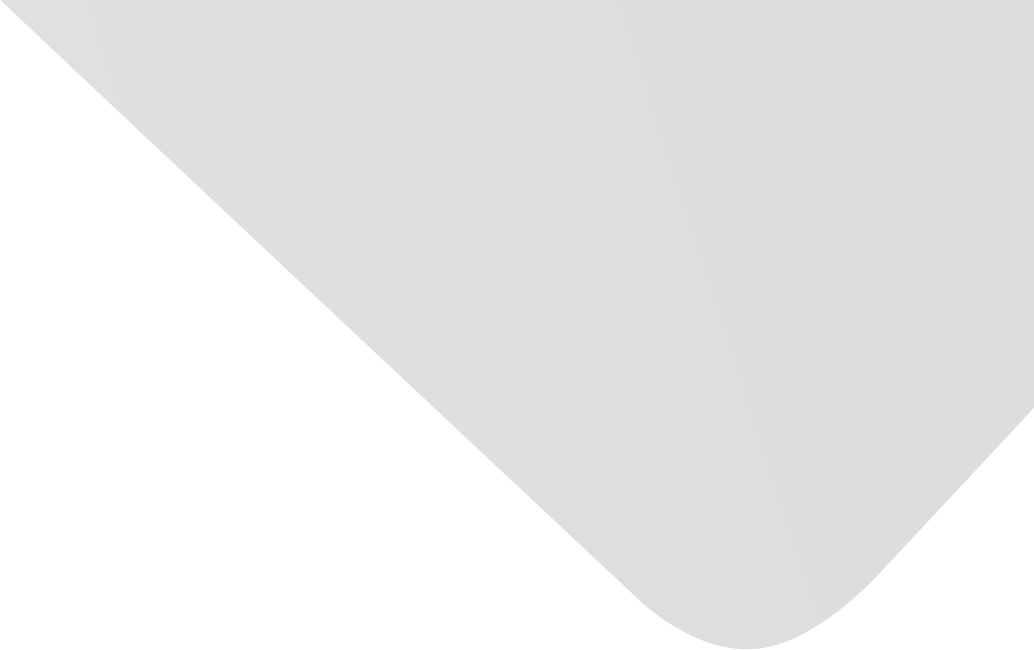
Predicting bank loan risks using machine learning algorithms
Other Title(s)
التنبؤ بمخاطر القروض المصرفية باستخدام خوارزميات تعلم الآلة
Joint Authors
al-Salim, Man Yunus Inad
Hassun, Safwan Umar
Source
al- Rafidain Journal of Computer Sciences and Mathematics
Issue
Vol. 14, Issue 1 (30 Jun. 2020), pp.149-158, 10 p.
Publisher
University of Mosul College of Computer Science and Mathematics
Publication Date
2020-06-30
Country of Publication
Iraq
No. of Pages
10
Main Subjects
Financial and Accounting Sciences
Topics
Abstract AR
تلعب القروض المصرفية دورا حاسما في تطوير الأعمال الاستثمارية للبنوك.
في الوقت الحاضر هناك العديد من القضايا المرتبطة بمخاطر القروض المصرفية.
مع ظهور أنظمة الحوسبة أصبحت البنوك قادرة على تسجيل بيانات المقترضين وفقا لمعاييرها.
في الواقع هناك كمية هائلة من بيانات المقترضين مما يجعل عملية اتخاذ القرار مهمة صعبة.
استخدمت العديد من الدراسات خوارزميات تنقيب البيانات لغرض تصنيف القروض من حيث الايفاء أو عدم الايفاء بسداد القرض بالاعتماد على بيانات المقترضين السابقة.
يمكن لهذا النوع من الخوارزميات مساعدة البنوك في اتخاذ قرارات المنح لعملائها.
في هذه الورقة تمت مقارنة أداء خوارزميات التعلم الآلي لغرض تصنيف مخاطر القروض المصرفية باستخدام المعايير القياسية ثم اختيار الشبكات العصبية متعددة الطبقات (Multilayer Perceptron) حيث أنها أعطت أفضل دقة مقارنة بخوارزميات RandomForest)، BayesNet، NaiveBayes & DTJ48).
Abstract EN
Bank loans play a crucial role in the development of banks investment business.
Nowadays, there are many risk-related issues associated with bank loans.
With the advent of computerization systems, banks have become able to register borrowers' data according their criteria.
In fact, there is a tremendous amount of borrowers' data, which makes the process of load management a challenging task.
Many studies have utilized data mining algorithms for the purpose of loans classification in terms of repayment or when the loans are not based on customers' financial history.
This kind of algorithms can help banks in making grant decisions for their customers.
In this paper, the performance of machine learning algorithms has been compared for the purpose of classifying bank loan risks using the standard criteria and then choosing (Multilayer Perceptron) as it has given best accuracy compared to RandomForest, BayesNet, NaiveBayes and DTJ48 algorithms.
American Psychological Association (APA)
al-Salim, Man Yunus Inad& Hassun, Safwan Umar. 2020. Predicting bank loan risks using machine learning algorithms. al- Rafidain Journal of Computer Sciences and Mathematics،Vol. 14, no. 1, pp.149-158.
https://search.emarefa.net/detail/BIM-1226308
Modern Language Association (MLA)
al-Salim, Man Yunus Inad& Hassun, Safwan Umar. Predicting bank loan risks using machine learning algorithms. al- Rafidain Journal of Computer Sciences and Mathematics Vol. 14, no. 1 (2020), pp.149-158.
https://search.emarefa.net/detail/BIM-1226308
American Medical Association (AMA)
al-Salim, Man Yunus Inad& Hassun, Safwan Umar. Predicting bank loan risks using machine learning algorithms. al- Rafidain Journal of Computer Sciences and Mathematics. 2020. Vol. 14, no. 1, pp.149-158.
https://search.emarefa.net/detail/BIM-1226308
Data Type
Journal Articles
Language
English
Notes
Includes bibliographical references : p. 158
Record ID
BIM-1226308