, Yaojun
Zhang, Fuzhi
Wang, Jian
Source
Security and Communication Networks
Issue
Vol. 2019, Issue 2019 (31 Dec. 2019), pp.1-13, 13 p.
Publisher
Hindawi Publishing Corporation
Publication Date
2019-08-05
Country of Publication
Egypt
No. of Pages
13
Main Subjects
Information Technology and Computer Science
Abstract EN
Due to the openness of the recommender systems, the attackers are likely to inject a large number of fake profiles to bias the prediction of such systems.
The traditional detection methods mainly rely on the artificial features, which are often extracted from one kind of user-generated information.
In these methods, fine-grained interactions between users and items cannot be captured comprehensively, leading to the degradation of detection accuracy under various types of attacks.
In this paper, we propose an ensemble detection method based on the automatic features extracted from multiple views.
Firstly, to collaboratively discover the shilling profiles, the users’ behaviors are analyzed from multiple views including ratings, item popularity, and user-user graph.
Secondly, based on the data preprocessed from multiple views, the stacked denoising autoencoders are used to automatically extract user features with different corruption rates.
Moreover, the features extracted from multiple views are effectively combined based on principal component analysis.
Finally, according to the features extracted with different corruption rates, the weak classifiers are generated and then integrated to detect attacks.
The experimental results on the MovieLens, Netflix, and Amazon datasets indicate that the proposed method can effectively detect various attacks.
American Psychological Association (APA)
Hao, Yaojun& Zhang, Fuzhi& Wang, Jian& Zhao, Qingshan& Cao, Jianfang. 2019. Detecting Shilling Attacks with Automatic Features from Multiple Views. Security and Communication Networks،Vol. 2019, no. 2019, pp.1-13.
https://search.emarefa.net/detail/BIM-1210516
Modern Language Association (MLA)
Hao, Yaojun…[et al.]. Detecting Shilling Attacks with Automatic Features from Multiple Views. Security and Communication Networks No. 2019 (2019), pp.1-13.
https://search.emarefa.net/detail/BIM-1210516
American Medical Association (AMA)
Hao, Yaojun& Zhang, Fuzhi& Wang, Jian& Zhao, Qingshan& Cao, Jianfang. Detecting Shilling Attacks with Automatic Features from Multiple Views. Security and Communication Networks. 2019. Vol. 2019, no. 2019, pp.1-13.
https://search.emarefa.net/detail/BIM-1210516
Data Type
Journal Articles
Language
English
Notes
Includes bibliographical references
Record ID
BIM-1210516