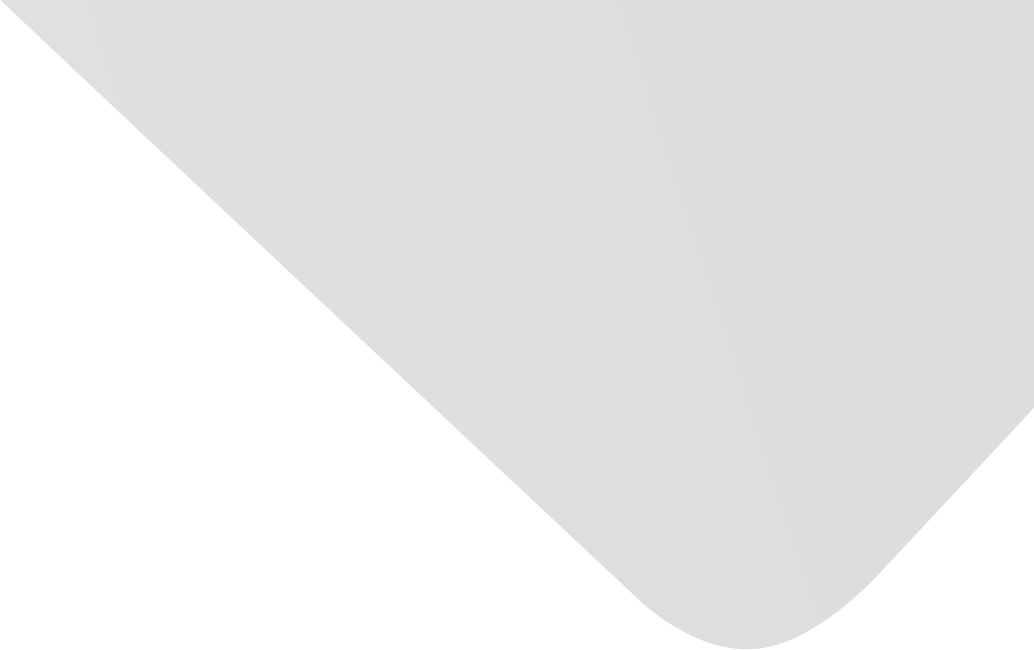
A comparison of COIVD-19 cases classification based on machine learning approaches
Joint Authors
Thalij, Saadi Hamad
Atiyyah, Uqbah Salim
Source
The Iraqi Journal of Electrical and Electronic Engineering
Issue
Vol. 18, Issue 1 (30 Jun. 2022), pp.139-143, 5 p.
Publisher
University of Basrah College of Engineering
Publication Date
2022-06-30
Country of Publication
Iraq
No. of Pages
5
Main Subjects
Information Technology and Computer Science
Topics
Abstract EN
COVID-19 emerged in 2019 in china, the worldwide spread rapidly, and caused many injuries and deaths among humans.
accurate and early detection of COVID-19 can ensure the long-term survival of patients and help prohibit the spread of the epidemic.
COVID-19 case classification techniques help health organizations quickly identify and treat severe cases.
algorithms of classification are one the essential matters for forecasting and making decisions to assist the diagnosis, early identification of COVID-19, and specify cases that require to intensive care unit to deliver the treatment at appropriate timing.
this paper is intended to compare algorithms of classification of machine learning to diagnose COVID-19 cases and measure their performance with many metrics, and measure mislabeling (false-positive and false-negative) to specify the best algorithms for speed and accuracy diagnosis.
in this paper, we focus onto classify the cases of covid-19 using the algorithms of machine learning.
we load the dataset and perform dataset preparation, pre-processing, analysis of data, selection of features, split of data, and use of classification algorithm.
in the first using four classification algorithms, (stochastic gradient descent, logistic regression, random forest, naive bayes), the outcome of algorithms accuracy respectively was 99.61%, 94.82% ,98.37%,96.57%, and the result of execution time for algorithms respectively were 0.01s, 0.7s, 0.20s, 0.04.
the stochastic gradient descent of mislabeling was better.
second, using four classification algorithms, (extreme-gradient boosting, decision tree, support vector machines, k_nearest neighbors), the outcome of algorithms accuracy was 98.37%, 99%, 97%, 88.4%, and the result of execution time for algorithms respectively were 0.18s, 0.02s, 0.3s, 0.01s.
the decision tree of mislabeling was better.
using machine learning helps improve allocate medical resources to maximize their utilization.
classification algorithm of clinical data for confirmed COVID-19 cases can help predict a patient's need to advance to the ICU or not need by using a global dataset of COVID-19 cases due to its accuracy and quality.
American Psychological Association (APA)
Atiyyah, Uqbah Salim& Thalij, Saadi Hamad. 2022. A comparison of COIVD-19 cases classification based on machine learning approaches. The Iraqi Journal of Electrical and Electronic Engineering،Vol. 18, no. 1, pp.139-143.
https://search.emarefa.net/detail/BIM-1380218
Modern Language Association (MLA)
Atiyyah, Uqbah Salim& Thalij, Saadi Hamad. A comparison of COIVD-19 cases classification based on machine learning approaches. The Iraqi Journal of Electrical and Electronic Engineering Vol. 18, no. 1 (Jun. 2022), pp.139-143.
https://search.emarefa.net/detail/BIM-1380218
American Medical Association (AMA)
Atiyyah, Uqbah Salim& Thalij, Saadi Hamad. A comparison of COIVD-19 cases classification based on machine learning approaches. The Iraqi Journal of Electrical and Electronic Engineering. 2022. Vol. 18, no. 1, pp.139-143.
https://search.emarefa.net/detail/BIM-1380218
Data Type
Journal Articles
Language
English
Notes
Includes bibliographical references : p. 143
Record ID
BIM-1380218