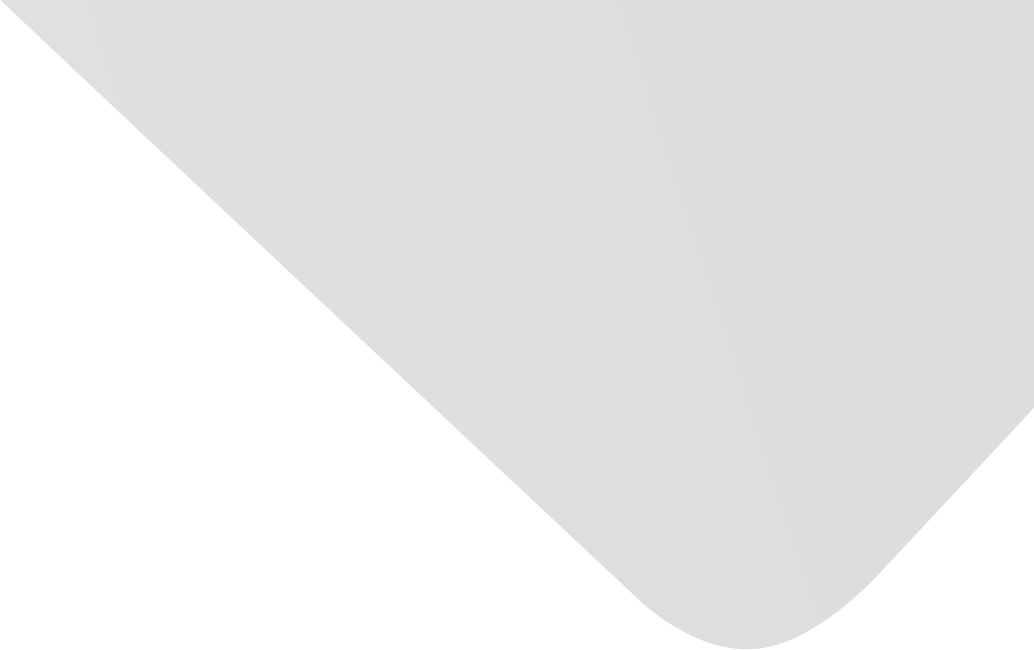
Performance evaluation of supervised machine learning classifiers for mapping natural language text to entity relationship models
Other Title(s)
تقييم أداء مصنفات تعلم الآلة الخاضعة للإشراف عند اشتقاق الكينونات الأزمة لبناء مخطط الكينونة العلاقة من نصوص اللغات الطبيعي
Author
Source
Journal of Pure and Applied Sciences
Issue
Vol. 20, Issue 1 (31 Mar. 2021), pp.6-10, 5 p.
Publisher
Publication Date
2021-03-31
Country of Publication
Libya
No. of Pages
5
Main Subjects
Information Technology and Computer Science
Abstract EN
Transforming natural language requirements into entities involves a thorough study of natural language text.
Sometimes mistakes are made by designers when manually performing this transformation.
Often, the process is time-consuming and inaccurate.
Hence, multiple research studies have been performed to assist inexperienced designers in mapping a natural language text into entities and reducing the time and error that such a method entails.
This work is part of those studies.
Human intervention is a significant constraint for prior studies.
In this paper, machine learning classifiers are used to eliminate human intervention.
The system performs well in predicting entities and has achieved 85%, 75% and 80% for recall, precision and the F-score, respectively.
The system also performs well in predicting nouns which do not represent entities and has achieved 68%, 79% and 76% for recall, precision and the F-score, respectively.
The performance level of the system is the same as other model generation tools found in the literature.
The system is distinguished from these tools in using machine learning classifiers as a technique for establishing entities with no human intervention.
Furthermore, the study finds that when distinguishing entities from other nouns, logic-based classifiers, perceptron-based classifiers and SVM classifiers perform better than statistical learning classifiers.
The decision tree classifier, neural network classifier and SVM classifier all work well.
The decision tree is the better because it can provide a decision tree that defines when a noun is an entity and when it is not based on given features; this is not the case with the neural network classifier and SVM classifier.
American Psychological Association (APA)
Umar, Musa Ahmad Muhammad. 2021. Performance evaluation of supervised machine learning classifiers for mapping natural language text to entity relationship models. Journal of Pure and Applied Sciences،Vol. 20, no. 1, pp.6-10.
https://search.emarefa.net/detail/BIM-1419213
Modern Language Association (MLA)
Umar, Musa Ahmad Muhammad. Performance evaluation of supervised machine learning classifiers for mapping natural language text to entity relationship models. Journal of Pure and Applied Sciences Vol. 20, no. 1 (Mar. 2021), pp.6-10.
https://search.emarefa.net/detail/BIM-1419213
American Medical Association (AMA)
Umar, Musa Ahmad Muhammad. Performance evaluation of supervised machine learning classifiers for mapping natural language text to entity relationship models. Journal of Pure and Applied Sciences. 2021. Vol. 20, no. 1, pp.6-10.
https://search.emarefa.net/detail/BIM-1419213
Data Type
Journal Articles
Language
English
Notes
Record ID
BIM-1419213