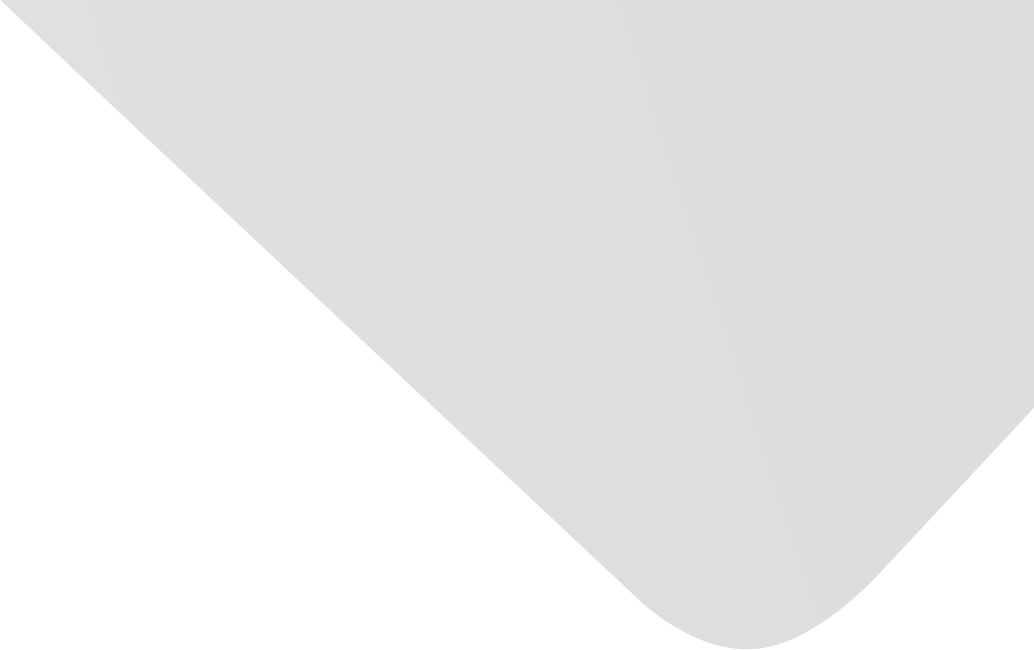
Rolling Bearing Fault Diagnosis Using Modified Neighborhood Preserving Embedding and Maximal Overlap Discrete Wavelet Packet Transform with Sensitive Features Selection
Joint Authors
Wu, Shoupeng
Ding, Enjie
Dong, Fei
Fan, Chunyang
Huang, Yanqiu
Cheng-hua, Huang
Source
Issue
Vol. 2018, Issue 2018 (31 Dec. 2018), pp.1-29, 29 p.
Publisher
Hindawi Publishing Corporation
Publication Date
2018-03-26
Country of Publication
Egypt
No. of Pages
29
Main Subjects
Abstract EN
In order to enhance the performance of bearing fault diagnosis and classification, features extraction and features dimensionality reduction have become more important.
The original statistical feature set was calculated from single branch reconstruction vibration signals obtained by using maximal overlap discrete wavelet packet transform (MODWPT).
In order to reduce redundancy information of original statistical feature set, features selection by adjusted rand index and sum of within-class mean deviations (FSASD) was proposed to select fault sensitive features.
Furthermore, a modified features dimensionality reduction method, supervised neighborhood preserving embedding with label information (SNPEL), was proposed to realize low-dimensional representations for high-dimensional feature space.
Finally, vibration signals collected from two experimental test rigs were employed to evaluate the performance of the proposed procedure.
The results show that the effectiveness, adaptability, and superiority of the proposed procedure can serve as an intelligent bearing fault diagnosis system.
American Psychological Association (APA)
Dong, Fei& Cheng-hua, Huang& Ding, Enjie& Wu, Shoupeng& Fan, Chunyang& Huang, Yanqiu. 2018. Rolling Bearing Fault Diagnosis Using Modified Neighborhood Preserving Embedding and Maximal Overlap Discrete Wavelet Packet Transform with Sensitive Features Selection. Shock and Vibration،Vol. 2018, no. 2018, pp.1-29.
https://search.emarefa.net/detail/BIM-1215297
Modern Language Association (MLA)
Dong, Fei…[et al.]. Rolling Bearing Fault Diagnosis Using Modified Neighborhood Preserving Embedding and Maximal Overlap Discrete Wavelet Packet Transform with Sensitive Features Selection. Shock and Vibration No. 2018 (2018), pp.1-29.
https://search.emarefa.net/detail/BIM-1215297
American Medical Association (AMA)
Dong, Fei& Cheng-hua, Huang& Ding, Enjie& Wu, Shoupeng& Fan, Chunyang& Huang, Yanqiu. Rolling Bearing Fault Diagnosis Using Modified Neighborhood Preserving Embedding and Maximal Overlap Discrete Wavelet Packet Transform with Sensitive Features Selection. Shock and Vibration. 2018. Vol. 2018, no. 2018, pp.1-29.
https://search.emarefa.net/detail/BIM-1215297
Data Type
Journal Articles
Language
English
Notes
Includes bibliographical references
Record ID
BIM-1215297