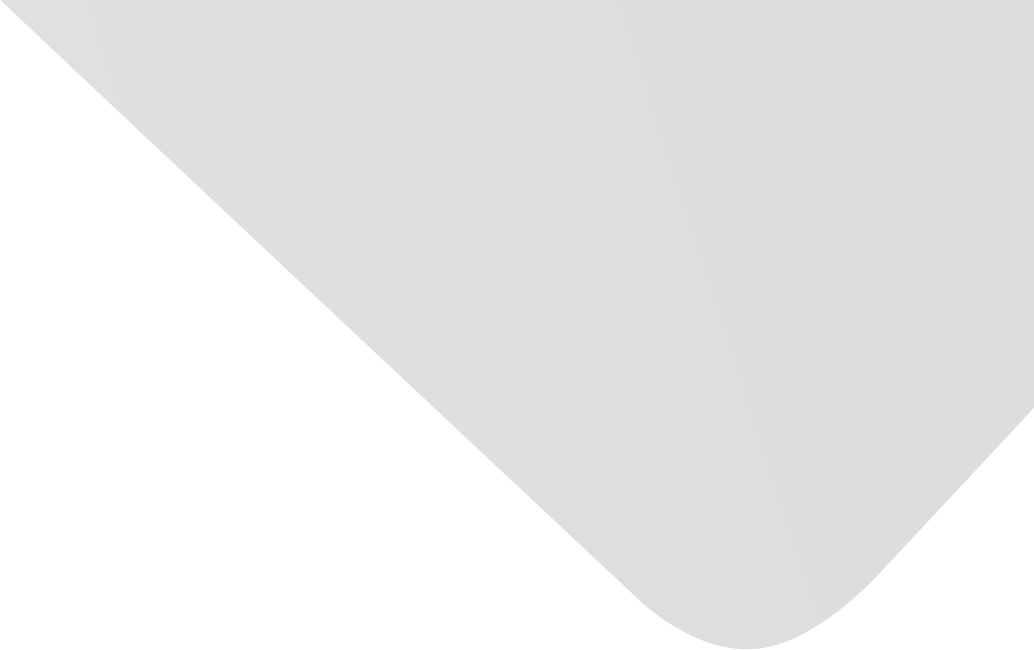
Identifying Capsule Defect Based on an Improved Convolutional Neural Network
Joint Authors
He, Jiao
Zhou, Junlin
Li, Guoli
Liu, Yongbin
Source
Issue
Vol. 2020, Issue 2020 (31 Dec. 2020), pp.1-9, 9 p.
Publisher
Hindawi Publishing Corporation
Publication Date
2020-07-11
Country of Publication
Egypt
No. of Pages
9
Main Subjects
Abstract EN
Capsules are commonly used as containers for most pharmaceuticals, and capsule quality is closely related to human health.
Given the actual demand for capsule production, this study proposes a capsule defect detection and recognition method based on an improved convolutional neural network (CNN) algorithm.
The algorithm is used for defect detection and classification in capsule production.
Defective and qualified capsule images in the actual production are collected as samples.
Then, a deep learning model based on the improved CNN is designed to train and test a capsule image dataset and identify defective capsules.
The improved CNN algorithm is based on regularization and the Adam optimizer (RACNN), on which a dropout layer and L2_regularization are added between the full connection and the output layer to solve the overfitting problem.
The Adam optimizer is introduced to accelerate model training and improve model convergence.
Then, cross entropy is used as a loss function to measure the prediction performance of the model.
By comparing the results of RACNN with different parameters, a detection method based on the optimal parameters of the RACNN model is finally selected.
Results show a 97.56% recognition accuracy of the proposed method.
Hence, this method could be used for the automatic identification and classification of defective capsules.
American Psychological Association (APA)
Zhou, Junlin& He, Jiao& Li, Guoli& Liu, Yongbin. 2020. Identifying Capsule Defect Based on an Improved Convolutional Neural Network. Shock and Vibration،Vol. 2020, no. 2020, pp.1-9.
https://search.emarefa.net/detail/BIM-1213174
Modern Language Association (MLA)
Zhou, Junlin…[et al.]. Identifying Capsule Defect Based on an Improved Convolutional Neural Network. Shock and Vibration No. 2020 (2020), pp.1-9.
https://search.emarefa.net/detail/BIM-1213174
American Medical Association (AMA)
Zhou, Junlin& He, Jiao& Li, Guoli& Liu, Yongbin. Identifying Capsule Defect Based on an Improved Convolutional Neural Network. Shock and Vibration. 2020. Vol. 2020, no. 2020, pp.1-9.
https://search.emarefa.net/detail/BIM-1213174
Data Type
Journal Articles
Language
English
Notes
Includes bibliographical references
Record ID
BIM-1213174