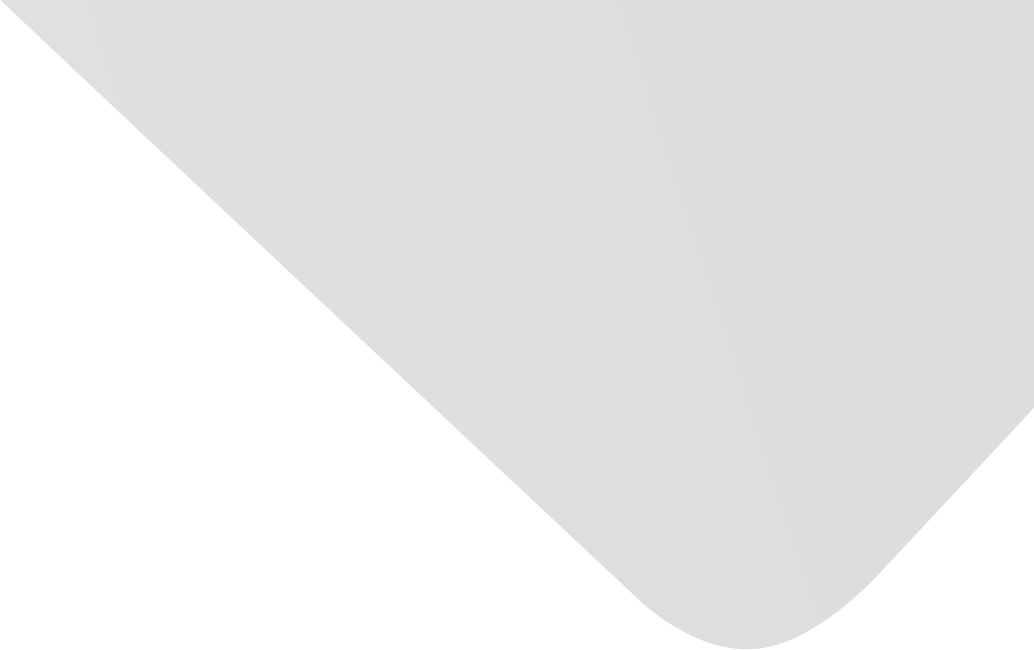
Embedded descriptor generation in faster R-CNN for multi-object tracking
Other Title(s)
إنشاء واصف متضمن داخل معمارية Faster R-Cnn من أجل تتبع كائنات متعددة
Joint Authors
Yunus, Yunus A.
al-Sayf, Khalil Ibrahim
Source
al- Rafidain Journal of Computer Sciences and Mathematics
Issue
Vol. 15, Issue 2 (31 Dec. 2021), pp.91-102, 12 p.
Publisher
University of Mosul College of Computer Science and Mathematics
Publication Date
2021-12-31
Country of Publication
Iraq
No. of Pages
12
Main Subjects
Information Technology and Computer Science
Topics
Abstract EN
With the rapid growth of computer usage to extract the required knowledge from a huge amount of information, such as a video file, significant attention has been brought towards multi-object detection and tracking.
Artificial Neural Networks (ANNs) have shown outstanding performance in multi-object detection, especially the Faster R-CNN network.
In this study, a new method is proposed for multi-object tracking based on descriptors generated by a neural network that is embedded in the Faster R-CNN.
This embedding allows the proposed method to directly output a descriptor for each object detected by the Faster R-CNN, based on the features detected by the Faster R-CNN to detect the object.
The use of these features allows the proposed method to output accurate values rapidly, as these features are already computed for the detection and have been able to provide outstanding performance in the detection stage.
The descriptors that are collected from the proposed method are then clustered into a number of clusters equal to the number of objects detected in the first frame of the video.
Then, for further frames, the number of clusters is increased until the distance between the centroid of the newly created cluster and the nearest centroid is less than the average distance among the centroids.
Newly added clusters are considered for new objects, whereas older ones are kept in case the object reappears in the video.
The proposed method is evaluated using the UA-DETRAC (University at Albany Detection and Tracking) dataset and has been able to achieve 64.8% MOTA and 83.6% MOTP, with a processing speed of 127.3 frames per second.
American Psychological Association (APA)
Yunus, Yunus A.& al-Sayf, Khalil Ibrahim. 2021. Embedded descriptor generation in faster R-CNN for multi-object tracking. al- Rafidain Journal of Computer Sciences and Mathematics،Vol. 15, no. 2, pp.91-102.
https://search.emarefa.net/detail/BIM-1298633
Modern Language Association (MLA)
Yunus, Yunus A.& al-Sayf, Khalil Ibrahim. Embedded descriptor generation in faster R-CNN for multi-object tracking. al- Rafidain Journal of Computer Sciences and Mathematics Vol. 15, no. 2 (2021), pp.91-102.
https://search.emarefa.net/detail/BIM-1298633
American Medical Association (AMA)
Yunus, Yunus A.& al-Sayf, Khalil Ibrahim. Embedded descriptor generation in faster R-CNN for multi-object tracking. al- Rafidain Journal of Computer Sciences and Mathematics. 2021. Vol. 15, no. 2, pp.91-102.
https://search.emarefa.net/detail/BIM-1298633
Data Type
Journal Articles
Language
English
Notes
Includes bibliographical references : p. 101-102
Record ID
BIM-1298633