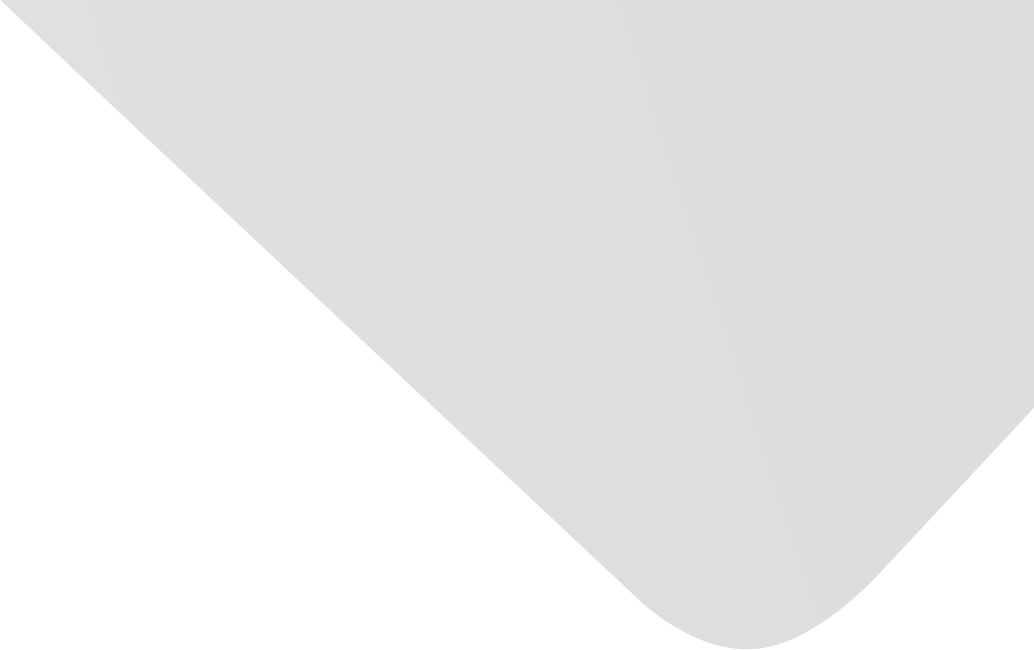
Effects of Challenging Weather and Illumination on Learning-Based License Plate Detection in Noncontrolled Environments
Joint Authors
Rio-Alvarez, A.
de Andres-Suarez, J.
Gonzalez-Rodriguez, M.
Fernandez-Lanvin, D.
López Pérez, B.
Source
Issue
Vol. 2019, Issue 2019 (31 Dec. 2019), pp.1-16, 16 p.
Publisher
Hindawi Publishing Corporation
Publication Date
2019-06-27
Country of Publication
Egypt
No. of Pages
16
Main Subjects
Abstract EN
License Plate Detection (LPD) is one of the most important steps of an Automatic License Plate Recognition (ALPR) system because it is the seed of the entire recognition process.
In indoor controlled environments, there are many effective methods for detecting license plates.
However, outdoors LPD is still a challenge due to the large number of factors that may affect the process and the results obtained.
It is an evidence that a complete training set of images including as many as possible license plates angles and sizes improves the performance of every classifier.
On this line of work, numerous training sets contain images taken under different weather conditions.
However, no studies tested the differences in the effectiveness of different descriptors for these different conditions.
In this paper, various classifiers were trained with features extracted from a set of rainfall images using different kinds of texture-based descriptors.
The accuracy of these specific trained classifiers over a test set of rainfall images was compared with the accuracy of the same descriptor-classifier pair trained with features extracted from an ideal conditions images set.
In the same way, we repeat the experiment with images affected by challenging illumination.
The research concludes, on one hand, that including images affected by rain, snow, or fog in the training sets does not improve the accuracy of the classifier detecting license plates over images affected by these weather conditions.
Classifiers trained with ideal conditions images improve the accuracy of license plate detection in images affected by rainfalls up to 19% depending on the kind of extracted features.
However, on the other hand, results evidence that including images affected by low illumination regardless of the kind of the selected feature increases the accuracy of the classifier up to 29%.
American Psychological Association (APA)
Rio-Alvarez, A.& de Andres-Suarez, J.& Gonzalez-Rodriguez, M.& Fernandez-Lanvin, D.& López Pérez, B.. 2019. Effects of Challenging Weather and Illumination on Learning-Based License Plate Detection in Noncontrolled Environments. Scientific Programming،Vol. 2019, no. 2019, pp.1-16.
https://search.emarefa.net/detail/BIM-1210750
Modern Language Association (MLA)
Rio-Alvarez, A.…[et al.]. Effects of Challenging Weather and Illumination on Learning-Based License Plate Detection in Noncontrolled Environments. Scientific Programming No. 2019 (2019), pp.1-16.
https://search.emarefa.net/detail/BIM-1210750
American Medical Association (AMA)
Rio-Alvarez, A.& de Andres-Suarez, J.& Gonzalez-Rodriguez, M.& Fernandez-Lanvin, D.& López Pérez, B.. Effects of Challenging Weather and Illumination on Learning-Based License Plate Detection in Noncontrolled Environments. Scientific Programming. 2019. Vol. 2019, no. 2019, pp.1-16.
https://search.emarefa.net/detail/BIM-1210750
Data Type
Journal Articles
Language
English
Notes
Includes bibliographical references
Record ID
BIM-1210750