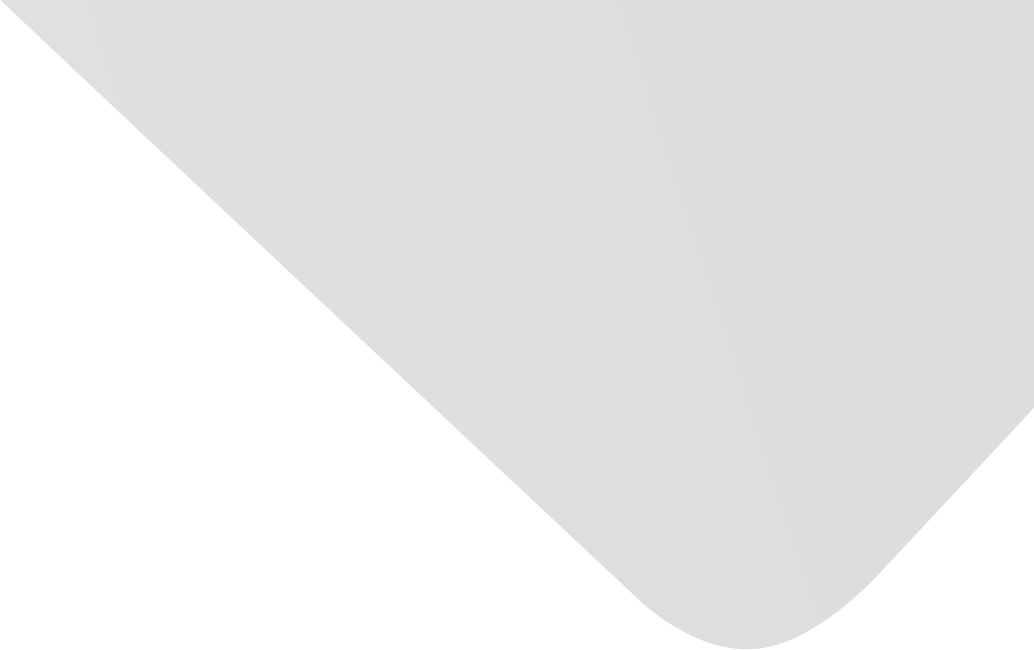
Fault Diagnosis of Planetary Gearbox Based on Motor Current Signal Analysis
Joint Authors
Jiang, Ziyuan
Han, Qinkai
Xu, Xueping
Source
Issue
Vol. 2020, Issue 2020 (31 Dec. 2020), pp.1-13, 13 p.
Publisher
Hindawi Publishing Corporation
Publication Date
2020-09-14
Country of Publication
Egypt
No. of Pages
13
Main Subjects
Abstract EN
Planetary gearbox is one of the most widely used core parts in heavy machinery.
Once it breaks down, it can lead to serious accidents and economic loss.
Induction motor current signal analysis (MCSA) is a noninvasive method that uses current to detect faults.
Currently, most MCSA-based fault diagnosis studies focus on the parallel shaft gearbox.
However, there is a paucity of studies on the planetary gearbox.
The effect of various signal processing methods on motor current and the performance of different machine learning models are rarely compared.
Therefore, fault diagnosis of planetary gearbox based MCSA is conducted in this study.
First, the effects of various faults on motor currents are studied.
Specifically, the characteristic frequencies of a fault in sun/planet/ring gears and supporting bearings of the planetary gearbox are derived.
Then, a signal preprocessing method, namely, singular spectrum analysis (SSA), is proposed to remove the supply frequency component in the current signal.
Subsequently, four classical machine learning models, including the support vector machine (SVM), decision tree (DT), random forest (RF), and AdaBoost, are used for fault classifications based on the features extracted via principal component analysis (PCA).
The convolutional neural network (CNN), which can automatically extract features, is also adopted.
The dynamic experiment of the planetary gearbox with seven types of faults, including tooth chipping in sun/planet/ring gears, inner race spall in planet bearing, inner/outer races, and ball spalls in input support bearing, is conducted.
Raw current signal in the time domain, reconstructed signal by SSA, and the current spectra in the frequency domain are used as the inputs of various models.
The classification results show that the PCA-SVM is the best model for learned data while CNN is the best model for unlearned data on average.
Furthermore, SSA mainly increases the accuracy of CNN in the time domain and exhibits a positive effect on unlearned data in the time domain.
The classification accuracy increases significantly after transforming the time domain current data to the frequency domain.
American Psychological Association (APA)
Jiang, Ziyuan& Han, Qinkai& Xu, Xueping. 2020. Fault Diagnosis of Planetary Gearbox Based on Motor Current Signal Analysis. Shock and Vibration،Vol. 2020, no. 2020, pp.1-13.
https://search.emarefa.net/detail/BIM-1212966
Modern Language Association (MLA)
Jiang, Ziyuan…[et al.]. Fault Diagnosis of Planetary Gearbox Based on Motor Current Signal Analysis. Shock and Vibration No. 2020 (2020), pp.1-13.
https://search.emarefa.net/detail/BIM-1212966
American Medical Association (AMA)
Jiang, Ziyuan& Han, Qinkai& Xu, Xueping. Fault Diagnosis of Planetary Gearbox Based on Motor Current Signal Analysis. Shock and Vibration. 2020. Vol. 2020, no. 2020, pp.1-13.
https://search.emarefa.net/detail/BIM-1212966
Data Type
Journal Articles
Language
English
Notes
Includes bibliographical references
Record ID
BIM-1212966