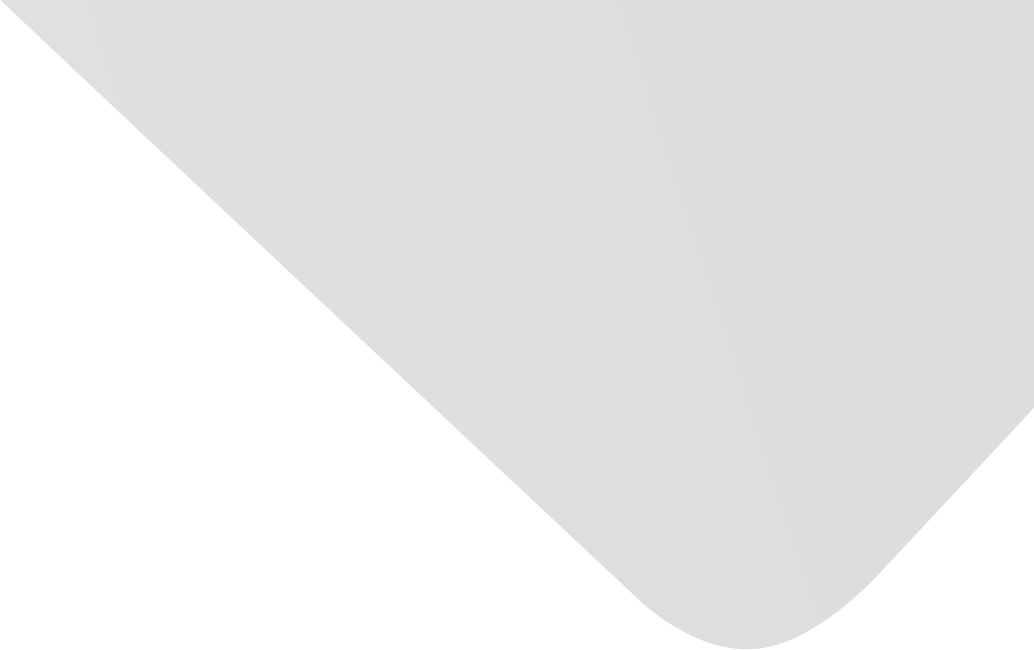
Fine-Grained Fault Diagnosis Method of Rolling Bearing Combining Multisynchrosqueezing Transform and Sparse Feature Coding Based on Dictionary Learning
Joint Authors
Sun, Guodong
Gao, Yuan
Lin, Kai
Hu, Ye
Source
Issue
Vol. 2019, Issue 2019 (31 Dec. 2019), pp.1-13, 13 p.
Publisher
Hindawi Publishing Corporation
Publication Date
2019-11-20
Country of Publication
Egypt
No. of Pages
13
Main Subjects
Abstract EN
To accurately diagnose fine-grained fault of rolling bearing, this paper proposed a new fault diagnosis method combining multisynchrosqueezing transform (MSST) and sparse feature coding based on dictionary learning (SFC-DL).
Firstly, the high-resolution time-frequency images of raw vibration signals, including different kinds of fine-grained faults of rolling bearing, were constructed by MSST.
Then, the basis dictionary was trained through nonnegative matrix factorization with sparseness constraints (NMFSC), and the trained basis dictionary was employed to extract features from time-frequency matrixes by using nonnegative linear equations.
Finally, a linear support vector machine (LSVM) was trained with features of training samples, and the trained LSVM was employed to diagnosis the fault classification of test samples.
Compared with state-of-the-art fault diagnosis methods, the proposed method, which was tested on the bearing dataset from Case Western Reserve University (CWRU), achieved the fine-grained classification of 10 mixed fault states.
Meanwhile, the proposed method was applied on the dataset from the Machinery Failure Prevention Technology (MFPT) Society and realized the classification of 3 fault states under different working conditions.
These results indicate that the proposed method has great robustness and could better meet the needs of practical engineering.
American Psychological Association (APA)
Sun, Guodong& Gao, Yuan& Lin, Kai& Hu, Ye. 2019. Fine-Grained Fault Diagnosis Method of Rolling Bearing Combining Multisynchrosqueezing Transform and Sparse Feature Coding Based on Dictionary Learning. Shock and Vibration،Vol. 2019, no. 2019, pp.1-13.
https://search.emarefa.net/detail/BIM-1210945
Modern Language Association (MLA)
Sun, Guodong…[et al.]. Fine-Grained Fault Diagnosis Method of Rolling Bearing Combining Multisynchrosqueezing Transform and Sparse Feature Coding Based on Dictionary Learning. Shock and Vibration No. 2019 (2019), pp.1-13.
https://search.emarefa.net/detail/BIM-1210945
American Medical Association (AMA)
Sun, Guodong& Gao, Yuan& Lin, Kai& Hu, Ye. Fine-Grained Fault Diagnosis Method of Rolling Bearing Combining Multisynchrosqueezing Transform and Sparse Feature Coding Based on Dictionary Learning. Shock and Vibration. 2019. Vol. 2019, no. 2019, pp.1-13.
https://search.emarefa.net/detail/BIM-1210945
Data Type
Journal Articles
Language
English
Notes
Includes bibliographical references
Record ID
BIM-1210945