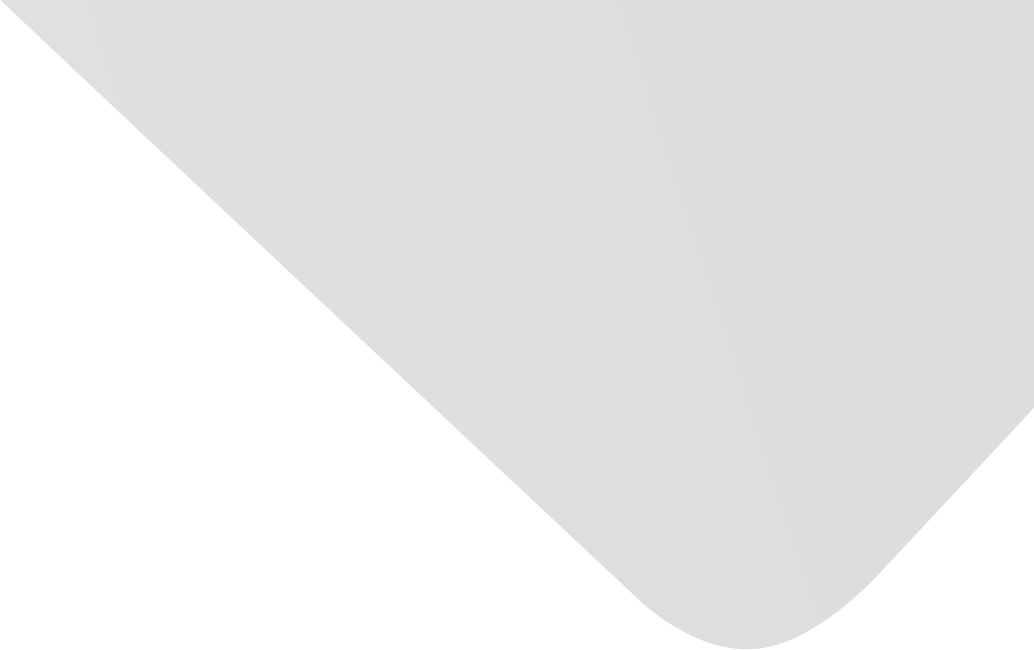
Reliable Fault Diagnosis of Rotary Machine Bearings Using a Stacked Sparse Autoencoder-Based Deep Neural Network
Joint Authors
Sohaib, Muhammad
Kim, Jong-Myon
Source
Issue
Vol. 2018, Issue 2018 (31 Dec. 2018), pp.1-11, 11 p.
Publisher
Hindawi Publishing Corporation
Publication Date
2018-05-07
Country of Publication
Egypt
No. of Pages
11
Main Subjects
Abstract EN
Due to enhanced safety, cost-effectiveness, and reliability requirements, fault diagnosis of bearings using vibration acceleration signals has been a key area of research over the past several decades.
Many fault diagnosis algorithms have been developed that can efficiently classify faults under constant speed conditions.
However, the performances of these traditional algorithms deteriorate with fluctuations of the shaft speed.
In the past couple of years, deep learning algorithms have not only improved the classification performance in various disciplines (e.g., in image processing and natural language processing), but also reduced the complexity of feature extraction and selection processes.
In this study, using complex envelope spectra and stacked sparse autoencoder- (SSAE-) based deep neural networks (DNNs), a fault diagnosis scheme is developed that can overcome fluctuations of the shaft speed.
The complex envelope spectrum made the frequency components associated with each fault type vibrant, hence helping the autoencoders to learn the characteristic features from the given input signals more readily.
Moreover, the implementation of SSAE-DNN for bearing fault diagnosis has avoided the need of handcrafted features that are used in traditional fault diagnosis schemes.
The experimental results demonstrate that the proposed scheme outperforms conventional fault diagnosis algorithms in terms of fault classification accuracy when tested with variable shaft speed data.
American Psychological Association (APA)
Sohaib, Muhammad& Kim, Jong-Myon. 2018. Reliable Fault Diagnosis of Rotary Machine Bearings Using a Stacked Sparse Autoencoder-Based Deep Neural Network. Shock and Vibration،Vol. 2018, no. 2018, pp.1-11.
https://search.emarefa.net/detail/BIM-1215150
Modern Language Association (MLA)
Sohaib, Muhammad& Kim, Jong-Myon. Reliable Fault Diagnosis of Rotary Machine Bearings Using a Stacked Sparse Autoencoder-Based Deep Neural Network. Shock and Vibration No. 2018 (2018), pp.1-11.
https://search.emarefa.net/detail/BIM-1215150
American Medical Association (AMA)
Sohaib, Muhammad& Kim, Jong-Myon. Reliable Fault Diagnosis of Rotary Machine Bearings Using a Stacked Sparse Autoencoder-Based Deep Neural Network. Shock and Vibration. 2018. Vol. 2018, no. 2018, pp.1-11.
https://search.emarefa.net/detail/BIM-1215150
Data Type
Journal Articles
Language
English
Notes
Includes bibliographical references
Record ID
BIM-1215150