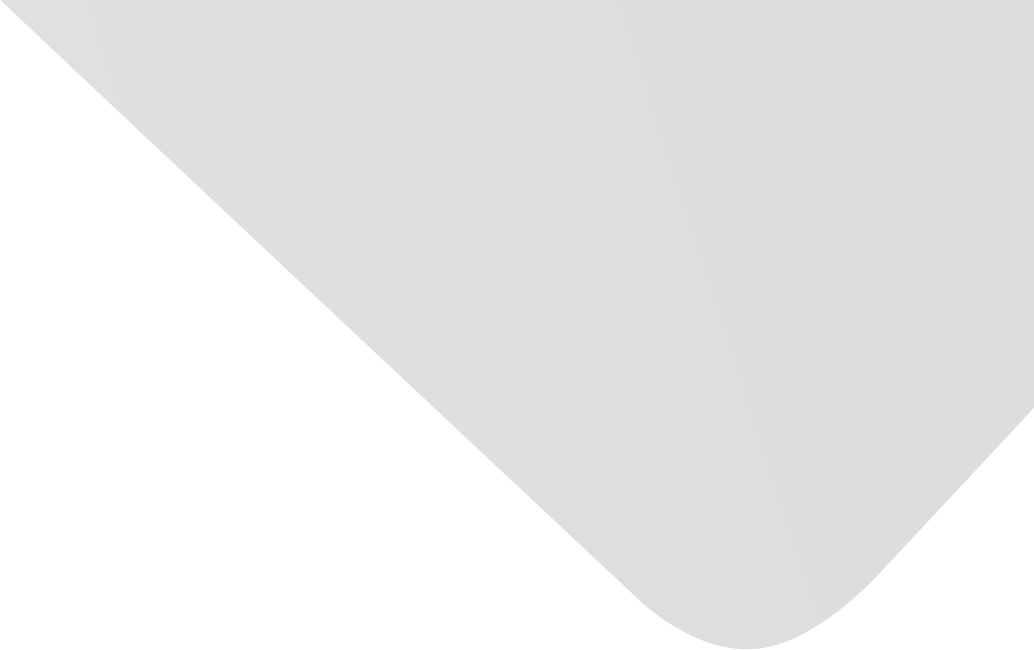
Milling Tool Wear State Recognition by Vibration Signal Using a Stacked Generalization Ensemble Model
Joint Authors
Jiang, Gedong
Hui, Yang
Mei, Xuesong
Tao, Tao
Pei, Changyu
Ma, Ziwei
Source
Issue
Vol. 2019, Issue 2019 (31 Dec. 2019), pp.1-16, 16 p.
Publisher
Hindawi Publishing Corporation
Publication Date
2019-11-03
Country of Publication
Egypt
No. of Pages
16
Main Subjects
Abstract EN
Milling tool wear state recognition plays an important role in controlling the quality of milled parts and reducing machine tool downtime.
However, the characteristics of milling process limit the accuracy and stability of tool condition monitoring (TCM) employing vibration signals.
To improve this problem, this paper explores the use of vibration signals as sensing approach for recognizing tool wear states during milling operation by using the stacked generalization (SG) ensemble model.
In this study, vibration signals collected during the milling process are analyzed through the time domain, frequency domain, and time-frequency domain to extract signal features.
The support vector machine recursive feature elimination (SVM-RFE) algorithm is used to select the main features which are most relevant to tool wear states.
The SG ensemble model based on SVM, decision tree (DT), naive Bayes (NB), and SG ensemble strategy is constructed to recognize tool wear states.
The proposed method is experimental verified, and the results show that the recognition accuracy of the established SG ensemble model is 98.74% and the overall G-mean and AUC evaluation value of the model is 0.98 and 0.98, respectively.
In addition, compared with other ensemble models and single models, the SG ensemble model based on vibration signals has better recognition accuracy and stability than other models.
American Psychological Association (APA)
Hui, Yang& Mei, Xuesong& Jiang, Gedong& Tao, Tao& Pei, Changyu& Ma, Ziwei. 2019. Milling Tool Wear State Recognition by Vibration Signal Using a Stacked Generalization Ensemble Model. Shock and Vibration،Vol. 2019, no. 2019, pp.1-16.
https://search.emarefa.net/detail/BIM-1211515
Modern Language Association (MLA)
Hui, Yang…[et al.]. Milling Tool Wear State Recognition by Vibration Signal Using a Stacked Generalization Ensemble Model. Shock and Vibration No. 2019 (2019), pp.1-16.
https://search.emarefa.net/detail/BIM-1211515
American Medical Association (AMA)
Hui, Yang& Mei, Xuesong& Jiang, Gedong& Tao, Tao& Pei, Changyu& Ma, Ziwei. Milling Tool Wear State Recognition by Vibration Signal Using a Stacked Generalization Ensemble Model. Shock and Vibration. 2019. Vol. 2019, no. 2019, pp.1-16.
https://search.emarefa.net/detail/BIM-1211515
Data Type
Journal Articles
Language
English
Notes
Includes bibliographical references
Record ID
BIM-1211515