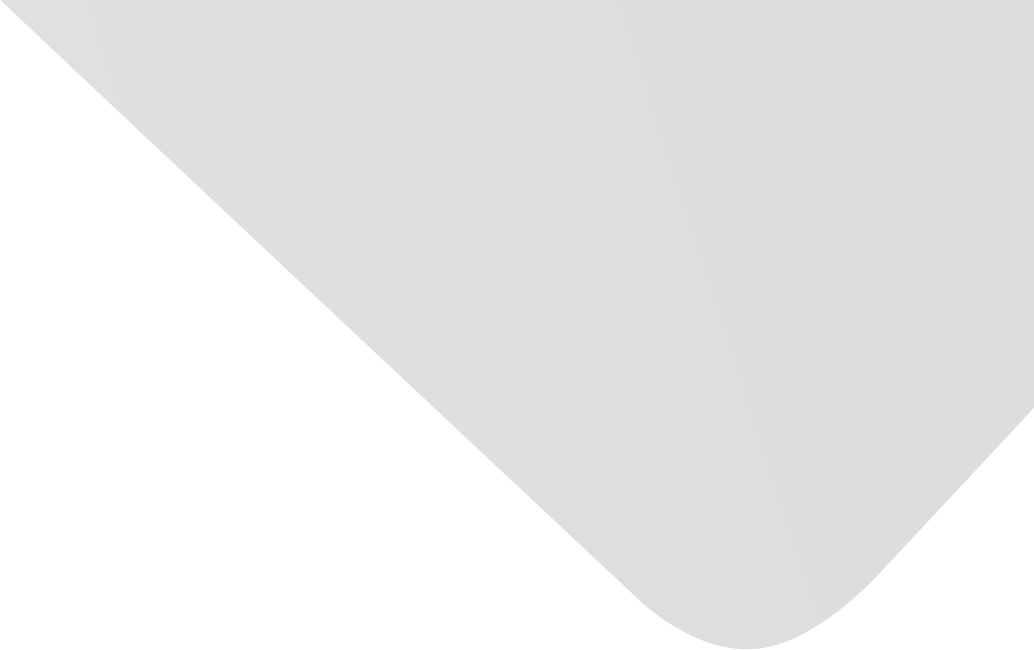
A Deep Multiscale Fusion Method via Low-Rank Sparse Decomposition for Object Saliency Detection Based on Urban Data in Optical Remote Sensing Images
Joint Authors
Source
Wireless Communications and Mobile Computing
Issue
Vol. 2020, Issue 2020 (31 Dec. 2020), pp.1-14, 14 p.
Publisher
Hindawi Publishing Corporation
Publication Date
2020-05-08
Country of Publication
Egypt
No. of Pages
14
Main Subjects
Information Technology and Computer Science
Abstract EN
The urban data provides a wealth of information that can support the life and work for people.
In this work, we research the object saliency detection in optical remote sensing images, which is conducive to the interpretation of urban scenes.
Saliency detection selects the regions with important information in the remote sensing images, which severely imitates the human visual system.
It plays a powerful role in other image processing.
It has successfully made great achievements in change detection, object tracking, temperature reversal, and other tasks.
The traditional method has some disadvantages such as poor robustness and high computational complexity.
Therefore, this paper proposes a deep multiscale fusion method via low-rank sparse decomposition for object saliency detection in optical remote sensing images.
First, we execute multiscale segmentation for remote sensing images.
Then, we calculate the saliency value, and the proposal region is generated.
The superpixel blocks of the remaining proposal regions of the segmentation map are input into the convolutional neural network.
By extracting the depth feature, the saliency value is calculated and the proposal regions are updated.
The feature transformation matrix is obtained based on the gradient descent method, and the high-level semantic prior knowledge is obtained by using the convolutional neural network.
The process is iterated continuously to obtain the saliency map at each scale.
The low-rank sparse decomposition of the transformed matrix is carried out by robust principal component analysis.
Finally, the weight cellular automata method is utilized to fuse the multiscale saliency graphs and the saliency map calculated according to the sparse noise obtained by decomposition.
Meanwhile, the object priors knowledge can filter most of the background information, reduce unnecessary depth feature extraction, and meaningfully improve the saliency detection rate.
The experiment results show that the proposed method can effectively improve the detection effect compared to other deep learning methods.
American Psychological Association (APA)
Zhang, Cheng& He, Dan. 2020. A Deep Multiscale Fusion Method via Low-Rank Sparse Decomposition for Object Saliency Detection Based on Urban Data in Optical Remote Sensing Images. Wireless Communications and Mobile Computing،Vol. 2020, no. 2020, pp.1-14.
https://search.emarefa.net/detail/BIM-1214517
Modern Language Association (MLA)
Zhang, Cheng& He, Dan. A Deep Multiscale Fusion Method via Low-Rank Sparse Decomposition for Object Saliency Detection Based on Urban Data in Optical Remote Sensing Images. Wireless Communications and Mobile Computing No. 2020 (2020), pp.1-14.
https://search.emarefa.net/detail/BIM-1214517
American Medical Association (AMA)
Zhang, Cheng& He, Dan. A Deep Multiscale Fusion Method via Low-Rank Sparse Decomposition for Object Saliency Detection Based on Urban Data in Optical Remote Sensing Images. Wireless Communications and Mobile Computing. 2020. Vol. 2020, no. 2020, pp.1-14.
https://search.emarefa.net/detail/BIM-1214517
Data Type
Journal Articles
Language
English
Notes
Includes bibliographical references
Record ID
BIM-1214517