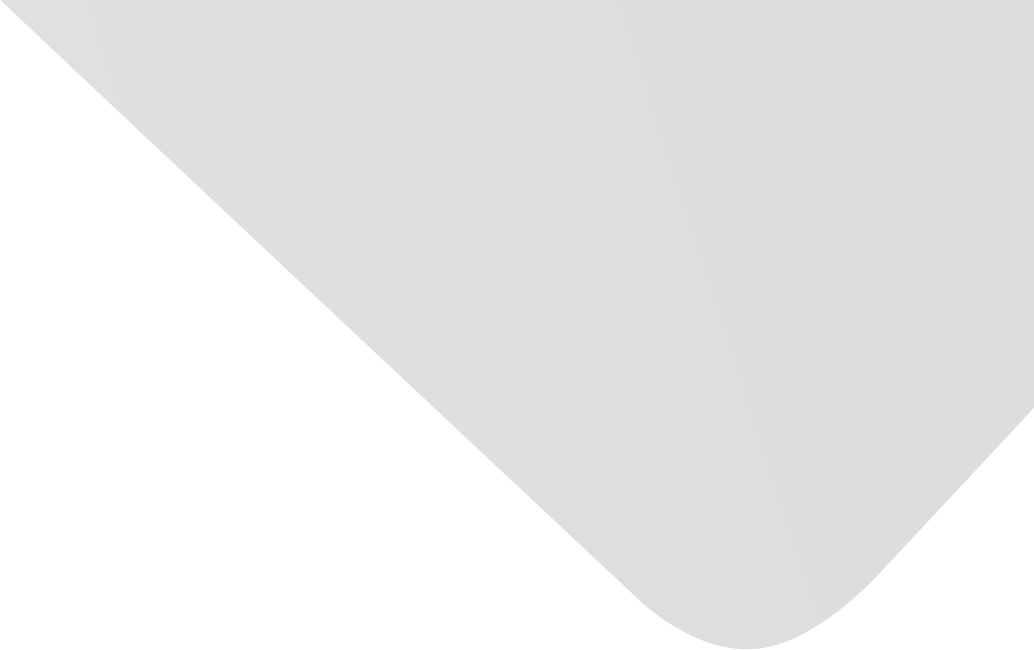
Uncovering the effects of data variation on protein sequence classification using deep learning
Joint Authors
Ismail, Rasha
Afifi, Yasamin M.
Badr, Najwa L.
Mustafa, Faridah A.
Source
International Journal of Intelligent Computing and Information Sciences
Issue
Vol. 22, Issue 2 (31 May. 2022), pp.112-125, 14 p.
Publisher
Ain Shams University Faculty of Computer and Information Sciences
Publication Date
2022-05-31
Country of Publication
Egypt
No. of Pages
14
Main Subjects
Information Technology and Computer Science
Topics
Abstract EN
Bioinformaticians face an issue in analyzing and studying protein similarity as the number of proteins grows.
protein sequence analysis helps in the prediction of protein functions.
it is critical for the analysis process to be able to appropriately categorize proteins based on their sequences.
the extraction of features from protein sequences is done using a variety of methods.
the goal of this study is to investigate the different variations of data on the classification performance of a deep learning model employing 3D data.
first, few research questions were formulated regarding the impact of the following criteria : dataset size, IMF importance, feature size, and preprocessing on the proposed deep learning classification process.
second, comprehensive experiments were conducted to answer the research questions.
Six feature extraction methods were utilized to create 3D features with two sizes (7 x 7 x 7 and 9 x 9 x 9), which were then fed into a convolutional neural network.
three datasets different in their sorts, sizes, and balance state were used.
accuracy, precision, recall and F1-score are the standard assessment metrics used.
experimental results draw significant conclusions.
first, the 7 x 7 x 7 feature matrix has a positive correlation between its dimensions, which improved the results.
second, using the sum of the first three IMF components had better impact than using the first IMF component.
third, the classification process did not benefit from the normalization of features for small datasets unlike the large dataset.
finally, the dataset size had a significant impact on training the CNN model, with a training accuracy reaching 84.03%.
American Psychological Association (APA)
Mustafa, Faridah A.& Afifi, Yasamin M.& Ismail, Rasha& Badr, Najwa L.. 2022. Uncovering the effects of data variation on protein sequence classification using deep learning. International Journal of Intelligent Computing and Information Sciences،Vol. 22, no. 2, pp.112-125.
https://search.emarefa.net/detail/BIM-1373834
Modern Language Association (MLA)
Mustafa, Faridah A.…[et al.]. Uncovering the effects of data variation on protein sequence classification using deep learning. International Journal of Intelligent Computing and Information Sciences Vol. 22, no. 2 (May. 2022), pp.112-125.
https://search.emarefa.net/detail/BIM-1373834
American Medical Association (AMA)
Mustafa, Faridah A.& Afifi, Yasamin M.& Ismail, Rasha& Badr, Najwa L.. Uncovering the effects of data variation on protein sequence classification using deep learning. International Journal of Intelligent Computing and Information Sciences. 2022. Vol. 22, no. 2, pp.112-125.
https://search.emarefa.net/detail/BIM-1373834
Data Type
Journal Articles
Language
English
Notes
Includes bibliographical references : p. 124-125
Record ID
BIM-1373834