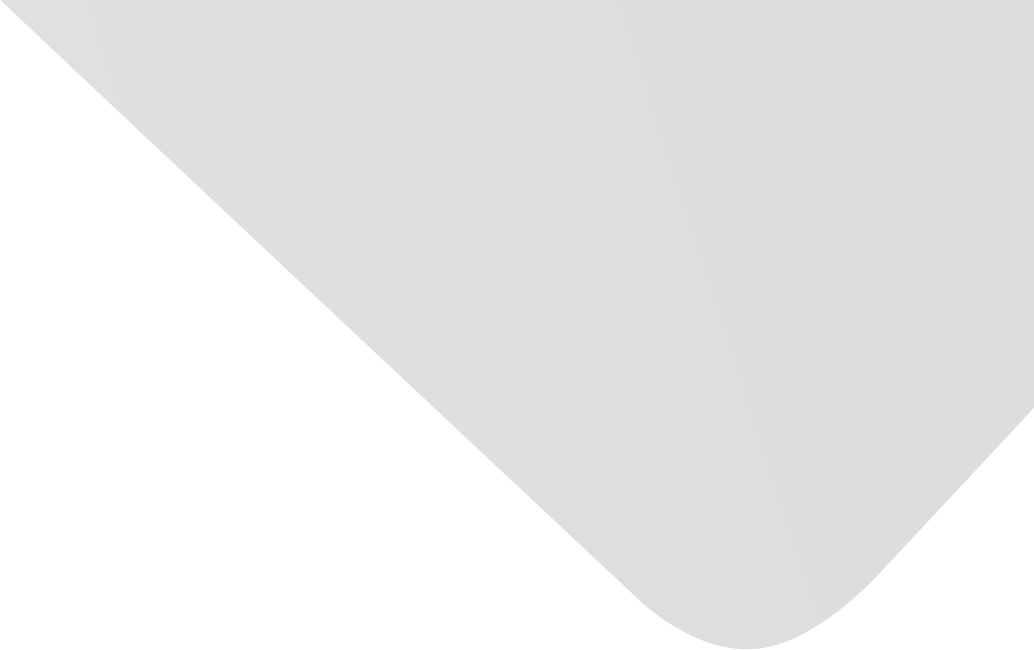
Feature pruning method for hidden Markova model-based anomaly detection : a comparison of performance
Joint Authors
Zuhdi, Muhammad
al-Haydari, Sulayman
Source
Jordanian Journal of Computetrs and Information Technology
Issue
Vol. 4, Issue 3 (31 Dec. 2018), pp.175-184, 10 p.
Publisher
Princess Sumaya University for Technology
Publication Date
2018-12-31
Country of Publication
Jordan
No. of Pages
10
Main Subjects
Information Technology and Computer Science
Abstract EN
Selecting effective and significant features for Hidden Markov Model (HMM) is very important for detecting anomalies in databases.
the goal of this research is to identify the most salient and important features in building HMM.
in order to improve the performance of hmm, an approach of feature pruning is proposed.
this approach is effective in detecting and classifying anomalies, very simple and easily implemented.
also, it is able to reduce computational complexity and time without compromising the model accuracy.
in this work, the proposed approach is applied to NSL-KDD (the new version of KDD Cup 99), DDoS, IoTPOT and UNSW_NB15 data sets.
those data sets are used to perform a comparative study that involves full feature set and a subset of significant features.
the experimental results show better performance in terms of efficiency and providing higher accuracy and lower false positive rate with reduced number of features, as well as eliminating irrelevant redundant or noisy features.
American Psychological Association (APA)
al-Haydari, Sulayman& Zuhdi, Muhammad. 2018. Feature pruning method for hidden Markova model-based anomaly detection : a comparison of performance. Jordanian Journal of Computetrs and Information Technology،Vol. 4, no. 3, pp.175-184.
https://search.emarefa.net/detail/BIM-1415328
Modern Language Association (MLA)
al-Haydari, Sulayman& Zuhdi, Muhammad. Feature pruning method for hidden Markova model-based anomaly detection : a comparison of performance. Jordanian Journal of Computetrs and Information Technology Vol. 4, no. 3 (Dec. 2018), pp.175-184.
https://search.emarefa.net/detail/BIM-1415328
American Medical Association (AMA)
al-Haydari, Sulayman& Zuhdi, Muhammad. Feature pruning method for hidden Markova model-based anomaly detection : a comparison of performance. Jordanian Journal of Computetrs and Information Technology. 2018. Vol. 4, no. 3, pp.175-184.
https://search.emarefa.net/detail/BIM-1415328
Data Type
Journal Articles
Language
English
Notes
Includes bibliographical references : p. 184
Record ID
BIM-1415328