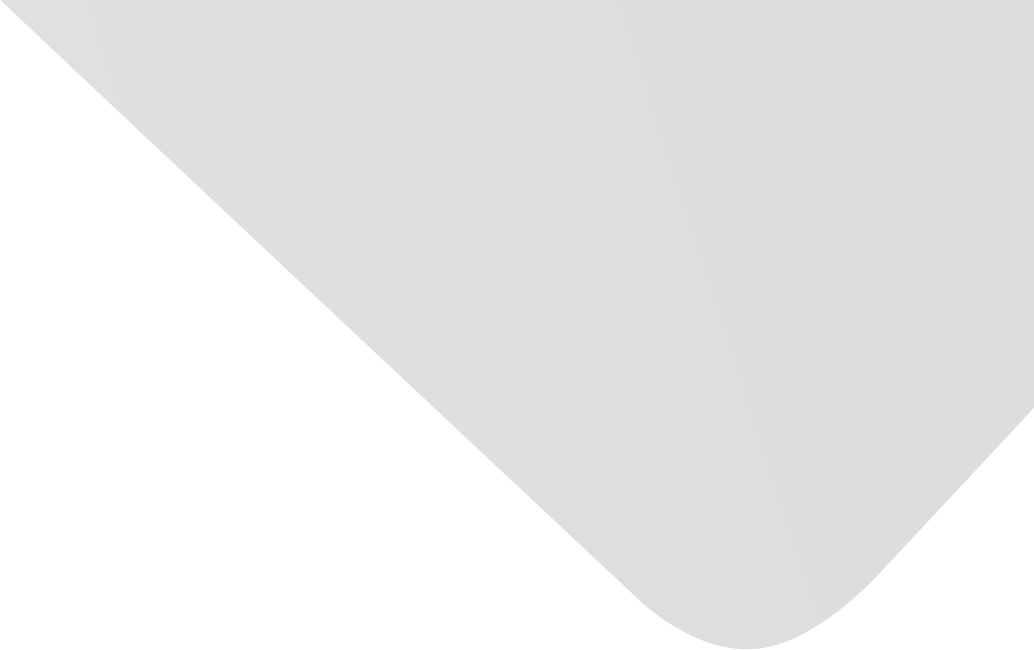
Image Annotation via Reconstitution Graph Learning Model
Joint Authors
Source
Wireless Communications and Mobile Computing
Issue
Vol. 2020, Issue 2020 (31 Dec. 2020), pp.1-9, 9 p.
Publisher
Hindawi Publishing Corporation
Publication Date
2020-12-14
Country of Publication
Egypt
No. of Pages
9
Main Subjects
Information Technology and Computer Science
Abstract EN
With great developments of computing technologies and data mining methods, image annotation has attracted much attraction in smart agriculture.
However, the semantic gap between labels and images poses great challenges on image annotation in agriculture, due to the label imbalance and difficulties in understanding obscure relationships of images and labels.
In this paper, an image annotation method based on graph learning is proposed to accurately annotate images.
Specifically, inspired by nearest neighbors, the semantic neighbor graph is introduced to generate preannotation, balancing unbalanced labels.
Then, the correlations between labels and images are modeled by the random dot product graph, to deeply mine semantics.
Finally, we perform experiments on two image sets.
The experimental results show that our method is much better than the previous method, which verifies the effectiveness of our model and the proposed method.
American Psychological Association (APA)
Chen, Shi& Wang, Meng& Chen, Xuan. 2020. Image Annotation via Reconstitution Graph Learning Model. Wireless Communications and Mobile Computing،Vol. 2020, no. 2020, pp.1-9.
https://search.emarefa.net/detail/BIM-1214587
Modern Language Association (MLA)
Chen, Shi…[et al.]. Image Annotation via Reconstitution Graph Learning Model. Wireless Communications and Mobile Computing No. 2020 (2020), pp.1-9.
https://search.emarefa.net/detail/BIM-1214587
American Medical Association (AMA)
Chen, Shi& Wang, Meng& Chen, Xuan. Image Annotation via Reconstitution Graph Learning Model. Wireless Communications and Mobile Computing. 2020. Vol. 2020, no. 2020, pp.1-9.
https://search.emarefa.net/detail/BIM-1214587
Data Type
Journal Articles
Language
English
Notes
Includes bibliographical references
Record ID
BIM-1214587