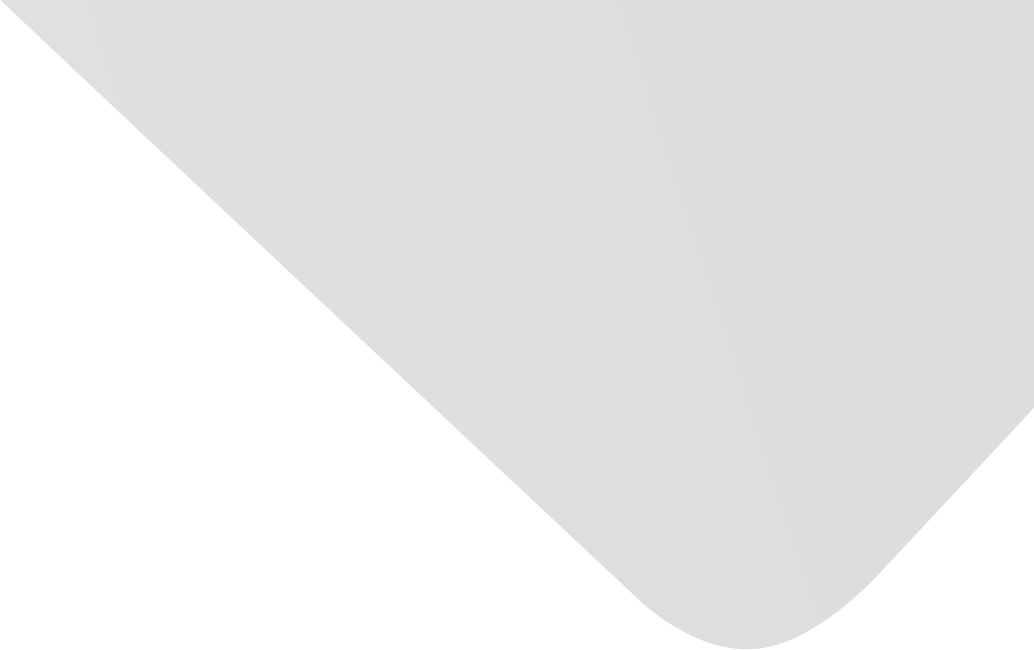
Predicting Fine-Grained Traffic Conditions via Spatio-Temporal LSTM
Joint Authors
Yang, Fangchun
Li, Jinglin
Zhou, Ao
Wei, Xiaojuan
Yuan, Quan
Chen, Kaihui
Source
Wireless Communications and Mobile Computing
Issue
Vol. 2019, Issue 2019 (31 Dec. 2019), pp.1-12, 12 p.
Publisher
Hindawi Publishing Corporation
Publication Date
2019-01-14
Country of Publication
Egypt
No. of Pages
12
Main Subjects
Information Technology and Computer Science
Abstract EN
Predicting traffic conditions for road segments is the prelude of working on intelligent transportation.
Many existing methods can be used for short-term or long-term traffic prediction, but they focus more on regions than on road segments.
The lack of fine-grained traffic predicting approach hinders the development of ITS.
Therefore, MapLSTM, a spatio-temporal long short-term memory network preluded by map-matching, is proposed in this paper to predict fine-grained traffic conditions.
MapLSTM first obtains the historical and real-time traffic conditions of road segments via map-matching.
Then LSTM is used to predict the conditions of the corresponding road segments in the future.
Breaking the single-index forecasting, MapLSTM can predict the vehicle speed, traffic volume, and the travel time in different directions of road segments simultaneously.
Experiments confirmed MapLSTM can not only achieve prediction for road segments based a large scale of GPS trajectories effectively but also have higher predicting accuracy than GPR and ConvLSTM.
Moreover, we demonstrate that MapLSTM can serve various applications in a lightweight way, such as cognizing driving preferences, learning navigation, and inferring traffic emissions.
American Psychological Association (APA)
Wei, Xiaojuan& Li, Jinglin& Yuan, Quan& Chen, Kaihui& Zhou, Ao& Yang, Fangchun. 2019. Predicting Fine-Grained Traffic Conditions via Spatio-Temporal LSTM. Wireless Communications and Mobile Computing،Vol. 2019, no. 2019, pp.1-12.
https://search.emarefa.net/detail/BIM-1212320
Modern Language Association (MLA)
Wei, Xiaojuan…[et al.]. Predicting Fine-Grained Traffic Conditions via Spatio-Temporal LSTM. Wireless Communications and Mobile Computing No. 2019 (2019), pp.1-12.
https://search.emarefa.net/detail/BIM-1212320
American Medical Association (AMA)
Wei, Xiaojuan& Li, Jinglin& Yuan, Quan& Chen, Kaihui& Zhou, Ao& Yang, Fangchun. Predicting Fine-Grained Traffic Conditions via Spatio-Temporal LSTM. Wireless Communications and Mobile Computing. 2019. Vol. 2019, no. 2019, pp.1-12.
https://search.emarefa.net/detail/BIM-1212320
Data Type
Journal Articles
Language
English
Notes
Includes bibliographical references
Record ID
BIM-1212320