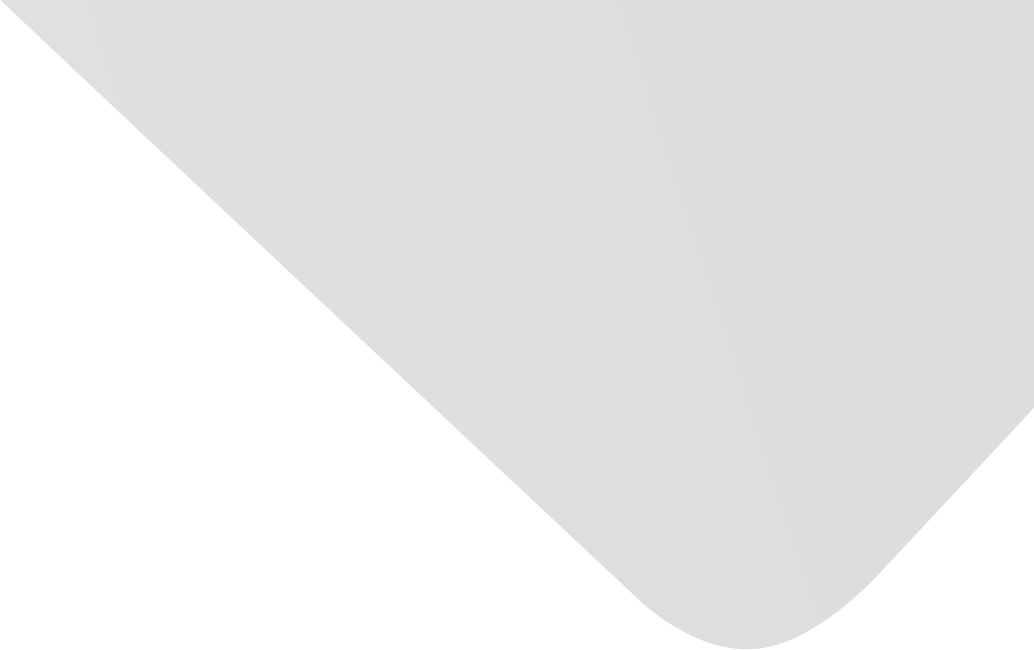
A Multichannel Biomedical Named Entity Recognition Model Based on Multitask Learning and Contextualized Word Representations
Joint Authors
Qu, Wen
Wei, Hao
Gao, Mingyuan
Zhou, Ai
Zhang, Yijia
Lu, Mingyu
Chen, Fei
Source
Wireless Communications and Mobile Computing
Issue
Vol. 2020, Issue 2020 (31 Dec. 2020), pp.1-13, 13 p.
Publisher
Hindawi Publishing Corporation
Publication Date
2020-08-10
Country of Publication
Egypt
No. of Pages
13
Main Subjects
Information Technology and Computer Science
Abstract EN
As the biomedical literature increases exponentially, biomedical named entity recognition (BNER) has become an important task in biomedical information extraction.
In the previous studies based on deep learning, pretrained word embedding becomes an indispensable part of the neural network models, effectively improving their performance.
However, the biomedical literature typically contains numerous polysemous and ambiguous words.
Using fixed pretrained word representations is not appropriate.
Therefore, this paper adopts the pretrained embeddings from language models (ELMo) to generate dynamic word embeddings according to context.
In addition, in order to avoid the problem of insufficient training data in specific fields and introduce richer input representations, we propose a multitask learning multichannel bidirectional gated recurrent unit (BiGRU) model.
Multiple feature representations (e.g., word-level, contextualized word-level, character-level) are, respectively, or collectively fed into the different channels.
Manual participation and feature engineering can be avoided through automatic capturing features in BiGRU.
In merge layer, multiple methods are designed to integrate the outputs of multichannel BiGRU.
We combine BiGRU with the conditional random field (CRF) to address labels’ dependence in sequence labeling.
Moreover, we introduce the auxiliary corpora with same entity types for the main corpora to be evaluated in multitask learning framework, then train our model on these separate corpora and share parameters with each other.
Our model obtains promising results on the JNLPBA and NCBI-disease corpora, with F1-scores of 76.0% and 88.7%, respectively.
The latter achieves the best performance among reported existing feature-based models.
American Psychological Association (APA)
Wei, Hao& Gao, Mingyuan& Zhou, Ai& Chen, Fei& Qu, Wen& Zhang, Yijia…[et al.]. 2020. A Multichannel Biomedical Named Entity Recognition Model Based on Multitask Learning and Contextualized Word Representations. Wireless Communications and Mobile Computing،Vol. 2020, no. 2020, pp.1-13.
https://search.emarefa.net/detail/BIM-1214925
Modern Language Association (MLA)
Wei, Hao…[et al.]. A Multichannel Biomedical Named Entity Recognition Model Based on Multitask Learning and Contextualized Word Representations. Wireless Communications and Mobile Computing No. 2020 (2020), pp.1-13.
https://search.emarefa.net/detail/BIM-1214925
American Medical Association (AMA)
Wei, Hao& Gao, Mingyuan& Zhou, Ai& Chen, Fei& Qu, Wen& Zhang, Yijia…[et al.]. A Multichannel Biomedical Named Entity Recognition Model Based on Multitask Learning and Contextualized Word Representations. Wireless Communications and Mobile Computing. 2020. Vol. 2020, no. 2020, pp.1-13.
https://search.emarefa.net/detail/BIM-1214925
Data Type
Journal Articles
Language
English
Notes
Includes bibliographical references
Record ID
BIM-1214925