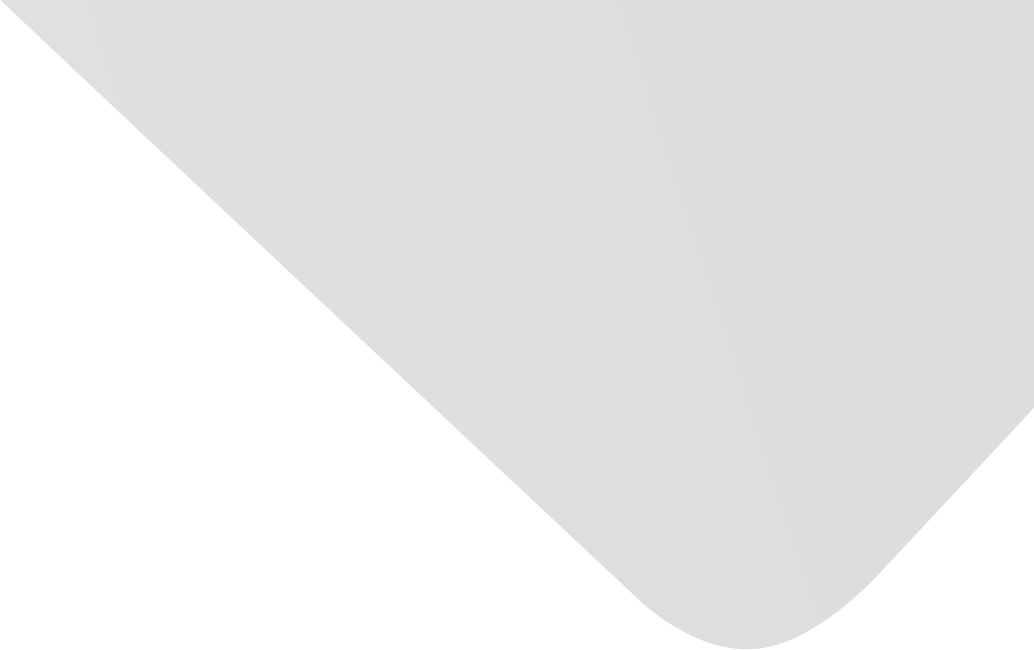
Leveraging Deep Learning Techniques for Malaria Parasite Detection Using Mobile Application
Joint Authors
Cheikhrouhou, Omar
Masud, Mehedi
Hossain, M. Shamim
Alhumyani, Hesham
Alshamrani, Sultan S.
Ibrahim, Saleh
Muhammad, Ghulam
Shorfuzzaman, Mohammad
Source
Wireless Communications and Mobile Computing
Issue
Vol. 2020, Issue 2020 (31 Dec. 2020), pp.1-15, 15 p.
Publisher
Hindawi Publishing Corporation
Publication Date
2020-07-08
Country of Publication
Egypt
No. of Pages
15
Main Subjects
Information Technology and Computer Science
Abstract EN
Malaria is a contagious disease that affects millions of lives every year.
Traditional diagnosis of malaria in laboratory requires an experienced person and careful inspection to discriminate healthy and infected red blood cells (RBCs).
It is also very time-consuming and may produce inaccurate reports due to human errors.
Cognitive computing and deep learning algorithms simulate human intelligence to make better human decisions in applications like sentiment analysis, speech recognition, face detection, disease detection, and prediction.
Due to the advancement of cognitive computing and machine learning techniques, they are now widely used to detect and predict early disease symptoms in healthcare field.
With the early prediction results, healthcare professionals can provide better decisions for patient diagnosis and treatment.
Machine learning algorithms also aid the humans to process huge and complex medical datasets and then analyze them into clinical insights.
This paper looks for leveraging deep learning algorithms for detecting a deadly disease, malaria, for mobile healthcare solution of patients building an effective mobile system.
The objective of this paper is to show how deep learning architecture such as convolutional neural network (CNN) which can be useful in real-time malaria detection effectively and accurately from input images and to reduce manual labor with a mobile application.
To this end, we evaluate the performance of a custom CNN model using a cyclical stochastic gradient descent (SGD) optimizer with an automatic learning rate finder and obtain an accuracy of 97.30% in classifying healthy and infected cell images with a high degree of precision and sensitivity.
This outcome of the paper will facilitate microscopy diagnosis of malaria to a mobile application so that reliability of the treatment and lack of medical expertise can be solved.
American Psychological Association (APA)
Masud, Mehedi& Alhumyani, Hesham& Alshamrani, Sultan S.& Cheikhrouhou, Omar& Ibrahim, Saleh& Muhammad, Ghulam…[et al.]. 2020. Leveraging Deep Learning Techniques for Malaria Parasite Detection Using Mobile Application. Wireless Communications and Mobile Computing،Vol. 2020, no. 2020, pp.1-15.
https://search.emarefa.net/detail/BIM-1214929
Modern Language Association (MLA)
Masud, Mehedi…[et al.]. Leveraging Deep Learning Techniques for Malaria Parasite Detection Using Mobile Application. Wireless Communications and Mobile Computing No. 2020 (2020), pp.1-15.
https://search.emarefa.net/detail/BIM-1214929
American Medical Association (AMA)
Masud, Mehedi& Alhumyani, Hesham& Alshamrani, Sultan S.& Cheikhrouhou, Omar& Ibrahim, Saleh& Muhammad, Ghulam…[et al.]. Leveraging Deep Learning Techniques for Malaria Parasite Detection Using Mobile Application. Wireless Communications and Mobile Computing. 2020. Vol. 2020, no. 2020, pp.1-15.
https://search.emarefa.net/detail/BIM-1214929
Data Type
Journal Articles
Language
English
Notes
Includes bibliographical references
Record ID
BIM-1214929