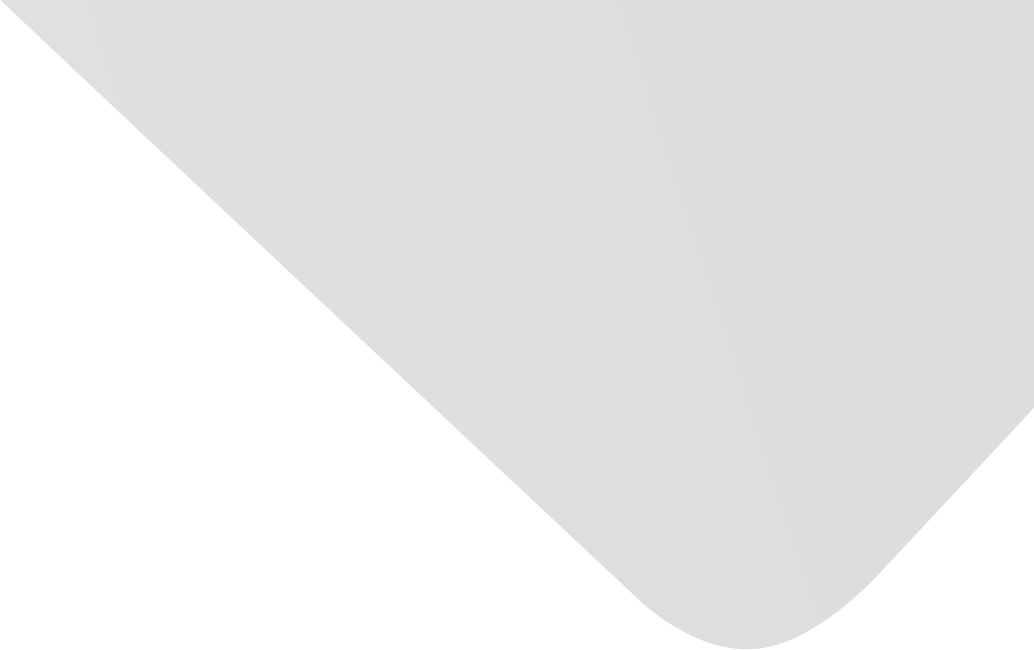
Intrusion detection system for NSL-KDD dataset based on deep learning and recursive feature elimination
Joint Authors
Muhammad, Bilal
Gbashi, Ekhlas K.
Source
Engineering and Technology Journal
Issue
Vol. 39, Issue 7 (31 Jul. 2021), pp.1069-1079, 11 p.
Publisher
Publication Date
2021-07-31
Country of Publication
Iraq
No. of Pages
11
Main Subjects
Information Technology and Computer Science
Topics
Abstract EN
Intrusion detection system is responsible for monitoring the systems and detect attacks, whether on (host or on a network) and identifying attacks that could come to the system and cause damage to them, that's mean an IDS prevents unauthorized access to systems by giving an alert to the administrator before causing any serious harm.
As a reasonable supplement of the firewall, intrusion detection technology can assist systems to deal with offensive, the Intrusions Detection Systems (IDSs) suffers from high false positive which leads to highly bad accuracy rate.
So this work is suggested to implement (IDS) by using a Recursive Feature Elimination to select features and use Deep Neural Network (DNN) and Recurrent Neural Network (RNN) for classification, the suggested model gives good results with high accuracy rate reaching 94% , DNN was used in the binary classification to classify either attack or Normal, while RNN was used in the classifications for the five classes (Normal, Dos, Probe, R2L, U2R).
The system was implemented by using (NSL-KDD) dataset, which was very efficient for offline analyses systems for Intrusion detection system is responsible for monitoring the systems and detect attacks, whether on (host or on a network) and identifying attacks that could come to the system and cause damage to them, that's mean an IDS prevents unauthorized access to systems by giving an alert to the administrator before causing any serious harm.
As a reasonable supplement of the firewall, intrusion detection technology can assist systems to deal with offensive, the Intrusions Detection Systems (IDSs) suffers from high false positive which leads to highly bad accuracy rate.
So this work is suggested to implement (IDS) by using a Recursive Feature Elimination to select features and use Deep Neural Network (DNN) and Recurrent Neural Network (RNN) for classification, the suggested model gives good results with high accuracy rate reaching 94% , DNN was used in the binary classification to classify either attack or Normal, while RNN was used in the classifications for the five classes (Normal, Dos, Probe, R2L, U2R).
The system was implemented by using (NSL-KDD) dataset, which was very efficient for offline analyses systems for IDS.
American Psychological Association (APA)
Muhammad, Bilal& Gbashi, Ekhlas K.. 2021. Intrusion detection system for NSL-KDD dataset based on deep learning and recursive feature elimination. Engineering and Technology Journal،Vol. 39, no. 7, pp.1069-1079.
https://search.emarefa.net/detail/BIM-1281535
Modern Language Association (MLA)
Muhammad, Bilal& Gbashi, Ekhlas K.. Intrusion detection system for NSL-KDD dataset based on deep learning and recursive feature elimination. Engineering and Technology Journal Vol. 39, no. 7 (2021), pp.1069-1079.
https://search.emarefa.net/detail/BIM-1281535
American Medical Association (AMA)
Muhammad, Bilal& Gbashi, Ekhlas K.. Intrusion detection system for NSL-KDD dataset based on deep learning and recursive feature elimination. Engineering and Technology Journal. 2021. Vol. 39, no. 7, pp.1069-1079.
https://search.emarefa.net/detail/BIM-1281535
Data Type
Journal Articles
Language
English
Notes
Includes bibliographical references : p. 1077-1079
Record ID
BIM-1281535