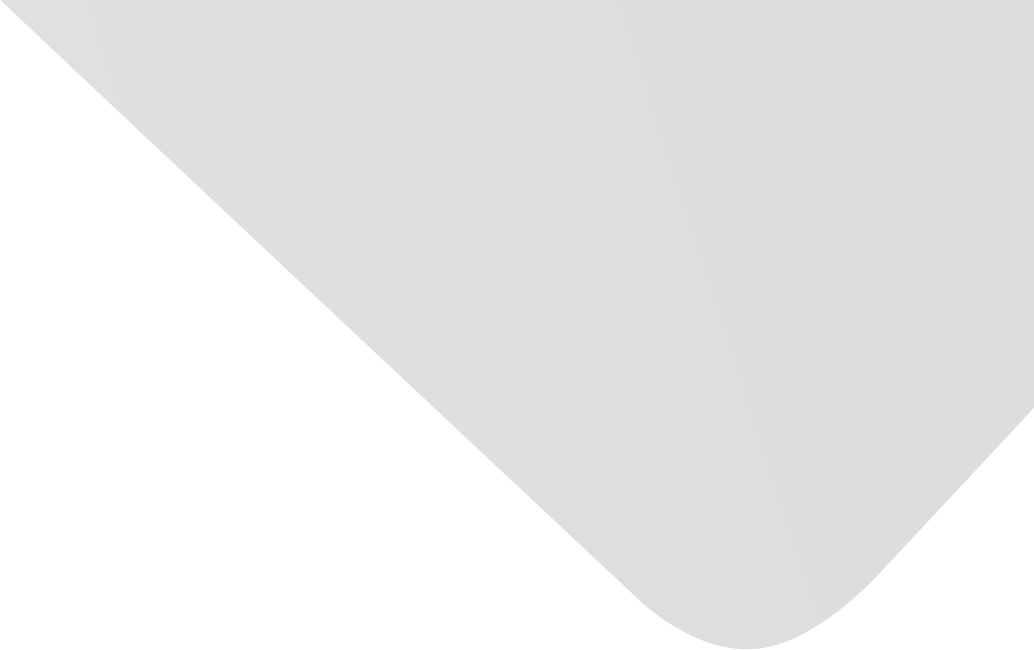
A Fault Diagnosis Model for Rotating Machinery Using VWC and MSFLA-SVM Based on Vibration Signal Analysis
Joint Authors
You, Lei
Fan, Wenjie
Li, Zongwen
Liang, Ying
Fang, Miao
Wang, Jin
Source
Issue
Vol. 2019, Issue 2019 (31 Dec. 2019), pp.1-16, 16 p.
Publisher
Hindawi Publishing Corporation
Publication Date
2019-02-12
Country of Publication
Egypt
No. of Pages
16
Main Subjects
Abstract EN
Fault diagnosis of rotating machinery mainly includes fault feature extraction and fault classification.
Vibration signal from the operation of machinery usually could help diagnosing the operational state of equipment.
Different types of fault usually have different vibrational features, which are actually the basis of fault diagnosis.
This paper proposes a novel fault diagnosis model, which extracts features by combining vibration severity, dyadic wavelet energy time-spectrum, and coefficient power spectrum of the maximum wavelet energy level (VWC) at the feature extraction stage.
At the stage of fault classification, we design a support vector machine (SVM) based on the modified shuffled frog-leaping algorithm (MSFLA) for the accurate classifying machinery fault method.
Specifically, we use the MSFLA method to optimize SVM parameters.
MSFLA can avoid getting trapped into local optimum, speeding up convergence, and improving classification accuracy.
Finally, we evaluate our model on real rotating machinery platform, which has four different states, i.e., normal state, eccentric axle fault (EAF), bearing pedestal fault (BPF), and sealing ring wear fault (SRWF).
As demonstrated by the results, the VWC method is efficient in extracting vibration signal features of rotating machinery.
Based on the extracted features, we further compare our classification method with other three fault classification methods, i.e., backpropagation neural network (BPNN), artificial chemical reaction optimization algorithm (ACROA-SVM), and SFLA-SVM.
The experiment results show that MSFLA-SVM achieves a much higher fault classification rate than BPNN, ACROA-SVM, and SFLA-SVM.
American Psychological Association (APA)
You, Lei& Fan, Wenjie& Li, Zongwen& Liang, Ying& Fang, Miao& Wang, Jin. 2019. A Fault Diagnosis Model for Rotating Machinery Using VWC and MSFLA-SVM Based on Vibration Signal Analysis. Shock and Vibration،Vol. 2019, no. 2019, pp.1-16.
https://search.emarefa.net/detail/BIM-1210994
Modern Language Association (MLA)
You, Lei…[et al.]. A Fault Diagnosis Model for Rotating Machinery Using VWC and MSFLA-SVM Based on Vibration Signal Analysis. Shock and Vibration No. 2019 (2019), pp.1-16.
https://search.emarefa.net/detail/BIM-1210994
American Medical Association (AMA)
You, Lei& Fan, Wenjie& Li, Zongwen& Liang, Ying& Fang, Miao& Wang, Jin. A Fault Diagnosis Model for Rotating Machinery Using VWC and MSFLA-SVM Based on Vibration Signal Analysis. Shock and Vibration. 2019. Vol. 2019, no. 2019, pp.1-16.
https://search.emarefa.net/detail/BIM-1210994
Data Type
Journal Articles
Language
English
Notes
Includes bibliographical references
Record ID
BIM-1210994