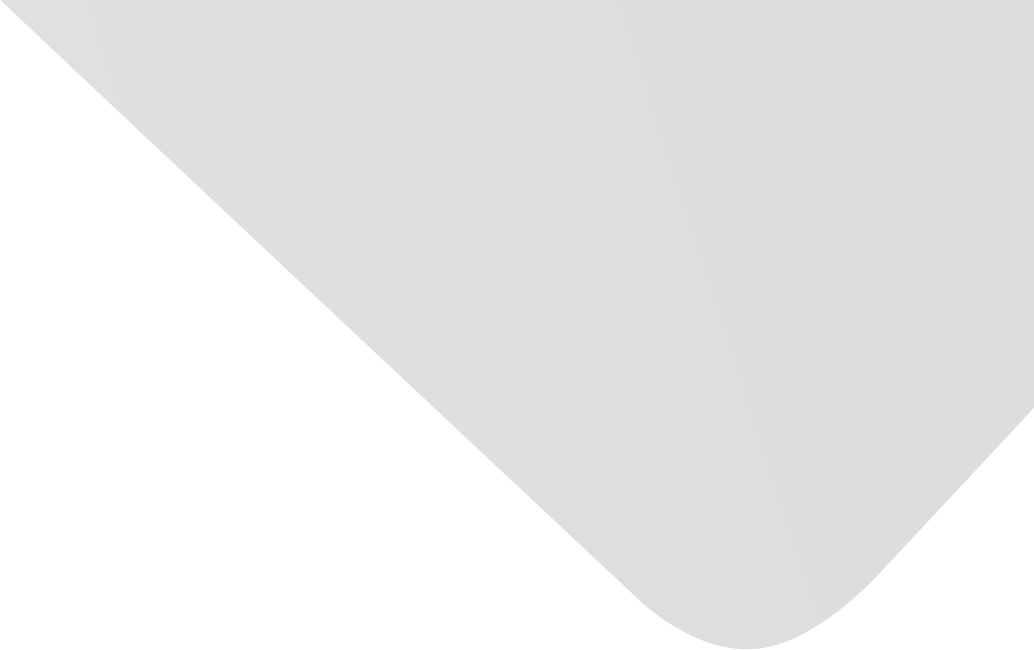
A Novel Faults Diagnosis Method for Rolling Element Bearings Based on ELCD and Extreme Learning Machine
Joint Authors
Liang, Mingliang
Su, Dongmin
Hu, Daidi
Ge, Mingtao
Source
Issue
Vol. 2018, Issue 2018 (31 Dec. 2018), pp.1-10, 10 p.
Publisher
Hindawi Publishing Corporation
Publication Date
2018-01-15
Country of Publication
Egypt
No. of Pages
10
Main Subjects
Abstract EN
A rolling bearing fault diagnosis method based on ensemble local characteristic-scale decomposition (ELCD) and extreme learning machine (ELM) is proposed.
Vibration signals were decomposed using ELCD, and numerous intrinsic scale components (ISCs) were obtained.
Next, time-domain index, energy, and relative entropy of intrinsic scale components were calculated.
According to the distance-based evaluation approach, sensitivity features can be extracted.
Finally, sensitivity features were input to extreme learning machine to identify rolling bearing fault types.
Experimental results show that the proposed method achieved better performance than support vector machine (SVM) and backpropagation (BP) neural network methods.
American Psychological Association (APA)
Liang, Mingliang& Su, Dongmin& Hu, Daidi& Ge, Mingtao. 2018. A Novel Faults Diagnosis Method for Rolling Element Bearings Based on ELCD and Extreme Learning Machine. Shock and Vibration،Vol. 2018, no. 2018, pp.1-10.
https://search.emarefa.net/detail/BIM-1215074
Modern Language Association (MLA)
Liang, Mingliang…[et al.]. A Novel Faults Diagnosis Method for Rolling Element Bearings Based on ELCD and Extreme Learning Machine. Shock and Vibration No. 2018 (2018), pp.1-10.
https://search.emarefa.net/detail/BIM-1215074
American Medical Association (AMA)
Liang, Mingliang& Su, Dongmin& Hu, Daidi& Ge, Mingtao. A Novel Faults Diagnosis Method for Rolling Element Bearings Based on ELCD and Extreme Learning Machine. Shock and Vibration. 2018. Vol. 2018, no. 2018, pp.1-10.
https://search.emarefa.net/detail/BIM-1215074
Data Type
Journal Articles
Language
English
Notes
Includes bibliographical references
Record ID
BIM-1215074