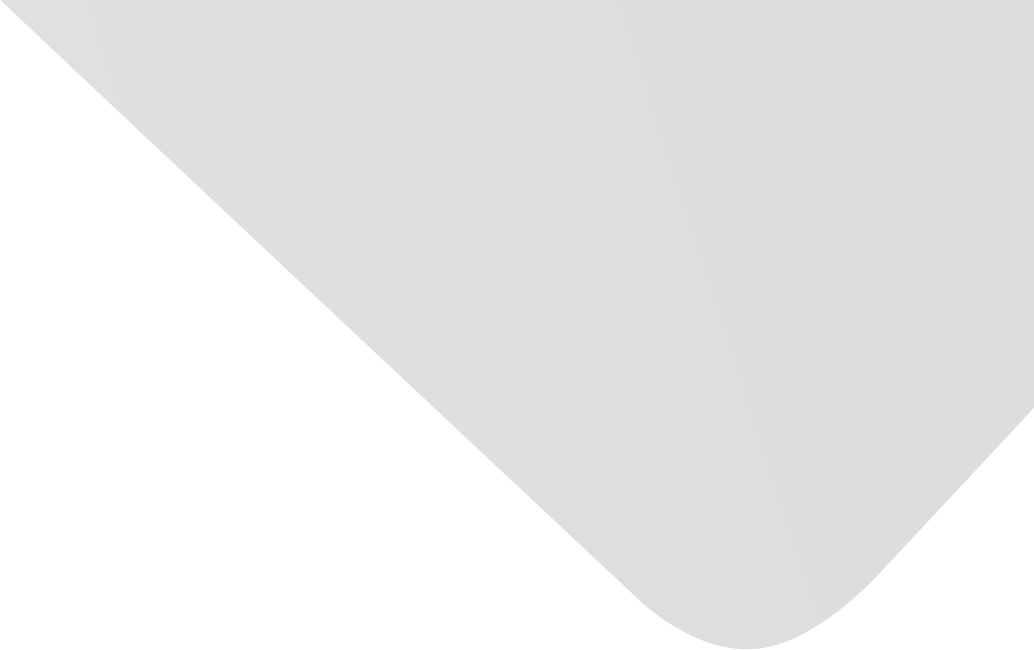
A fire detection algorithm using convolutional neural network
Other Title(s)
خوارزمية جديدة للكشف عن الحرائق باستخدام نهج التعلم العميق
Author
Source
Journal of King Abdulaziz University : Engineering Sciences
Issue
Vol. 32, Issue 2 (31 Dec. 2022), pp.39-55, 17 p.
Publisher
King Abdulaziz University Scientific Publishing Center
Publication Date
2022-12-31
Country of Publication
Saudi Arabia
No. of Pages
17
Main Subjects
Abstract AR
النار تدمر كل شيء في طريقها.
إنه أكبر خطر يسبب الكوارث.
يمكن أن تبدأ من اشتعال صغير و يمكن أن يؤدي إلى خسارة كبيرة أو كارثة غير مرغوب فيها.
يفقد الناس حياتهم من الحرائق.
وفقا للرابطة الوطنية للحماية من الحرائق (NFPA)، بلغت حالات الحرائق المبلغ عنها حوالي 1400000 في عام 2020 بينما تسببت هذه الحالات في ما يقرب من 3500 حالة وفاة مدنية.
بالإضافة إلى ذلك، فإن عدد المدنيين المصابين من الحرائق حوالي 15000 و تبلغ الكلفة التقديرية للخسائر في العقارات حوالي 21 مليار دولار أمريكي.
و بالتالي، أصبح الكشف عن الحرائق موضوعا مهما للغاية خاصة بسبب التكنولوجيا السريعة و المتوسطة.
في هذه الورقة، يتم اقتراح طريقة بسيطة و سريعة و دقيقة للكشف عن الحرائق باستخدام التعلم العميق.
تم تطوير هذه الطريقة بناء على تقنيات معالجة الصور و الشبكة العصبية.
إذا لم يتم الكشف عن الحريق مبكرا، فإن مستوى الأكسجين ينخفض و قد يؤدي ذلك إلى الاختناق.
لذلك، يمكن أن تساعدنا هذه الطريقة من خلال اكتشاف الحرائق في مرحلة مبكرة.
يقوم هذا النهج بتقسيم الصور و ترشيحها على شكل بكسل بناء على عدة شروط و محددات و مميزات و خصائص لهذه الصور مثل الألوان وشدة سطوعها و وضوحها و الملمس باللهب مع انعكاسها.
يستخدم MATLAB كأداة محاكاة لإجراء العديد من التجارب للتحقق من فعالية الطريقة المقترحة.
تظهر النتائج التي تم الحصول عليها أن الدقة كانت أكثر من 97% عند تطبيقها على أكثر من 700 صورة لأغراض التدريب و الاختبار.
أخيرا تم توفير نتائج دراسة مقارنة مرجعية بين النهج المقترح و بعض الدراسات المقدمة سابقا للكشف عن الحرائق.
Abstract EN
Fire destroys everything on its way.
It is the most dangerous hazard that causes disasters.
It can be started from a small ignition which could lead to a big loss or unwanted disaster.
People lose their lives from fires.
According to National Fire Protection Association (NFPA), the reported fire cases were nearly 1,400,000 in 2020 while those cases caused almost 3,500 civilian deaths.
In addition, the number of civilians injured from fires is around 15,000 and the estimated properties damage is around 21 billion in US Dollars.
Thus, detection of fire has become a very important topic especially due to the rapid and evolving of technology.
In this paper, a simple, fast and accurate method of fire detection using deep learning is proposed.
This method is developed based on image processing techniques.
If the fire is not detected early, then the Oxygen level decreases which leads to suffocation.
So, this method can help us by detecting fires at an early stage.
This approach filters an image into pixels based on thresholds according to some features such as colors, immobility source and flame texture with its reflection.
MATLAB is used as a simulation tool to conduct several experiments to verify the effectiveness of the proposed method.
The obtained results show that the accuracy was over 97% when applied on more than 700 images for training and testing purposes.
American Psychological Association (APA)
al-Shaykhi, Ahmad A.. 2022. A fire detection algorithm using convolutional neural network. Journal of King Abdulaziz University : Engineering Sciences،Vol. 32, no. 2, pp.39-55.
https://search.emarefa.net/detail/BIM-1469509
Modern Language Association (MLA)
al-Shaykhi, Ahmad A.. A fire detection algorithm using convolutional neural network. Journal of King Abdulaziz University : Engineering Sciences Vol. 32, no. 2 (2022), pp.39-55.
https://search.emarefa.net/detail/BIM-1469509
American Medical Association (AMA)
al-Shaykhi, Ahmad A.. A fire detection algorithm using convolutional neural network. Journal of King Abdulaziz University : Engineering Sciences. 2022. Vol. 32, no. 2, pp.39-55.
https://search.emarefa.net/detail/BIM-1469509
Data Type
Journal Articles
Language
English
Notes
Includes bibliographical references : p. 53-54
Record ID
BIM-1469509