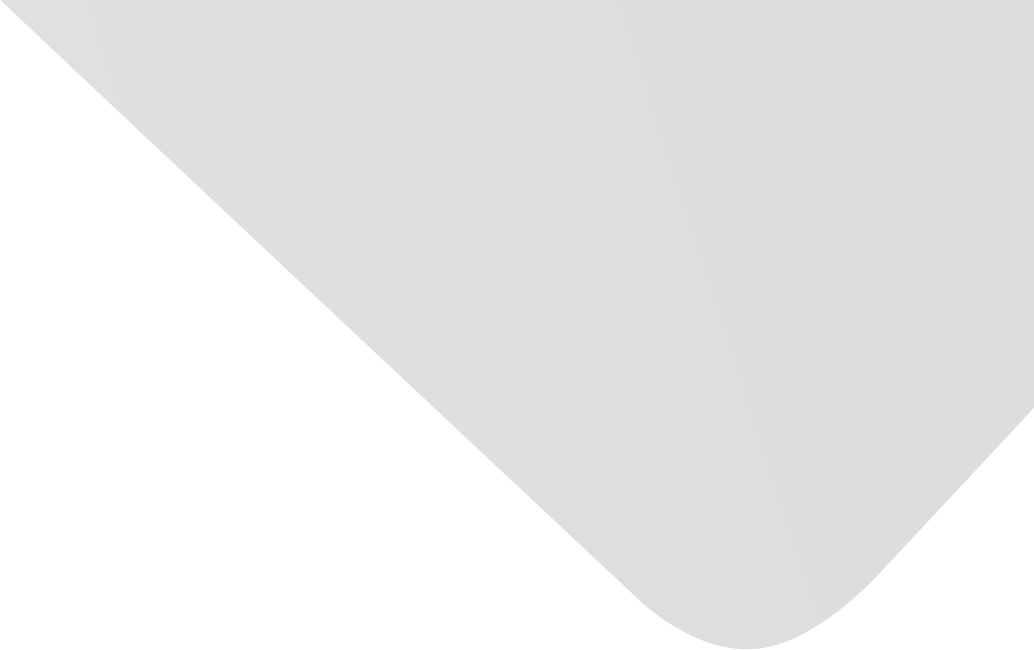
Hand gesture recognition of static letters American sign language (ASL) using deep learning
Joint Authors
Abd al-Husayn, Abd al-Wahab A.
Rahim, Firas Abd al-Razzaq
Source
Engineering and Technology Journal
Issue
Vol. 38, Issue 6A (30 Jun. 2020), pp.926-937, 12 p.
Publisher
Publication Date
2020-06-30
Country of Publication
Iraq
No. of Pages
12
Main Subjects
Topics
Abstract EN
An American Sign Language (ASL) is a complex language.
It is depending on the special gesture stander of marks.
These marks are represented by hands with assistance by facial expression and body posture.
ASL is the main communication language of deaf and people who have hard hearing from North America and other partsof the world.
In this paper, Gesture recognition is proposed of static ASL using Deep Learning.
The contribution consists of two solutions to the problem.
The first one is resizedwith Bicubic static ASL binary images.
Besides that, good recognition results in of detection the boundary hand using the Robert edge detection method.
The second solution is to classify the 24 alphabetsstatic characters of ASL using Convolution Neural Network (CNN) and Deep Learning.
The classification accuracy equals to 99.3 % and the error of loss function is 0.0002.
According to 36 minutes with 15 seconds of elapsed time result and 100 iterations.
The training is fast and gives the very good results, in comparison with other related works of CNN, SVM, and ANN for is a complex language.
It is depending on the special gesture stander of marks.
These marks are represented by hands with assistance by facial expression and body posture.
ASL is the main communication language of deaf and people who have hard hearing from North America and other partsof the world.
In this paper, Gesture recognition is proposed of static ASL using Deep Learning.
The contribution consists of two solutions to the problem.
The first one is resizedwith Bicubic static ASL binary images.
Besides that, good recognition results in of detection the boundary hand using the Robert edge detection method.
The second solution is to classify the 24 alphabetsstatic characters of ASL using Convolution Neural Network (CNN) and Deep Learning.
The classification accuracy equals to 99.3 % and the error of loss function is 0.0002.
According to 36 minutes with 15 seconds of elapsed time result and 100 iterations.
The training is fast and gives the very good results, in comparison with other related works of CNN, SVM, and ANN for training.
American Psychological Association (APA)
Abd al-Husayn, Abd al-Wahab A.& Rahim, Firas Abd al-Razzaq. 2020. Hand gesture recognition of static letters American sign language (ASL) using deep learning. Engineering and Technology Journal،Vol. 38, no. 6A, pp.926-937.
https://search.emarefa.net/detail/BIM-1236508
Modern Language Association (MLA)
Abd al-Husayn, Abd al-Wahab A.& Rahim, Firas Abd al-Razzaq. Hand gesture recognition of static letters American sign language (ASL) using deep learning. Engineering and Technology Journal Vol. 38, no. 6A (2020), pp.926-937.
https://search.emarefa.net/detail/BIM-1236508
American Medical Association (AMA)
Abd al-Husayn, Abd al-Wahab A.& Rahim, Firas Abd al-Razzaq. Hand gesture recognition of static letters American sign language (ASL) using deep learning. Engineering and Technology Journal. 2020. Vol. 38, no. 6A, pp.926-937.
https://search.emarefa.net/detail/BIM-1236508
Data Type
Journal Articles
Language
English
Notes
Includes bibliographical references : p. 936-937
Record ID
BIM-1236508