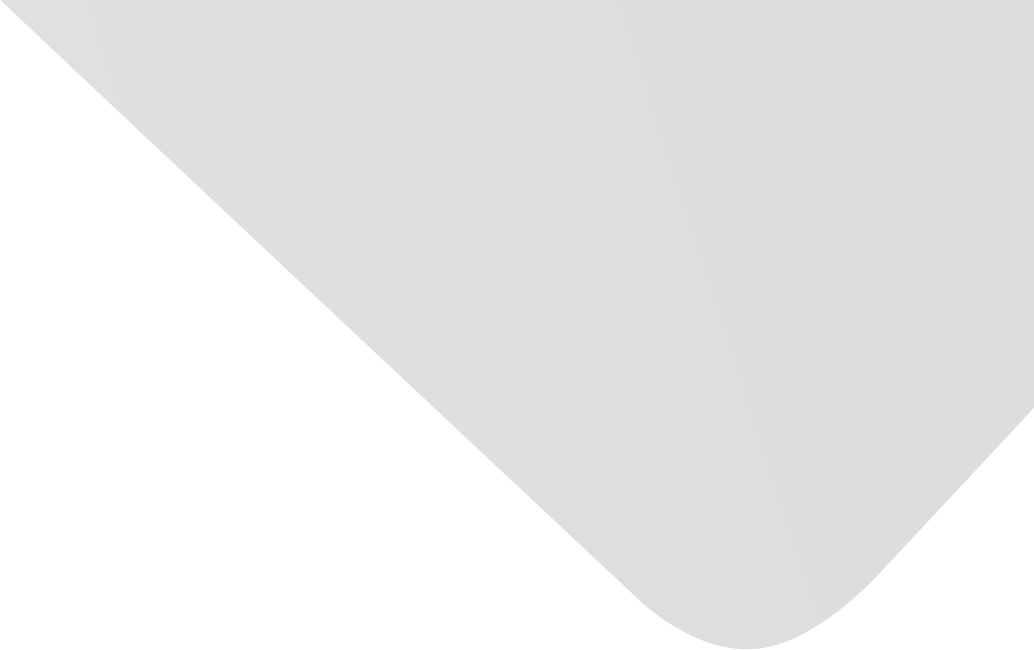
Hybridization of swarm for features selection to modeling heart attack data
Other Title(s)
تهجين الأسراب لاختيار الميزات لنمذجة بيانات النوبات القلبية
Joint Authors
Hasan, Umar Shakir
Salih, Ibrahim Ahmad
Source
al- Rafidain Journal of Computer Sciences and Mathematics
Issue
Vol. 16, Issue 2 (31 Dec. 2022), pp.25-34, 10 p.
Publisher
University of Mosul College of Computer Science and Mathematics
Publication Date
2022-12-31
Country of Publication
Iraq
No. of Pages
10
Main Subjects
Information Technology and Computer Science
Abstract AR
يعد توقع النوبات القلبية باستخدام التعلم الآلي موضوعا مهما.
تحتوي مجموعات البيانات الطبية سمات مختلفة منها ماهو مرتبط بالفئة المستهدفة للتنبؤ و منها ماهو غير مرتبط بالاضافة الى ان مجموعات البيانات تكون غير متوازنة بشكل مفرط ممايؤدي الى انحياز نماذج تعلم الآلة عند نمذجة النوبات القلبية.
و لنمذجة مجموعة بيانات النوبات القلبية الغير متوازنة تقترح هذه الورقة تهجين الاسراب (PSO, BAT, BCS) لتحديد الميزات و اعتماد الprecision لصنف الاقلية كدالة لياقة لكل سرب لتحديد الميزات ذات التأثير.
و لنمذجة مجموعة البيانات التي تم تحديد السمات فيها تم اقتراح استخدام مبدا ال ( boosting (Catboost كمصنف للتنبؤ بالنوبات القلبية.
تمت مقارنة الطريقة المختارة لتحديد الميزات مع كل من الاسراب الثلاثة ومقارنة خوارزمية Catboost مع خوارزميات التصنيف التقليدية ( naive Bayes, decision trees).
توصلت الدراسة الى ان الطريقة المقترحة في دمج نتائج خورازميات الاسراب المختارة في تحديد الميزات يعد حلا و اعدا في مجال تحديد الميزات ويزيد من دقة النظام وان نماذج تعلم الآلة التقليدية تنحاز في حالة مجمو عات البيانات الغير متوازنة و ان تحديد السمات ذات الاهمية وفقا للفئة المستهدفة ذات تأثير على اداء النماذج بالاضافة الى ان تحديد المعلمات الفائقة يقلل من انحياز النموذج المختار.
حقق النموذج النهائي دقة عامة 97% بمقياس (Accuracy و 80% بمقياس (precision لفئة الاقلية.
Abstract EN
Predicting heart attacks using machine learning is an important topic.
Medical data sets contain different features, some of which are related to the target group for prediction and some are not.
In addition, the data sets are excessively unbalanced, which leads to the bias of machine learning models when modeling heart attacks.
To model the unbalanced heart attack data set, this paper proposes the hybridization of Particle swarm optimization (PSO), BAT, and Cuckoo Search (CS) to select the features and adopt the precision for minority classes as a fitness function for each swarm to select the influential features.
In order to model the data, set in which the features were selected, it was proposed to use the boosting (Catboost) as a classifier for predicting heart attacks.
The proposed method to select features has been compared with each of the three swarms, and the Catboost algorithm has been compared to traditional classification algorithms (naive Bayes, decision trees).
The study found that the proposed method of hybridization of the results of the (PSO, BAT, and BCS) algorithms in selecting features is a promising solution in the field of selecting features and increases the accuracy of the system, and that traditional machine learning models are biased in the case of unbalanced data sets and that selecting the important features according to the target class has an impact on the performance of the models, In addition, the definition of hyperparameters reduces the bias of the selected model.
The final model achieved an overall accuracy of 96% on the Accuracy scale and 56% the Precision scale for minority class on the
American Psychological Association (APA)
Hasan, Umar Shakir& Salih, Ibrahim Ahmad. 2022. Hybridization of swarm for features selection to modeling heart attack data. al- Rafidain Journal of Computer Sciences and Mathematics،Vol. 16, no. 2, pp.25-34.
https://search.emarefa.net/detail/BIM-1493483
Modern Language Association (MLA)
Hasan, Umar Shakir& Salih, Ibrahim Ahmad. Hybridization of swarm for features selection to modeling heart attack data. al- Rafidain Journal of Computer Sciences and Mathematics Vol. 16, no. 2 (2022), pp.25-34.
https://search.emarefa.net/detail/BIM-1493483
American Medical Association (AMA)
Hasan, Umar Shakir& Salih, Ibrahim Ahmad. Hybridization of swarm for features selection to modeling heart attack data. al- Rafidain Journal of Computer Sciences and Mathematics. 2022. Vol. 16, no. 2, pp.25-34.
https://search.emarefa.net/detail/BIM-1493483
Data Type
Journal Articles
Language
English
Notes
Includes bibliographical references : p. 33-34
Record ID
BIM-1493483