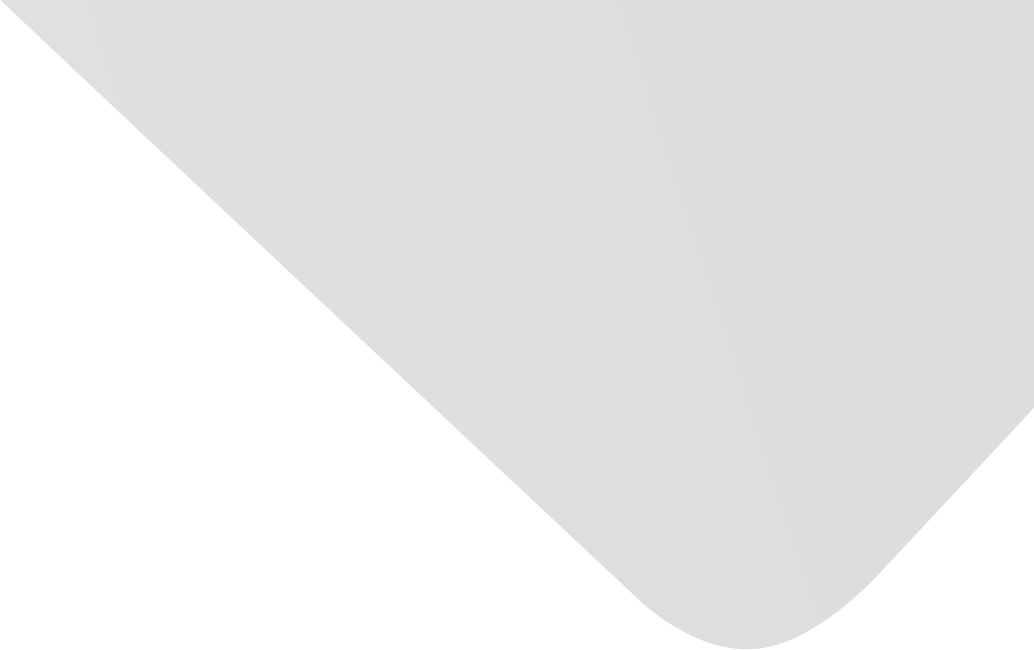
Stacknet based decision fusion classifier for network intrusion detection
Joint Authors
Nti, Isaac Kofi
Narko Boateng, Owusu
Adekoya, Adebayo Felix
Somanathan, Arjun Remadevi
Source
The International Arab Journal of Information Technology
Issue
Vol. 19, Issue 3A (s) (31 May. 2022), pp.478-490, 13 p.
Publisher
Zarqa University Deanship of Scientific Research
Publication Date
2022-05-31
Country of Publication
Jordan
No. of Pages
13
Main Subjects
Information Technology and Computer Science
Abstract EN
Network intrusion is a subject of great concern to a variety of stakeholders.
Decision fusion (ensemble) models that combine several base learners have been widely used to enhance detection rate of unauthorised network intrusion.
However, the design of such an optimal decision fusion classifier is a challenging and open problem.
The Matthews Correlation Coefficient (MCC) is an effective measure for detecting associations between variables in many fields; however, very few studies have applied it in selecting weak learners to the best of the authors’ knowledge.
In this paper, we propose a decision fusion model with correlation-based MCC weak learner selection technique to augment the classification performance of the decision fusion model under a StackNet strategy.
Specifically, the proposed model sought to improve the association between the prediction accuracy and diversity of base classifiers.
We compare our proposed model with five other ensemble models, a deep neural model and two stand-alone state-of-the-art classifiers commonly used in network intrusion detection based on accuracy, the Area Under Curve (AUC), recall, precision, F1-score and Kappa evaluation metrics.
The experimental results using benchmark dataset KDDcup99 from Kaggle shows that the proposed model has a identified unauthorised network traffic at 99.8% accuracy, Extreme Gradient Boosting (Xgboost) (97.61%), Catboost (97.49%), Light Gradient Boosting Machine (LightGBM) (98.3%), Multilayer Perceptron (MLP) (97.7%), Random Forest (RF) (97.97%), Extra Trees Classifier (ET) (95.82%), Different decision (DT) (96.95%) and, K-Nearest Neighbor (KNN) (95.56), indicating that it is a more efficient and better intrusion detection system.
American Psychological Association (APA)
Nti, Isaac Kofi& Narko Boateng, Owusu& Adekoya, Adebayo Felix& Somanathan, Arjun Remadevi. 2022. Stacknet based decision fusion classifier for network intrusion detection. The International Arab Journal of Information Technology،Vol. 19, no. 3A (s), pp.478-490.
https://search.emarefa.net/detail/BIM-1437122
Modern Language Association (MLA)
Nti, Isaac Kofi…[et al.]. Stacknet based decision fusion classifier for network intrusion detection. The International Arab Journal of Information Technology Vol. 19, no. 3A (Special issue) (2022), pp.478-490.
https://search.emarefa.net/detail/BIM-1437122
American Medical Association (AMA)
Nti, Isaac Kofi& Narko Boateng, Owusu& Adekoya, Adebayo Felix& Somanathan, Arjun Remadevi. Stacknet based decision fusion classifier for network intrusion detection. The International Arab Journal of Information Technology. 2022. Vol. 19, no. 3A (s), pp.478-490.
https://search.emarefa.net/detail/BIM-1437122
Data Type
Journal Articles
Language
English
Notes
Includes bibliographical references : p. 487-489
Record ID
BIM-1437122