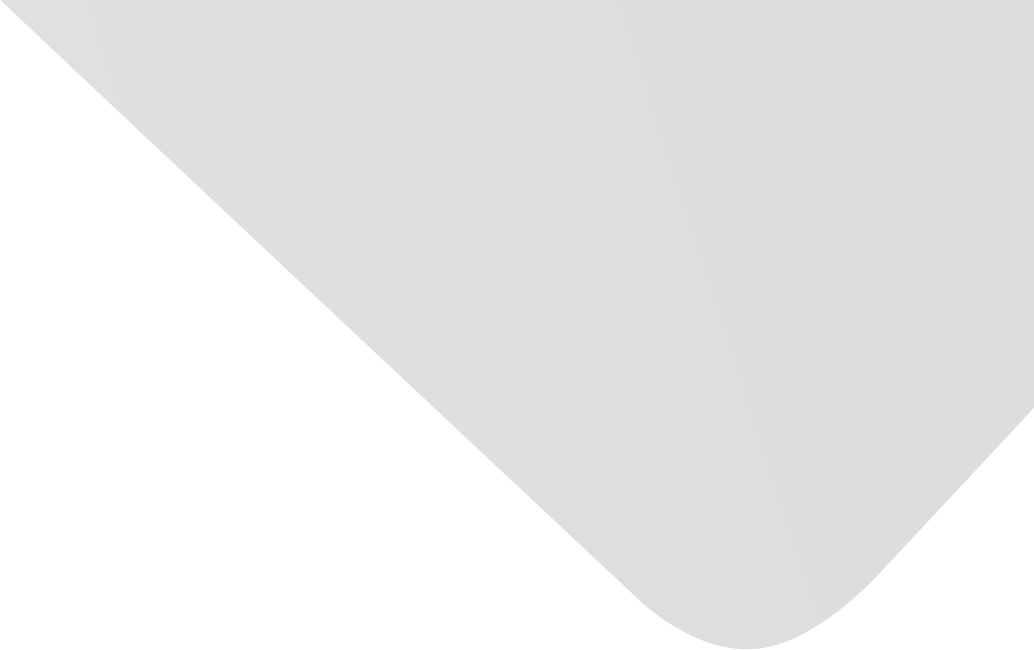
Air Pollution Concentration Forecast Method Based on the Deep Ensemble Neural Network
Joint Authors
Guo, Canyang
Liu, Genggeng
Chen, Chi-Hua
Source
Wireless Communications and Mobile Computing
Issue
Vol. 2020, Issue 2020 (31 Dec. 2020), pp.1-13, 13 p.
Publisher
Hindawi Publishing Corporation
Publication Date
2020-10-05
Country of Publication
Egypt
No. of Pages
13
Main Subjects
Information Technology and Computer Science
Abstract EN
The global environment has become more polluted due to the rapid development of industrial technology.
However, the existing machine learning prediction methods of air quality fail to analyze the reasons for the change of air pollution concentration because most of the prediction methods take more focus on the model selection.
Since the framework of recent deep learning is very flexible, the model may be deep and complex in order to fit the dataset.
Therefore, overfitting problems may exist in a single deep neural network model when the number of weights in the deep neural network model is large.
Besides, the learning rate of stochastic gradient descent (SGD) treats all parameters equally, resulting in local optimal solution.
In this paper, the Pearson correlation coefficient is used to analyze the inherent correlation of PM2.5 and other auxiliary data such as meteorological data, season data, and time stamp data which are applied to cluster for enhancing the performance.
Extracted features are helpful to build a deep ensemble network (EN) model which combines the recurrent neural network (RNN), long short-term memory (LSTM) network, and gated recurrent unit (GRU) network to predict the PM2.5 concentration of the next hour.
The weights of the submodel change with the accuracy of them in the validation set, so the ensemble has generalization ability.
The adaptive moment estimation (Adam) an algorithm for stochastic optimization is used to optimize the weights instead of SGD.
In order to compare the overall performance of different algorithms, the mean absolute error (MAE) and mean absolute percentage error (MAPE) are used as accuracy metrics in the experiments of this study.
The experiment results show that the proposed method achieves an accuracy rate (i.e., MAE=6.19 and MAPE=16.20%) and outperforms the comparative models.
American Psychological Association (APA)
Guo, Canyang& Liu, Genggeng& Chen, Chi-Hua. 2020. Air Pollution Concentration Forecast Method Based on the Deep Ensemble Neural Network. Wireless Communications and Mobile Computing،Vol. 2020, no. 2020, pp.1-13.
https://search.emarefa.net/detail/BIM-1214743
Modern Language Association (MLA)
Guo, Canyang…[et al.]. Air Pollution Concentration Forecast Method Based on the Deep Ensemble Neural Network. Wireless Communications and Mobile Computing No. 2020 (2020), pp.1-13.
https://search.emarefa.net/detail/BIM-1214743
American Medical Association (AMA)
Guo, Canyang& Liu, Genggeng& Chen, Chi-Hua. Air Pollution Concentration Forecast Method Based on the Deep Ensemble Neural Network. Wireless Communications and Mobile Computing. 2020. Vol. 2020, no. 2020, pp.1-13.
https://search.emarefa.net/detail/BIM-1214743
Data Type
Journal Articles
Language
English
Notes
Includes bibliographical references
Record ID
BIM-1214743