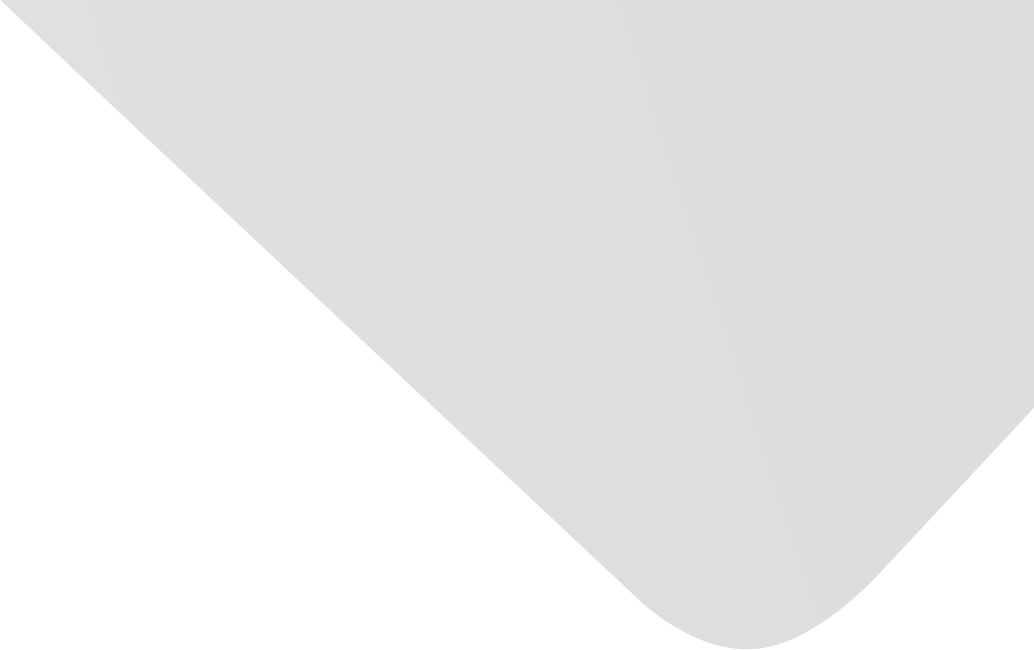
Bearing Fault Diagnosis Using a Support Vector Machine Optimized by an Improved Ant Lion Optimizer
Joint Authors
Wang, Guangbin
Tao, Jie
Yang, Dalian
Shen, Yiping
Miao, Jingjing
Zhang, Fanyu
Source
Issue
Vol. 2019, Issue 2019 (31 Dec. 2019), pp.1-20, 20 p.
Publisher
Hindawi Publishing Corporation
Publication Date
2019-06-27
Country of Publication
Egypt
No. of Pages
20
Main Subjects
Abstract EN
Bearing is an important mechanical component that easily fails in a bad working environment.
Support vector machines can be used to diagnose bearing faults; however, the recognition ability of the model is greatly affected by the kernel function and its parameters.
Unfortunately, optimal parameters are difficult to select.
To address these limitations, an escape mechanism and adaptive convergence conditions were introduced to the ALO algorithm.
As a result, the EALO method was proposed and has been applied to the more accurate selection of SVM model parameters.
To assess the model, the vibration acceleration signals of normal, inner ring fault, outer ring fault, and ball fault bearings were collected at different rotation speeds (1500 r/min, 1800 r/min, 2100 r/min, and 2400 r/min).
The vibration signals were decomposed using the variational mode decomposition (VMD) method.
The features were extracted through the kernel function to fuse the energy value of each VMD component.
In these experiments, the two most important parameters for the support vector machine—the Gaussian kernel parameter σ and the penalty factor C—were optimized using the EALO algorithm, ALO algorithm, genetic algorithm (GA), and particle swarm optimization (PSO) algorithm.
The performance of these four methods to optimize the two parameters was then compared and analyzed, with the EALO method having the best performance.
The recognition rates for bearing faults under different tested rotation speeds were improved when the SVM model parameters optimized by the EALO were used.
American Psychological Association (APA)
Yang, Dalian& Miao, Jingjing& Zhang, Fanyu& Tao, Jie& Wang, Guangbin& Shen, Yiping. 2019. Bearing Fault Diagnosis Using a Support Vector Machine Optimized by an Improved Ant Lion Optimizer. Shock and Vibration،Vol. 2019, no. 2019, pp.1-20.
https://search.emarefa.net/detail/BIM-1211695
Modern Language Association (MLA)
Yang, Dalian…[et al.]. Bearing Fault Diagnosis Using a Support Vector Machine Optimized by an Improved Ant Lion Optimizer. Shock and Vibration No. 2019 (2019), pp.1-20.
https://search.emarefa.net/detail/BIM-1211695
American Medical Association (AMA)
Yang, Dalian& Miao, Jingjing& Zhang, Fanyu& Tao, Jie& Wang, Guangbin& Shen, Yiping. Bearing Fault Diagnosis Using a Support Vector Machine Optimized by an Improved Ant Lion Optimizer. Shock and Vibration. 2019. Vol. 2019, no. 2019, pp.1-20.
https://search.emarefa.net/detail/BIM-1211695
Data Type
Journal Articles
Language
English
Notes
Includes bibliographical references
Record ID
BIM-1211695