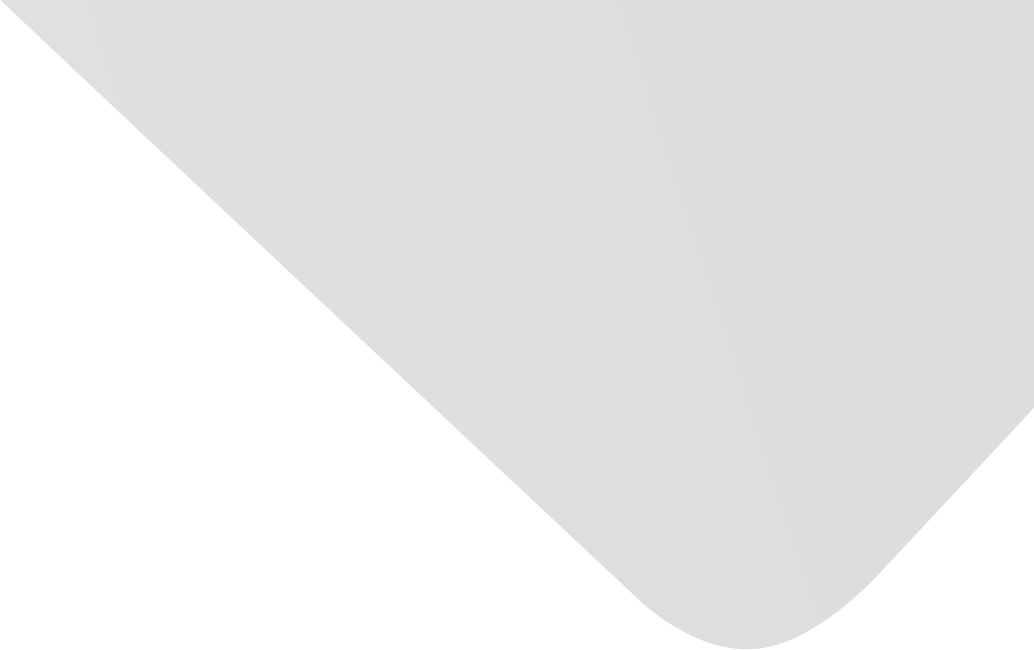
Railway Subgrade Defect Automatic Recognition Method Based on Improved Faster R-CNN
Joint Authors
Source
Issue
Vol. 2018, Issue 2018 (31 Dec. 2018), pp.1-12, 12 p.
Publisher
Hindawi Publishing Corporation
Publication Date
2018-06-14
Country of Publication
Egypt
No. of Pages
12
Main Subjects
Abstract EN
Railway subgrade defect is the serious threat to train safety.
Vehicle-borne GPR method has become the main railway subgrade detection technology with its advantages of rapidness and nondestructiveness.
However, due to the large amount of detection data and the variety in defect shape and size, defect recognition is a challenging task.
In this work, the method based on deep learning is proposed to recognize defects from the ground penetrating radar (GPR) profile of subgrade detection data.
Based on the Faster R-CNN framework, the improvement strategies of feature cascade, adversarial spatial dropout network (ASDN), Soft-NMS, and data augmentation have been integrated to improve recognition accuracy, according to the characteristics of subgrade defects.
The experimental results indicates that compared with traditional SVM+HOG method and the baseline Faster R-CNN, the improved model can achieve better performance.
The model robustness is demonstrated by a further comparison experiment of various defect types.
In addition, the improvements to model performance of each improvement strategy are verified by an ablation experiment of improvement strategies.
This paper tries to explore the new thinking for the application of deep learning method in the field of railway subgrade defect recognition.
American Psychological Association (APA)
Xu, Xinjun& Lei, Yang& Yang, Feng. 2018. Railway Subgrade Defect Automatic Recognition Method Based on Improved Faster R-CNN. Scientific Programming،Vol. 2018, no. 2018, pp.1-12.
https://search.emarefa.net/detail/BIM-1214705
Modern Language Association (MLA)
Xu, Xinjun…[et al.]. Railway Subgrade Defect Automatic Recognition Method Based on Improved Faster R-CNN. Scientific Programming No. 2018 (2018), pp.1-12.
https://search.emarefa.net/detail/BIM-1214705
American Medical Association (AMA)
Xu, Xinjun& Lei, Yang& Yang, Feng. Railway Subgrade Defect Automatic Recognition Method Based on Improved Faster R-CNN. Scientific Programming. 2018. Vol. 2018, no. 2018, pp.1-12.
https://search.emarefa.net/detail/BIM-1214705
Data Type
Journal Articles
Language
English
Notes
Includes bibliographical references
Record ID
BIM-1214705