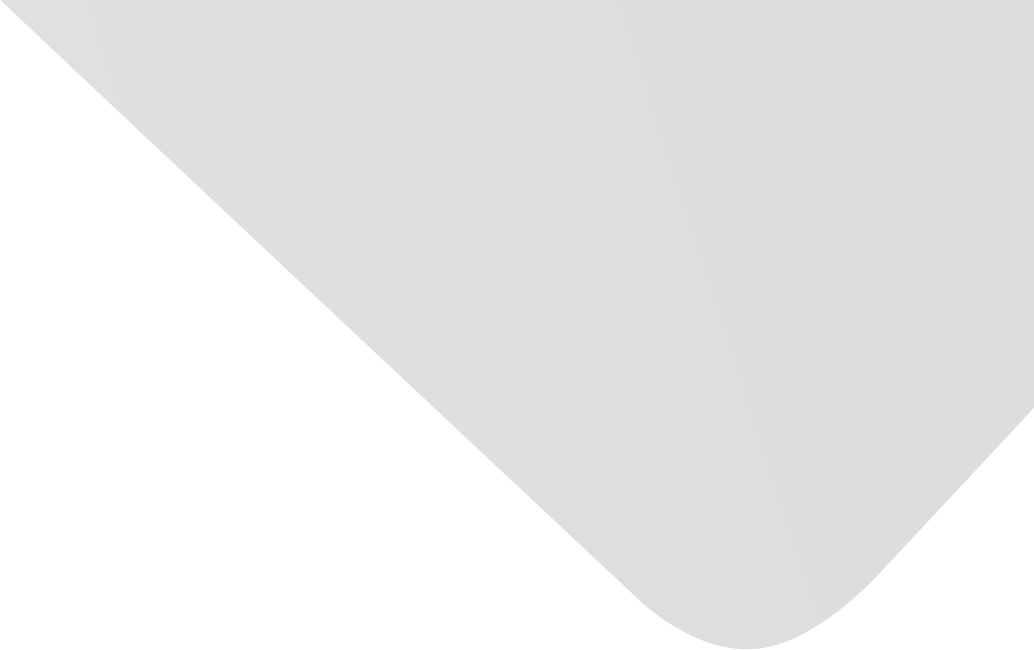
Rolling Bearing Fault Feature Extraction Based on Adaptive Tunable Q-Factor Wavelet Transform and Spectral Kurtosis
Joint Authors
Chen, Qingguang
Zhao, Jianlong
Zhang, Yongchao
Source
Issue
Vol. 2020, Issue 2020 (31 Dec. 2020), pp.1-19, 19 p.
Publisher
Hindawi Publishing Corporation
Publication Date
2020-07-30
Country of Publication
Egypt
No. of Pages
19
Main Subjects
Abstract EN
The fault feature of the rolling bearing is difficult to extract when weak fault occurs and interference exists.
The tunable Q-factor wavelet transform (TQWT) can effectively extract the weak fault characteristic of the rolling bearing, but the manual selection of the Q-factor affects the decomposition result and only using TQWT presents interference.
Aiming at the above problems, an adaptive tunable Q-factor wavelet transform (ATQWT) and spectral kurtosis (SK) method is proposed in this paper.
Firstly, the method applies particle swarm optimization (PSO) to seek the optimized Q-factor for avoiding manual selection, which uses the kurtosis value of the transient impact component as the particle fitness function.
The rolling bearing fault signal is decomposed into continuous oscillation component and transient impact component containing fault feature by the optimized Q-factor.
Then, due to the presence of interference in the decomposition result of ATQWT, the SK analysis of the transient impact component is used to determine the frequency band of periodic impact component characterizing fault feature by fast kurtogram.
Finally, the band-pass filter established through the above frequency band is employed to filter the interference in the transient impact component.
Simulation and experimental results indicate that the ATQWT can highlight the periodic impact component characterizing rolling bearing fault feature, and the SK can filter interference in the transient impact component, which improves feature extraction effect and has great significance to enhance fault diagnosis accuracy of the rolling bearing.
Compared with EEMD-TQWT and TQWT-SK, the fault feature extracted by the proposed method is prominent and effective.
American Psychological Association (APA)
Zhao, Jianlong& Zhang, Yongchao& Chen, Qingguang. 2020. Rolling Bearing Fault Feature Extraction Based on Adaptive Tunable Q-Factor Wavelet Transform and Spectral Kurtosis. Shock and Vibration،Vol. 2020, no. 2020, pp.1-19.
https://search.emarefa.net/detail/BIM-1213115
Modern Language Association (MLA)
Zhao, Jianlong…[et al.]. Rolling Bearing Fault Feature Extraction Based on Adaptive Tunable Q-Factor Wavelet Transform and Spectral Kurtosis. Shock and Vibration No. 2020 (2020), pp.1-19.
https://search.emarefa.net/detail/BIM-1213115
American Medical Association (AMA)
Zhao, Jianlong& Zhang, Yongchao& Chen, Qingguang. Rolling Bearing Fault Feature Extraction Based on Adaptive Tunable Q-Factor Wavelet Transform and Spectral Kurtosis. Shock and Vibration. 2020. Vol. 2020, no. 2020, pp.1-19.
https://search.emarefa.net/detail/BIM-1213115
Data Type
Journal Articles
Language
English
Notes
Includes bibliographical references
Record ID
BIM-1213115