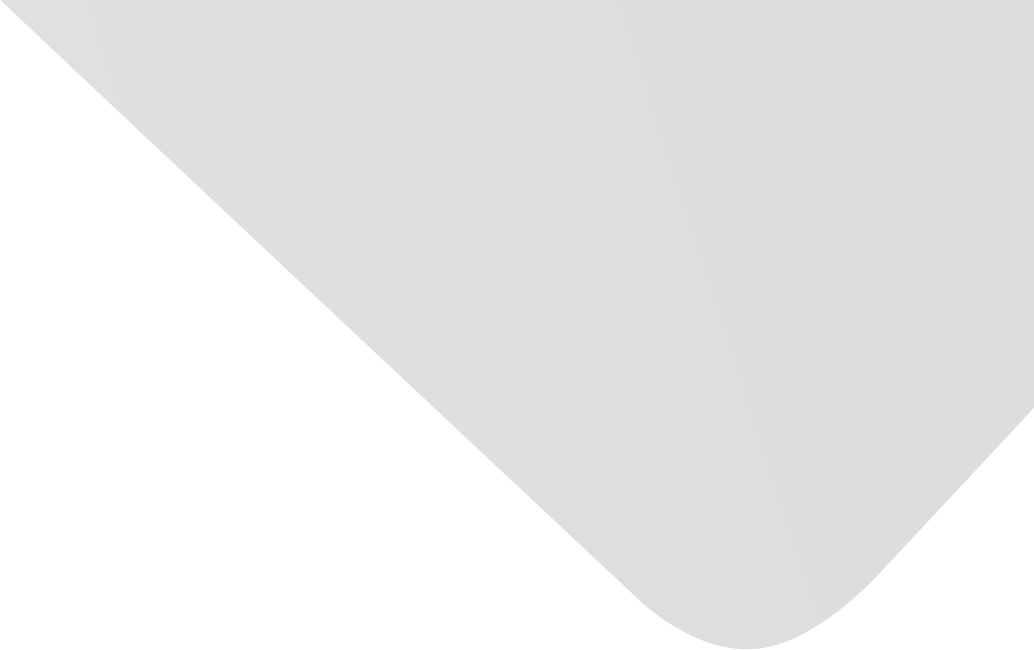
Breast Cancer Detection in the IOT Health Environment Using Modified Recursive Feature Selection
Joint Authors
Haq, Amin Ul
Li, Jian Ping
Memon, Muhammad Hammad
Memon, Muhammad Hunain
Zhou, Wang
Source
Wireless Communications and Mobile Computing
Issue
Vol. 2019, Issue 2019 (31 Dec. 2019), pp.1-19, 19 p.
Publisher
Hindawi Publishing Corporation
Publication Date
2019-11-11
Country of Publication
Egypt
No. of Pages
19
Main Subjects
Information Technology and Computer Science
Abstract EN
The accurate and efficient diagnosis of breast cancer is extremely necessary for recovery and treatment in early stages in IoT healthcare environment.
Internet of Things has witnessed the transition in life for the last few years which provides a way to analyze both the real-time data and past data by the emerging role of artificial intelligence and data mining techniques.
The current state-of-the-art method does not effectively diagnose the breast cancer in the early stages, and most of the ladies suffered from this dangerous disease.
Thus, the early detection of breast cancer significantly poses a great challenge for medical experts and researchers.
To solve the problem of early-stage detection of breast cancer, we proposed machine learning-based diagnostic system which effectively classifies the malignant and benign people in the environment of IoT.
In the development of our proposed system, a machine learning classifier support vector machine is used to classify the malignant and benign people.
To improve the classification performance of the classification system, we used a recursive feature selection algorithm to select more suitable features from the breast cancer dataset.
The training/testing splits method is applied for training and testing of the classifier for the best predictive model.
Additionally, the classifier performance has been checked on by using performance evaluation metrics such as classification, specificity, sensitivity, Matthews’s correlation coefficient, F1-score, and execution time.
To test the proposed method, the dataset “Wisconsin Diagnostic Breast Cancer” has been used in this research study.
The experimental results demonstrate that the recursive feature selection algorithm selects the best subset of features, and the classifier SVM achieved optimal classification performance on this best subset of features.
The SVM kernel linear achieved high classification accuracy (99%), specificity (99%), and sensitivity (98%), and the Matthews’s correlation coefficient is 99%.
From these experimental results, we concluded that the proposed system performance is excellent due to the selection of more appropriate features that are selected by the recursive feature selection algorithm.
Furthermore, we suggest this proposed system for effective and efficient early stages diagnosis of breast cancer.
Thus, through this system, the recovery and treatment will be more effective for breast cancer.
Lastly, the implementation of the proposed system is very reliable in all aspects of IoT healthcare for breast cancer.
American Psychological Association (APA)
Memon, Muhammad Hammad& Li, Jian Ping& Haq, Amin Ul& Memon, Muhammad Hunain& Zhou, Wang. 2019. Breast Cancer Detection in the IOT Health Environment Using Modified Recursive Feature Selection. Wireless Communications and Mobile Computing،Vol. 2019, no. 2019, pp.1-19.
https://search.emarefa.net/detail/BIM-1212166
Modern Language Association (MLA)
Memon, Muhammad Hammad…[et al.]. Breast Cancer Detection in the IOT Health Environment Using Modified Recursive Feature Selection. Wireless Communications and Mobile Computing No. 2019 (2019), pp.1-19.
https://search.emarefa.net/detail/BIM-1212166
American Medical Association (AMA)
Memon, Muhammad Hammad& Li, Jian Ping& Haq, Amin Ul& Memon, Muhammad Hunain& Zhou, Wang. Breast Cancer Detection in the IOT Health Environment Using Modified Recursive Feature Selection. Wireless Communications and Mobile Computing. 2019. Vol. 2019, no. 2019, pp.1-19.
https://search.emarefa.net/detail/BIM-1212166
Data Type
Journal Articles
Language
English
Notes
Includes bibliographical references
Record ID
BIM-1212166